Time series analysis of synthetic time series generated with a saturated/unsaturated zone model
crossref(2022)
Abstract
<p>Time series analysis with response functions is a versatile approach to analyze measured head series in observation wells. In such an analysis, the response function of the groundwater does not change with time. This approach works well when the groundwater recharge is a linear function of the measured rainfall and evaporation, as was shown through the analysis of synthetic time series generated with a saturated groundwater model where the groundwater recharge is applied directly to the saturated zone. In this research, the method was evaluated for situations where the groundwater recharge cannot be approximated well as a linear function of the measured rainfall and evaporation. Synthetic time series were generated with a two-dimensional saturated/unsaturated zone model (Hydrus2D) and analyzed with response functions. Performance of the time series model was improved through inclusion of a new root zone model consisting of a single reservoir. Reservoir inflow is measured rainfall. Reservoir outflow is evaporation and groundwater recharge, where the evaporation is a function of the amount of water stored in the root zone and the recharge is a function of both the amount of water stored in the root zone and the groundwater table. The new root zone model is a promising tool for the analysis of head series in areas with thick unsaturated zones and/or high potential evaporative fluxes.</p>
MoreTranslated text
求助PDF
上传PDF
View via Publisher
AI Read Science
AI Summary
AI Summary is the key point extracted automatically understanding the full text of the paper, including the background, methods, results, conclusions, icons and other key content, so that you can get the outline of the paper at a glance.
Example
Background
Key content
Introduction
Methods
Results
Related work
Fund
Key content
- Pretraining has recently greatly promoted the development of natural language processing (NLP)
- We show that M6 outperforms the baselines in multimodal downstream tasks, and the large M6 with 10 parameters can reach a better performance
- We propose a method called M6 that is able to process information of multiple modalities and perform both single-modal and cross-modal understanding and generation
- The model is scaled to large model with 10 billion parameters with sophisticated deployment, and the 10 -parameter M6-large is the largest pretrained model in Chinese
- Experimental results show that our proposed M6 outperforms the baseline in a number of downstream tasks concerning both single modality and multiple modalities We will continue the pretraining of extremely large models by increasing data to explore the limit of its performance
Upload PDF to Generate Summary
Must-Reading Tree
Example
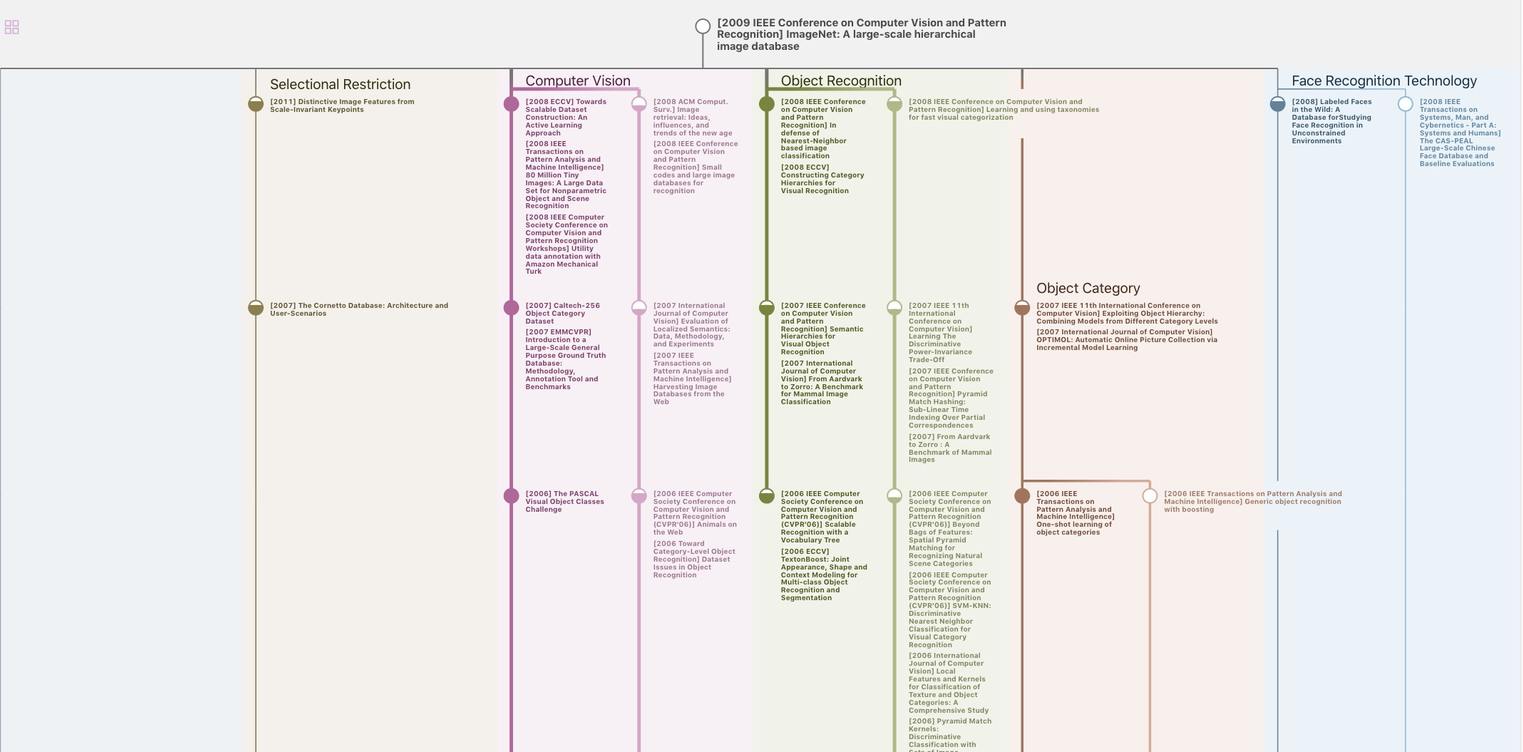
Generate MRT to find the research sequence of this paper
Data Disclaimer
The page data are from open Internet sources, cooperative publishers and automatic analysis results through AI technology. We do not make any commitments and guarantees for the validity, accuracy, correctness, reliability, completeness and timeliness of the page data. If you have any questions, please contact us by email: report@aminer.cn
Chat Paper
GPU is busy, summary generation fails
Rerequest