Can Organic Chemistry Literature Enable Machine Learning Yield Prediction ?
crossref(2022)
摘要
Synthetic yield prediction using machine learning is intensively studied. While previous work focused on an ideal use case, High-Throughput Experiment datasets, predicting yields using literature data remains elusive. We built a large literature- based dataset of more than a thousand reactions, focusing on the activation of carbon-oxygen bonds of phenol derivatives under nickel catalysis. Detailed reaction conditions and associated yields were manually curated and stored in an open- access database. We assessed the performances of state-of-the-art machine learning models on this dataset, and explored their ability to realize predictions on novel publications, coupling partners and substrates. Our work shows that on well- designed yield prediction tasks, machine learning can have practical applications, and provides a unique public database for further improvements of these methods adapted to literature chemical data.
更多查看译文
AI 理解论文
溯源树
样例
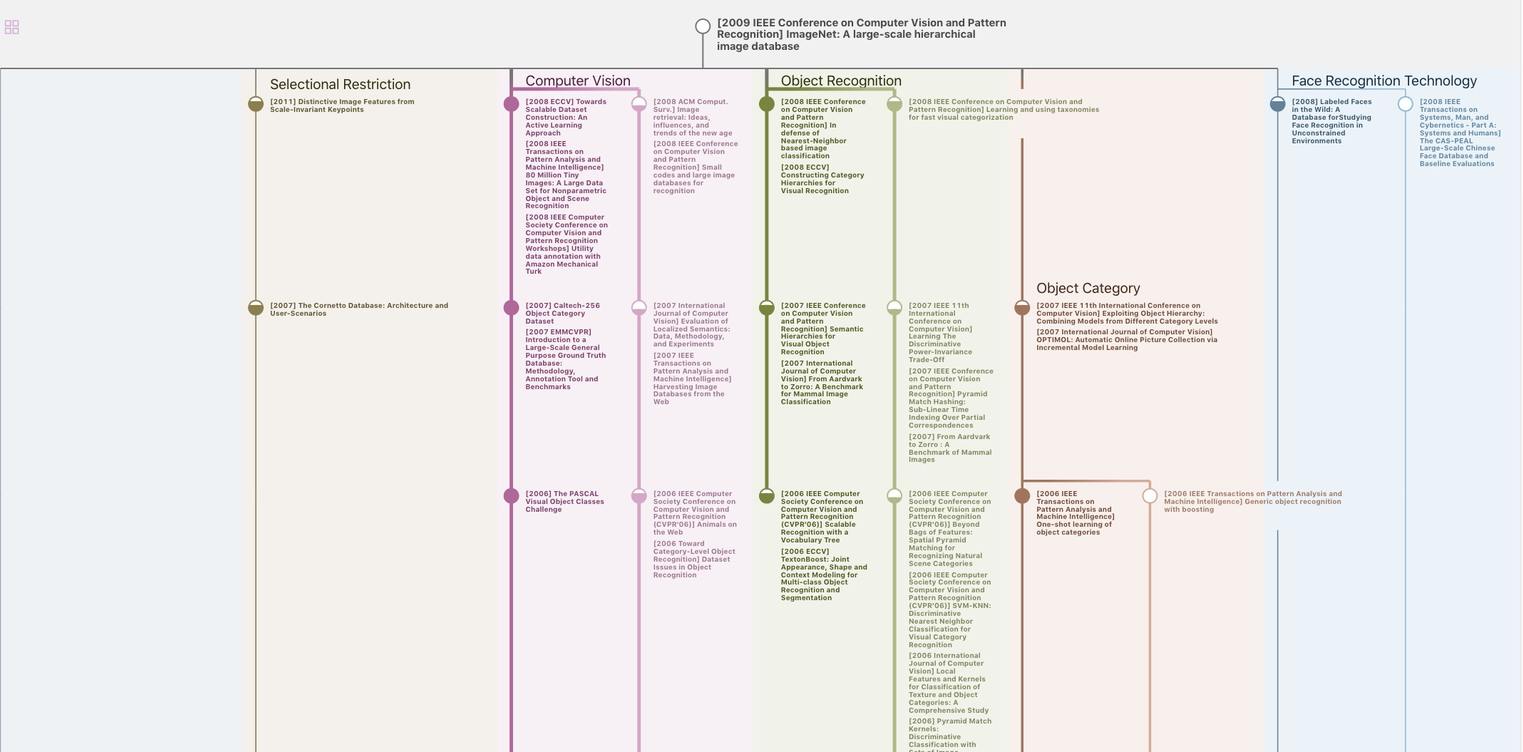
生成溯源树,研究论文发展脉络
Chat Paper
正在生成论文摘要