Towards real-time probabilistic ash deposition forecasting for Aotearoa New Zealand
Research Square (Research Square)(2022)
摘要
Abstract Volcanic ashfall forecasts are highly dependent on eruption parameters and synoptic weather conditions at the time and location of the eruption. In Aotearoa, New Zealand, MetService and GNS Science have been jointly developing an ashfall forecast system that incorporates 4D high-resolution numerical weather prediction (NWP) and eruption parameters into the HYSPLIT model, a state-of-the art hybrid Eulerian and Lagrangian dispersion model widely used for volcanic ash. However, these forecasts are based on discrete eruption parameters combined with a deterministic weather forecast and thus provide no information on output uncertainty. This shortcoming hinders stakeholder decision making, particularly near the geographical margin of forecasted ashfall and in areas with large gradients in forecasted ash deposition. This study presents a new approach that incorporates uncertainty from both eruptive and meteorologic inputs to deliver uncertainty in the model output. To this end, we developed probabilistic density functions (PDFs) for the three key eruption parameters (plume height, mass eruption rate, eruption duration) tailored to Aotearoa’s volcanoes, and combine them with NWP ensemble datasets to generate probabilistic ashfall forecasts using the HYSPLIT model. We show that the Latin Hypercube Sampling (LHS) technique can be used to representatively span this high-dimensional parameter space with fewer model runs than Monte Carlo techniques, thus allowing this methodology to be used in near real-time forecast systems. We also propose new probabilistic summary products such as hazard matrices, probability of exceedance of cumulative ashfall, and arrival time forecasting, which together support public information and emergency responders decision making.
更多查看译文
关键词
probabilistic ash deposition forecasting,aotearoa new zealand,new zealand,real-time
AI 理解论文
溯源树
样例
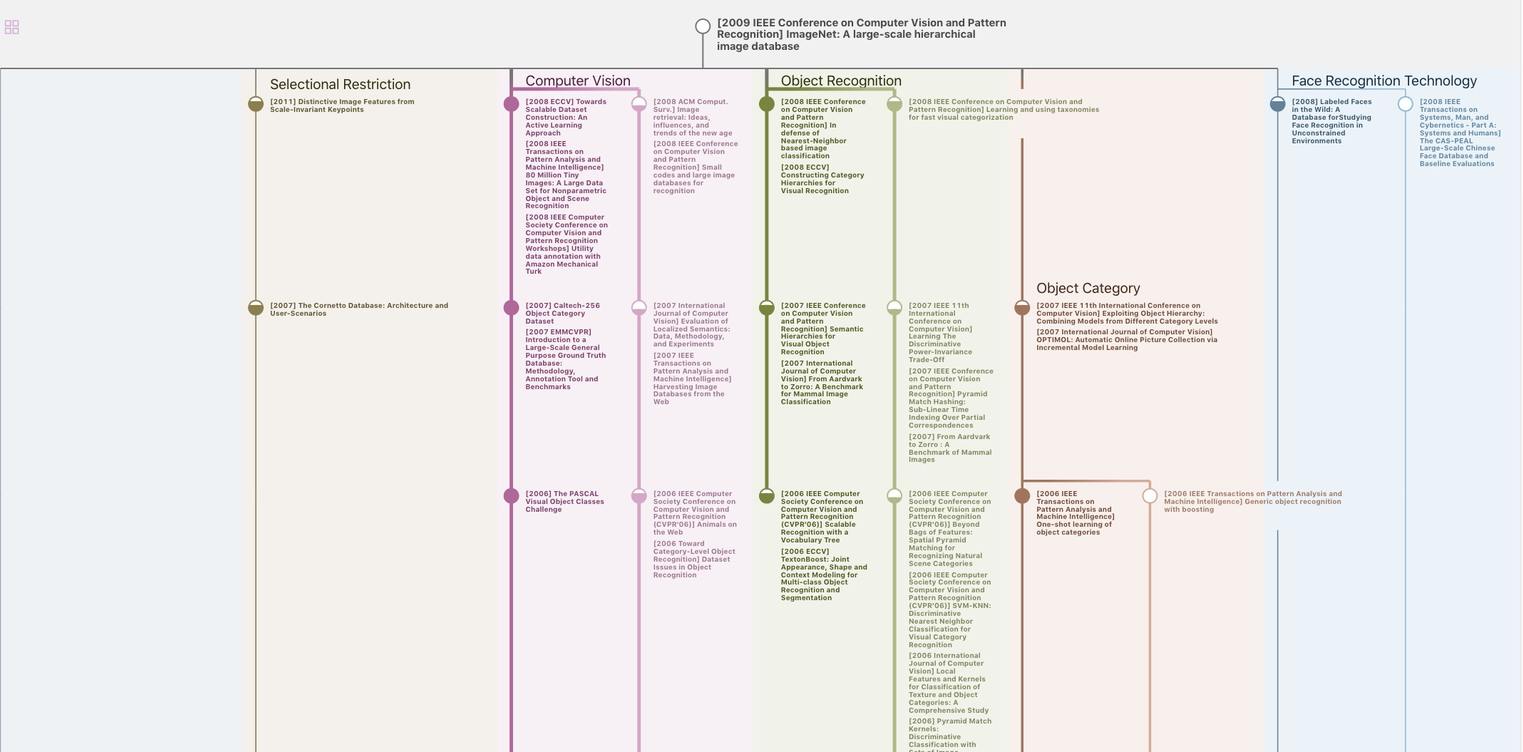
生成溯源树,研究论文发展脉络
Chat Paper
正在生成论文摘要