Machine Learning Classification of Dust Impact Signals Observed by The Solar Orbiter Radio and Plasma Waves Instrument
crossref(2022)
摘要
<p>We present results from automatic classification of dust waveforms observed by The Solar Orbiter Radio and Plasma Waves Instrument.</p><p>Every day, several dust particles impacts the Solar Orbiter as the probe travels trough the inner heliosphere. The dust impact produces a cloud of electrons and ions on the spacecraft surface and the free charge causes a sharp and characteristic voltage signal, which decays towards the equilibrium potential after a few milliseconds via interaction with the ambient plasma. Detection and analysis of the characteristic dust waveform can be used to map the density, size and velocity distribution of dust particles in the inner heliosphere, and thus enhance our understanding of the role of dust in the solar system. Such statistical analysis do however require reliable dust detection software.</p><p>It is challenging to automatically detect and separate dust waveforms from other signal shapes by "hard coded" algorithms. Both due to spacecraft charging, causing variable shapes of impact signals, and since electromagnetic waves (such as solitary waves) may induce resembling voltage signals. Here we present results of waveform classification using various supervised machine learning techniques, where manually classified data is used both to train and test the classifiers.</p><p>We investigate automatic machine learning classification as a possible tool to make statistical analysis of the distribution of dust in the inner heliosphere more reliable and easier to conduct. Furthermore, the classifier may possibly be used on data (after pre-processing) from other spacecrafts with similar instruments, such as the Parker Solar Probe (PSP), the Solar Terrestrial Relations Observatory (STEREO) and the Magnetospheric Multiscale (MMS) mission.</p>
更多查看译文
AI 理解论文
溯源树
样例
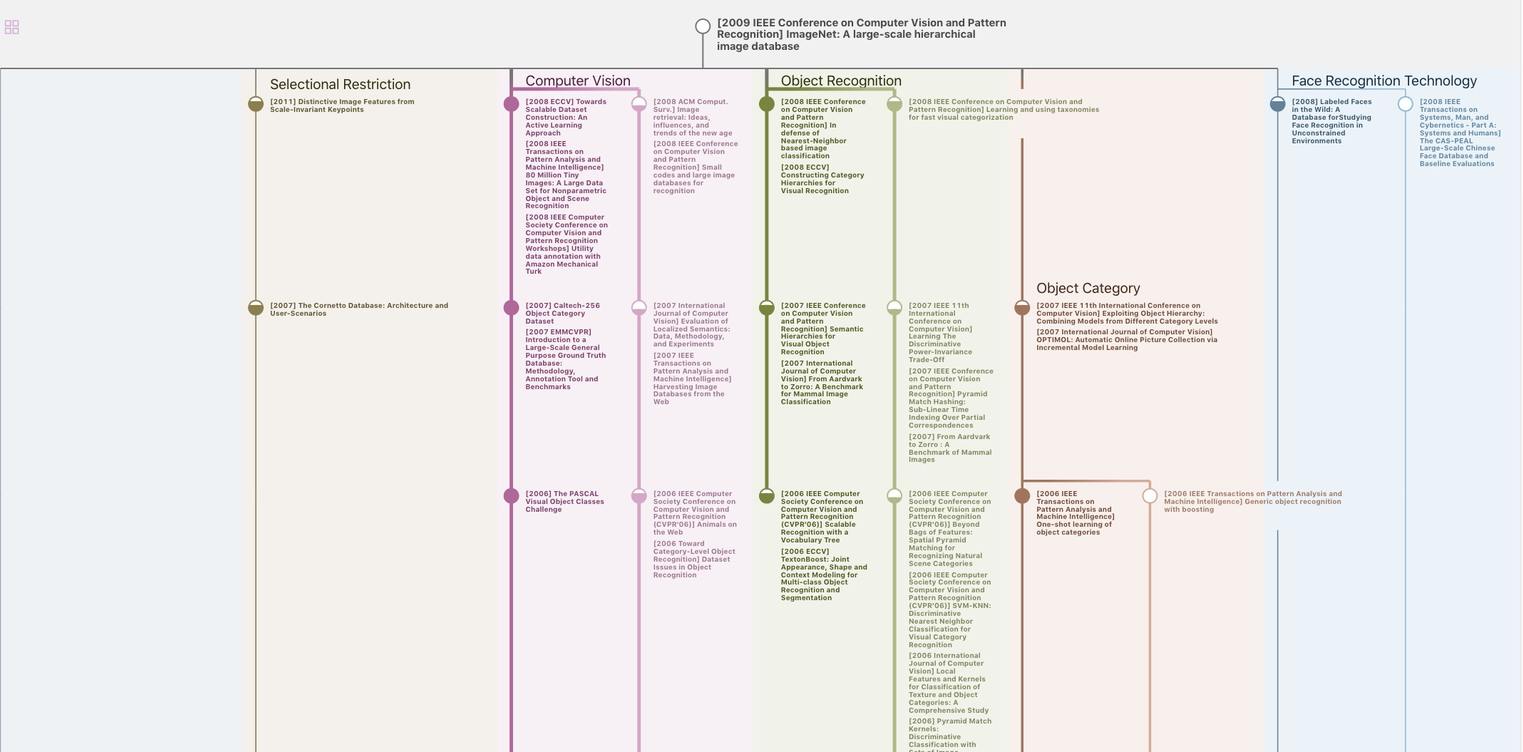
生成溯源树,研究论文发展脉络
Chat Paper
正在生成论文摘要