Application of unsupervised machine learning techniques for lithological and soil mapping in Ossa-Morena Zone
crossref(2022)
摘要
<p>Unsupervised methods are a good entry point for satellite image classification, requiring little to no input, and outputting an analysis, in the form of a thematic map, that may act as a guide for more user input intensive methods. For this work, we use K-means methods to classify satellite and drone imagery that cover the Ossa-Morena Zone (OMZ), in Portugal, and assess their capacity for lithological and soil mapping. The drone is equipped with a High Precision NDVI Single Sensor and was flown over the ancient mines of Mociços, Mostardeira and Santa Eulália. The OMZ is a tectonostratigraphic domain shared between Portugal and Spain, divided in Sectors, extraordinarily rich and diverse from a lithological, stratigraphical, and structural point-of-view; for this work, we will focus on the Estremoz-Barrancos sector, comprised of a Neoproterozoic to Devonian metasedimentary succession, with a low-grade metamorphism in greenschist facies, and the Santa Eulália Plutonic Complex (SEPC), an elliptic late-Variscan granitic massif that crosscuts the Alter do Chão-Elvas Sector and the Blastomylonitic belt, constituted by two granitic facies, a few small mafic bodies, and some roof pendants that belong to the Alter do Chão-Elvas Sector.</p><p>The imagery used correspond to high-level satellite imagery products gathered between 2004 to 2006 (ASTER) and 2017 to 2021 (Landsat 8 and Sentinel-2), and drone imagery captured on May 6th and August 31st, 2021.</p><p>The K-means was applied to a variable number of selected bands, including band ratios, and tested for different number of initial clusters and different distance algorithms (Minimum Distance and Spectral Angle Mapping). Afterwards, it was assessed its ability to outlining and classify different geological structures by comparing the results to the geological map of OMZ.</p><p>The obtained thematic maps points towards poorer results when using a larger selection of bands - for instance, ASTER bands 1 to 9 (in which bands 1 to 3N were resampled to 30m) -, due to interspersion of different classes, whereas when using band ratio combinations, such as 4/2 and 6/(5+7) (ASTER), the produced map successfully classifies the major geological features present in the region, with increased sharpness between contacts with a higher number of classes.</p><p>Results show that K-means, when used under the correct conditions and parameters, has the potential for lithological and soil mapping through image classification, both for satellite and drone imagery.</p><p>Future work will focus on the integration of a pre-processing step for band selection using ML techniques, such as through Principal Component Analysis, Minimum Noise Fraction and Random Forest.</p>
更多查看译文
AI 理解论文
溯源树
样例
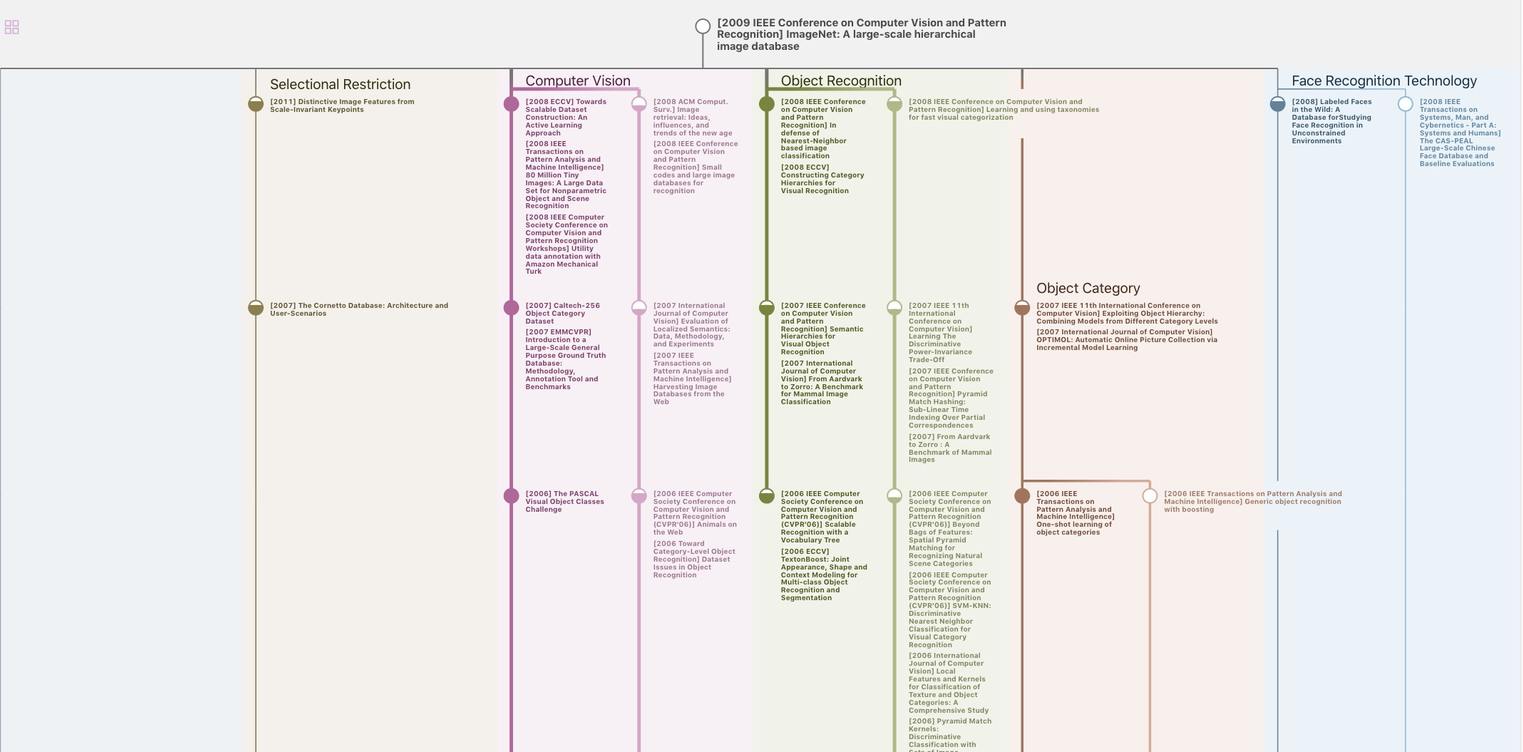
生成溯源树,研究论文发展脉络
Chat Paper
正在生成论文摘要