A Tensor Based Framework For Community Detection In Dynamic Networks
2017 IEEE INTERNATIONAL CONFERENCE ON ACOUSTICS, SPEECH AND SIGNAL PROCESSING (ICASSP)(2017)
摘要
Many systems from human brain to the networks on social media, can be modeled as graphs. The network structure helps us understand, predict and optimize the behavior of dynamical systems. One of the important tools in understanding network topology is community detection. Even though community detection methods are well developed for static networks, the extensions to the dynamic case are more limited. In this paper, we introduce two tensor based frameworks, windowed and running time, for identifying and tracking the network community structure across time. The frameworks take the history of the networks into account. The proposed approach relies on determining the subspace across time using the Tucker decomposition of a tensor constructed from networks across time. We also propose a computationally efficient way to update the subspace estimates across time to track changes in community structure. The proposed approach is evaluated on both simulated and real dynamic networks.
更多查看译文
关键词
Tensor decomposition, Dynamic networks, Spectral Clustering
AI 理解论文
溯源树
样例
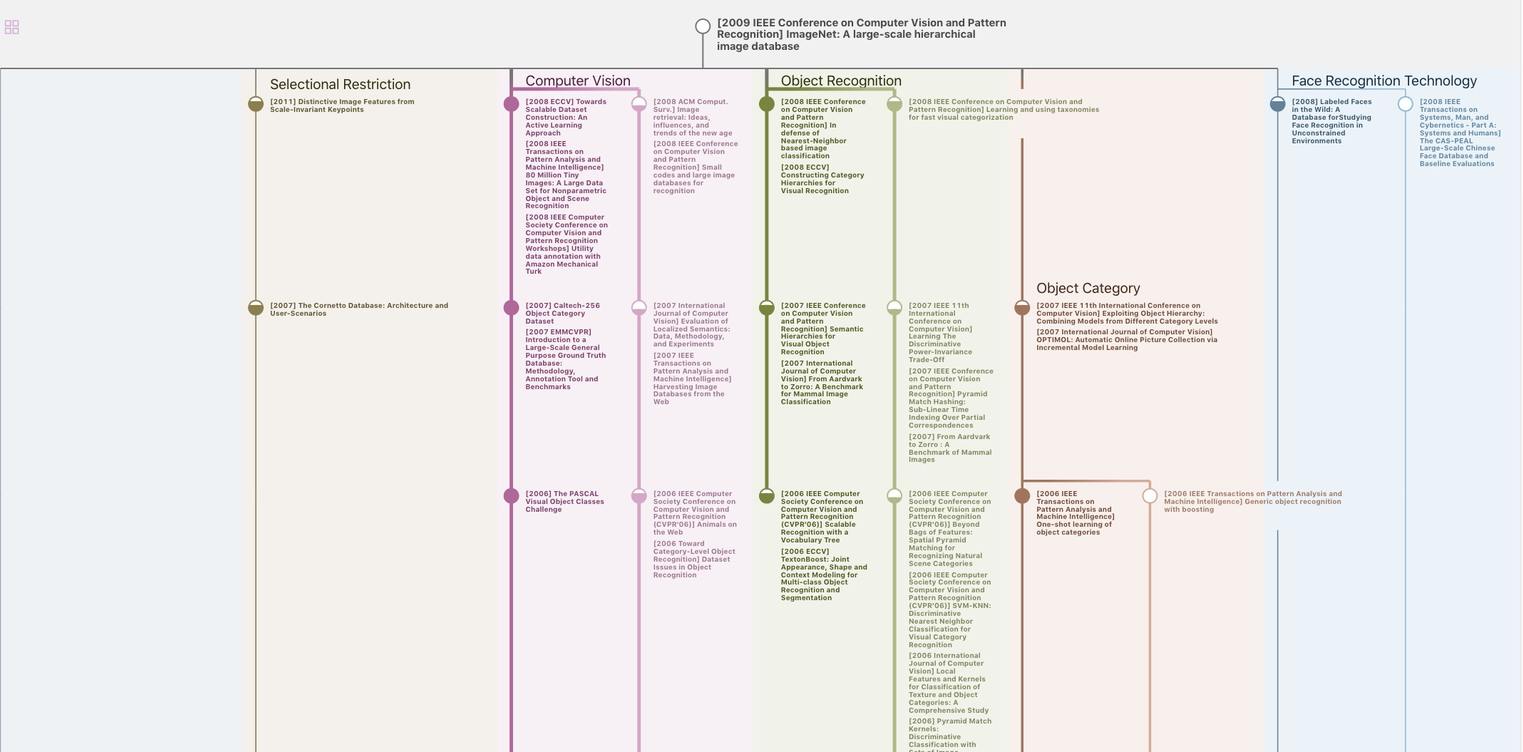
生成溯源树,研究论文发展脉络
Chat Paper
正在生成论文摘要