Exploring the relationship between S2S temperature forecasting errors and Earth system variables
crossref(2022)
摘要
<p>Accurate weather forecasts can help to reduce costs and impacts related to weather and corresponding extremes. The quality of weather forecasts has improved considerably in recent decades as models are representing more physical processes, and can increasingly benefit from assimilating comprehensive Earth observation data. However, this increased complexity presents a challenge for pinpointing weaknesses in the forecast models’ process representations, which is needed to support continuous improvement in forecast accuracy.</p><p>In this study, we use a comprehensive set of observation-based ecological, hydrological and meteorological variables to study their potential for explaining temperature forecast errors at the weekly time scale. For this purpose, we computed Spearman correlations between each considered variable and the forecast error obtained from the ECMWF S2S re-forecasts dataset with lead times between 1 and 6 weeks. This is done across the globe for the time period 2001-2017. The results suggest that circulation-related variables such as wind speed and spatial pressure differences are overall most strongly related to forecast errors across the globe, suggesting that an improved representation of the large-scale circulation in the forecast model has the greatest potential to improve temperature forecasts. At the same time we found particular regions and seasons in which other variables are more strongly related to forecast errors, for  instance: i) during the growing season in Central Europe, Central Africa and Northern South America, the vegetation greenness and soil moisture are relevant, and ii) meteorological variables such as solar radiation, precipitation and sea surface temperature are relevant in Asia and Eastern Europe during boreal summer and autumn. Additionally, we found that the actual values of the variables are generally more strongly related to the forecast errors than their anomalies, pointing towards a systematic nature of the errors. Towards longer lead times, in contrast, the relevance of anomalies increases while correlations with the absolute values decrease. This highlights that biophysical information beyond the mean seasonal cycle can be informative for temperature forecasts. </p><p>Our identification of variables related to forecast errors can inform the development of forecast models and data assimilation schemes, considering that most of the highlighted variables have corresponding satellite datasets available in near-real-time on a global scale.</p>
更多查看译文
AI 理解论文
溯源树
样例
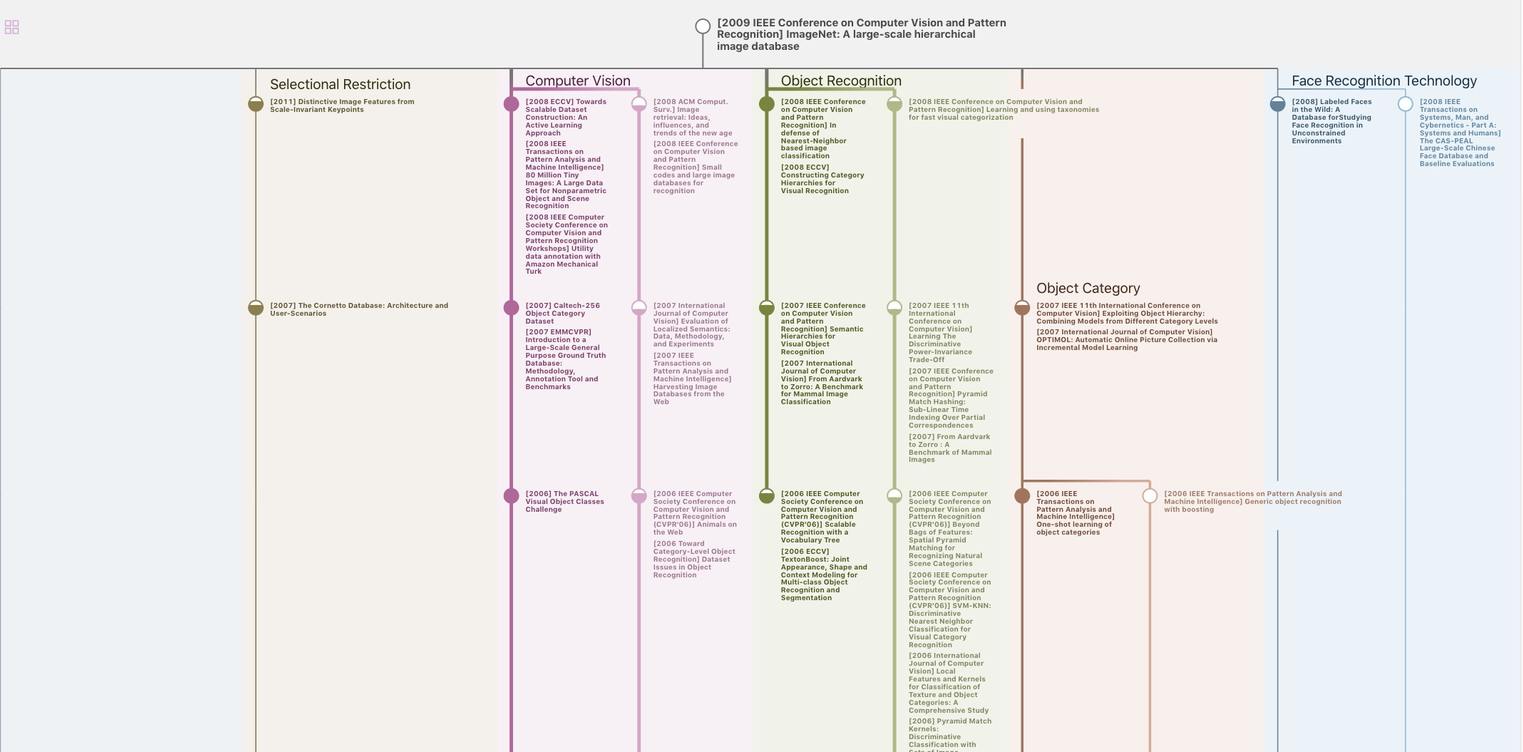
生成溯源树,研究论文发展脉络
Chat Paper
正在生成论文摘要