Semi-Universal Adversarial Perturbations
INDIGO (University of Illinois at Chicago)(2022)
摘要
The present work introduces a framework for learning and selecting semi-universal adversarial perturbations. It relies on a joint estimation of multiple universal adversarial perturbations which are chosen in an unsupervised manner depending on the sample to attack. Two algorithmic solutions, with convergence guarantees under Lipschitz continuity assumptions, are proposed to handle either small scale or large scale datasets. Numerical experiments, conducted on benchmark datasets, support its unifying aspect between universal and specific attacks as the number of perturbations grows. In addition, the learned perturbations display strong patterns indicative of the existing similarities between the training instances of different classes.
更多查看译文
关键词
semi-universal
AI 理解论文
溯源树
样例
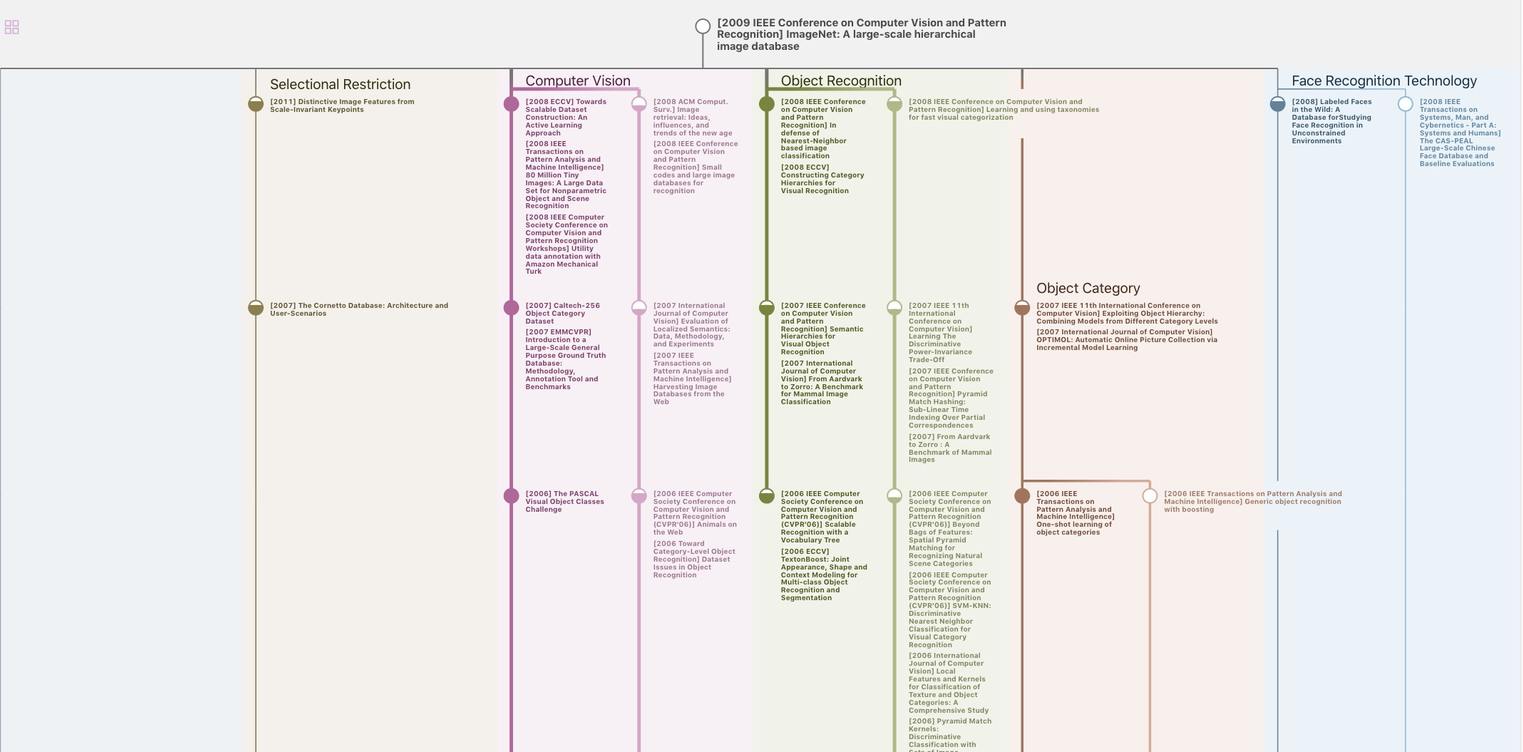
生成溯源树,研究论文发展脉络
Chat Paper
正在生成论文摘要