Efficient Parameter Estimation of Sampled Random Fields Using the Debiased Spatial Whittle Likelihood
crossref(2022)
摘要
<p>We establish a theoretical framework, an algorithmic basis, and a computational workflow for the statistical analysis of multi-variate multi-dimensional random fields - sampled (possibly irregularly, with missing data) and finite (possibly bounded irregularly). Our research is practically motivated by geodetic and scientific problems of topography and gravity analysis in geophysics and planetary physics, but our solutions fulfill the more general need for sophisticated methods of inference that can be applied to massive remote-sensing data sets, and as such, our mathematical, statistical, and computational solutions transcend any particular application. The generic problem that we are addressing is: two (or more) spatial fields are observed, e.g., by passive or active sensing, and we desire a parsimonious statistical description of them, individually and in their relation to one another. We consider the fields to be realizations of a random process, parameterized as a Matern covariance structure, a very flexible description that includes, as special cases, many of the known models in popular use (e.g. exponential, autoregressive, von Karman, Gaussian, Whittle, ...) Our fundamental question is how to find estimates of the parameters of a Matern process, and the distribution of those estimates for uncertainty quantification. Our answer is, fundamentally: via maximum-likelihood estimation.  We now provide a computationally and statistically efficient method for estimating the parameters of a stochastic covariance model observed on a regular spatial grid in any number of dimensions. Our proposed method, which we call the Debiased Spatial Whittle likelihood, makes important corrections to the well-known Whittle likelihood to account for large sources of bias caused by boundary effects and aliasing. We generalise the approach to flexibly allow for significant volumes of missing data including those with lower-dimensional substructure, and for irregular sampling boundaries. We build a theoretical framework under relatively weak assumptions which ensures consistency and asymptotic normality in numerous practical settings including missing data and non-Gaussian processes. We also extend our consistency results to multivariate processes. We provide detailed implementation guidelines which ensure the estimation procedure can still be conducted in O(n log n) operations, where n is the number of points of the encapsulating rectangular grid, thus keeping the computational scalability of Fourier and Whittle-based methods for large data sets. We validate our procedure over a range of simulated and real world settings, and compare with state-of-the-art alternatives, demonstrating the enduring practical appeal of Fourier-based methods, provided they are corrected and augmented by the procedures that we developed.</p>
更多查看译文
AI 理解论文
溯源树
样例
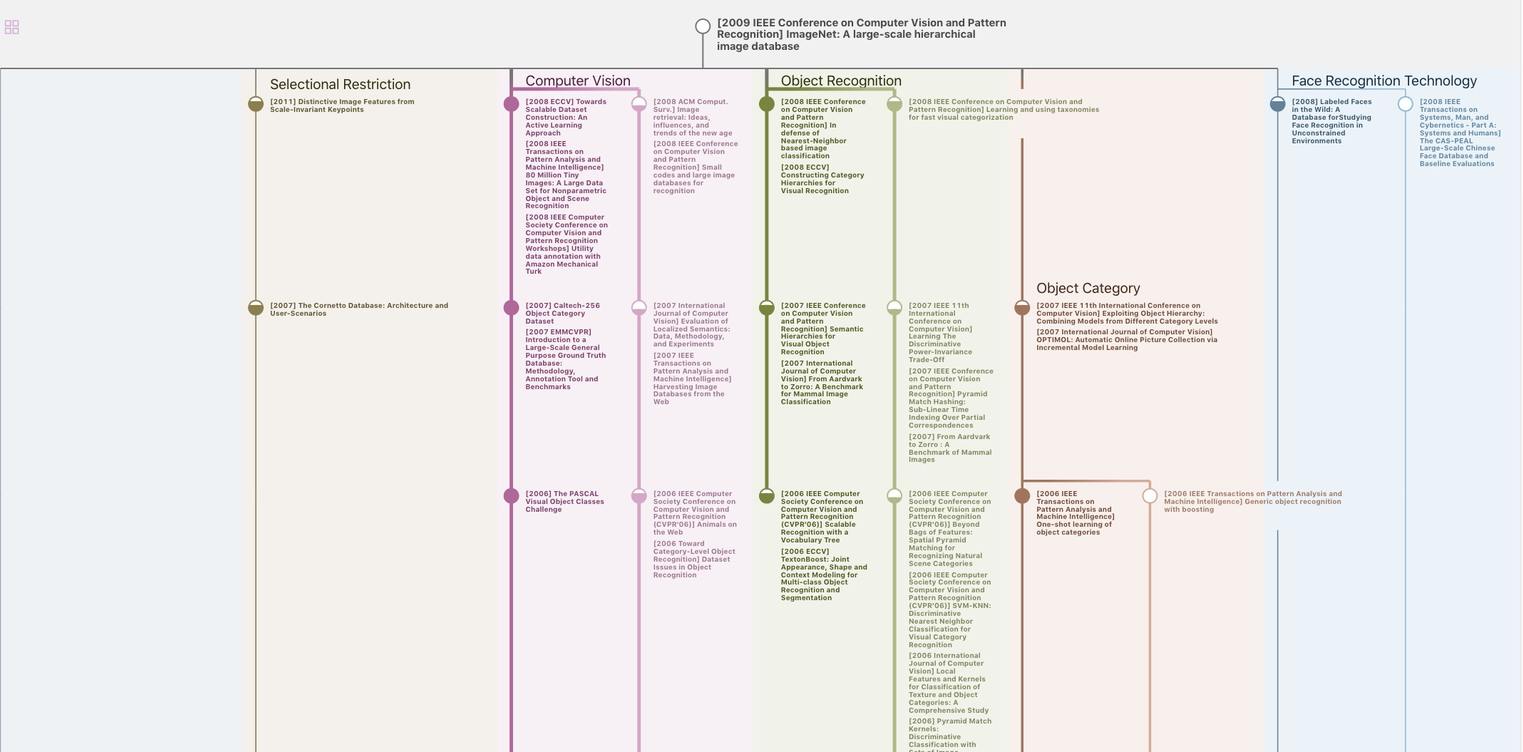
生成溯源树,研究论文发展脉络
Chat Paper
正在生成论文摘要