A Domain-Change Approach to the Semantic Labelling of Remote Sensing Images
crossref(2022)
摘要
<p>For many years, image classification – mainly based on pixel brightness statistics – has been among the<br>most popular remote sensing applications. However, during recent years, many users were more and<br>more interested in the application-oriented semantic labelling of remotely sensed image objects being<br>depicted in given images.<br>In parallel, the development of deep learning algorithms has led to several powerful image<br>classification and annotation tools that became popular in the remote sensing community. In most<br>cases, these publicly available tools combine efficient algorithms with expert knowledge and/or<br>external information ingested during an initial training phase, and we often encounter two alternative<br>types of deep learning approaches, namely Autoencoders (AEs) and Convolutional Neural Networks<br>(CNNs). Both approaches try to convert the pixel data of remote sensing images into semantic maps of<br>the imaged areas.<br>In our case, we made an attempt to provide an efficient new semantic annotation tool that helps in<br>the semantic interpretation of newly recorded images with known and/or possibly unknown content.<br>Typical cases are remote sensing images depicting unexpected and hitherto uncharted phenomena<br>such as flooding events or destroyed infrastructure. When we resort to the commonly applied AE or<br>CNN software packages we cannot expect that existing statistics, or a few initial ground-truth<br>annotations made by an image interpreter, will automatically lead to a perfect understanding of the<br>image content. Instead, we have to discover and combine a number of additional relationships that<br>define the actual content of a selected image and many of its characteristics.<br>Our approach consists of a two-stage domain-change approach where we first convert an image into<br>a purely mathematical ‘topic representation’ initially introduced by Blei [1]. This representation<br>provides statistics-based topics that do not yet require final application-oriented labelling describing<br>physical categories or phenomena and support the idea of explainable machine learning [2]. Then,<br>during a second stage, we try to derive physical image content categories by exploiting a weighted<br>multi-level neural network approach that converts weighted topics into individual application-oriented<br>labels. This domain-changing learning stage limits label noise and is initially supported by an image<br>interpreter allowing the joint use of pixel statistics and expert knowledge [3]. The activity of the image<br>interpreter can be limited to a few image patches.<br>We tested our approach on a number of different use cases (e.g., polar ice, agriculture, natural<br>disasters) and found that our concept provides promising results.  <br>[1] D.M. Blei, A.Y. Ng, and M.I. Jordan, (2003). Latent Dirichlet Allocation, Journal of Machine Learning<br>Research, Vol. 3, pp. 993-1022.<br>[2] C. Karmakar, C.O. Dumitru, G. Schwarz, and M. Datcu (2020). Feature-free explainable data mining<br>in SAR images using latent Dirichlet allocation, IEEE Journal of Selected Topics in Applied Earth<br>Observations and Remote Sensing, Vol. 14, pp. 676-689.<br>[3] C.O. Dumitru, G. Schwarz, and M. Datcu (2021). Semantic Labelling of Globally Distributed Urban<br>and Non-Urban Satellite Images Using High-Resolution SAR Data, IEEE Journal of Selected Topics in<br>Applied Earth Observations and Remote Sensing, Vol. 15, pp. 6009-6068.</p>
更多查看译文
AI 理解论文
溯源树
样例
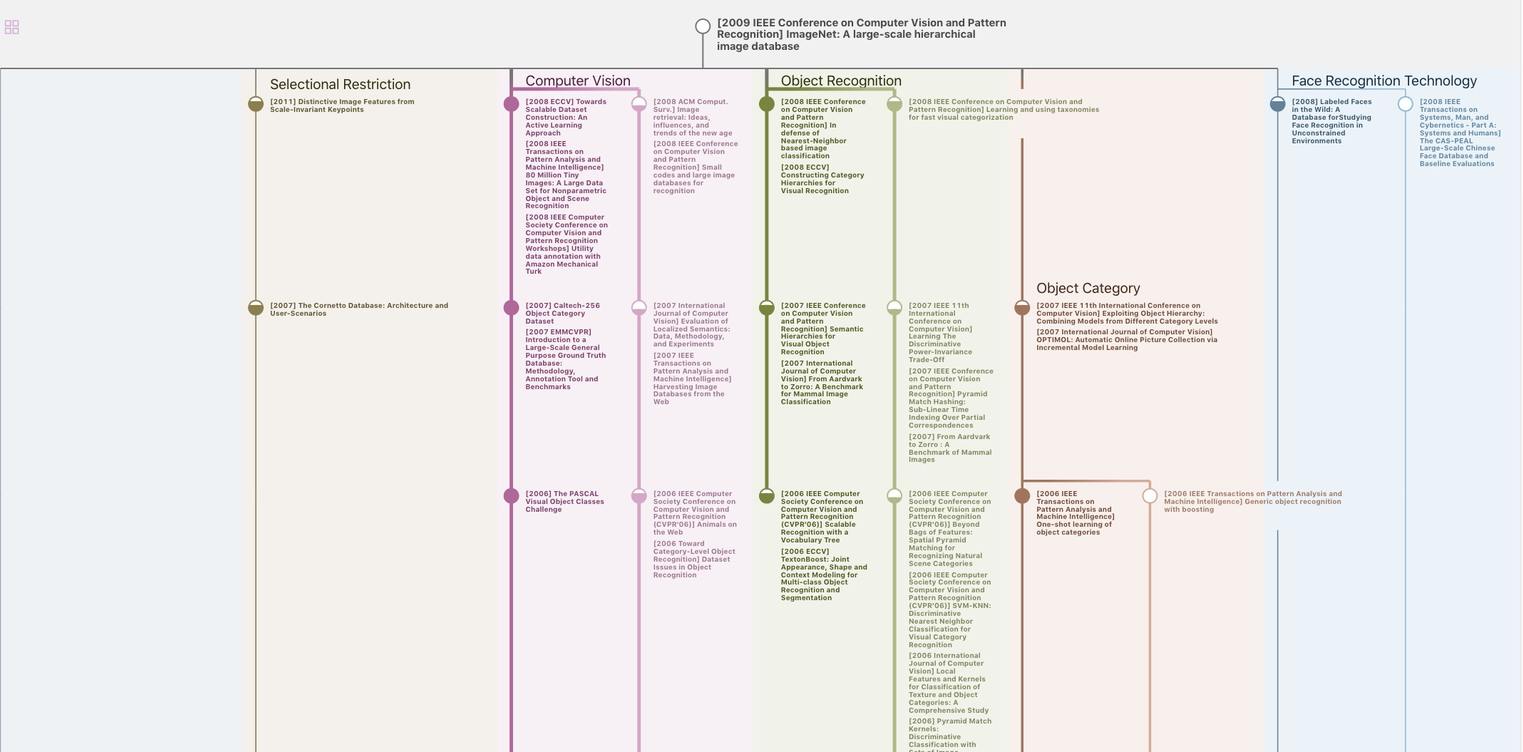
生成溯源树,研究论文发展脉络
Chat Paper
正在生成论文摘要