Choose your own weather adventure: deep weather generation for “what-if” climate scenarios
crossref(2022)
摘要
<p>Climate change is making extreme weather more extreme. Given the inherent uncertainty of long-term climate projections, there is growing need for rapid, plausible “what-if” climate scenarios to help users understand climate exposure and examine resilience and mitigation strategies. Since the 1980s, such “what-if” scenarios have been created using stochastic weather generators. However, it is very challenging for traditional weather generation algorithms to create realistic extreme climate scenarios because the weather data being modeled is highly imbalanced, contains spatiotemporal dependencies and has extreme weather events exacerbated by a changing climate.</p><p>There are few works comparing and evaluating stochastic multisite (i.e., gridded) weather generators, and no existing work that compares promising deep learning approaches for weather generation with classical stochastic weather generators. We will present the culmination of a multi-year effort to perform a systematic evaluation of stochastic weather generators and deep generative models for multisite precipitation synthesis. Among other things, we show that variational auto-encoders (VAE) offer an encouraging pathway for efficient and controllable climate scenario synthesis – especially for extreme events. Our proposed VAE schema selects events with different characteristics in the normalized latent space (from rare to common) and generates high-quality scenarios using the trained decoder. Improvements are provided via latent space clustering and bringing histogram-awareness to the VAE loss.</p><p>This research will serve as a guide for improving the design of deep learning architectures and algorithms for application in Earth science, including feature representation and uncertainty quantification of Earth system data and the characterization of so-called “grey swan” events.</p>
更多查看译文
AI 理解论文
溯源树
样例
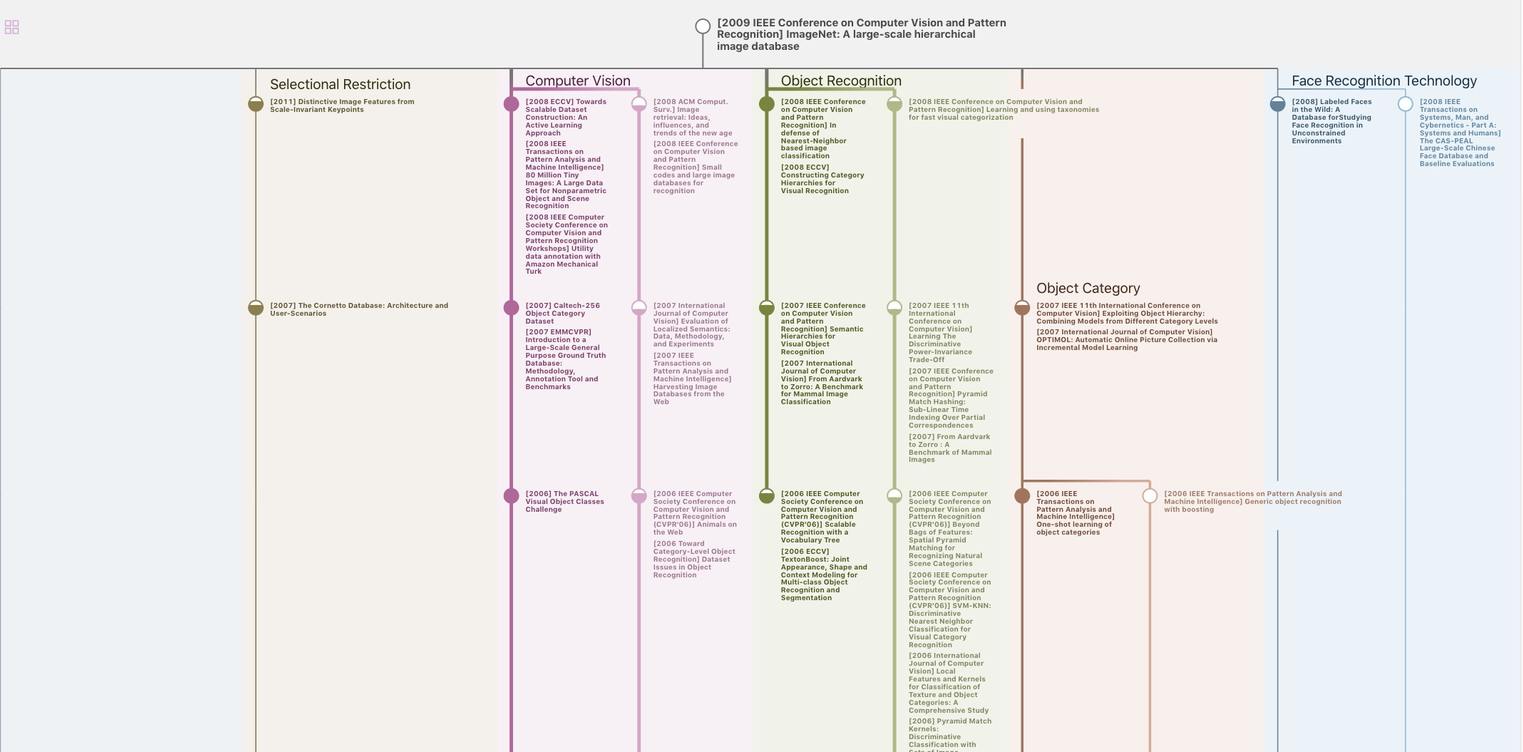
生成溯源树,研究论文发展脉络
Chat Paper
正在生成论文摘要