Prediction Of Postoperative Pulmonary Complications Using Clinical Data And Machine Learning
Research Square (Research Square)(2022)
摘要
Abstract In this study, we aimed to predict postoperative pulmonary complications (PPC) with the help of machine learning (ML) in cardiac operations.We prospectively gathered the preoperative and intraoperative data of 918 patients (random split: n=745 and n=173), who were candidates for elective cardiac surgeries. Patients were observed for any pulmonary complications 24, 48, and 72 hours after the surgery. Feature selection, univariate, and multivariate analysis were performed using an ML algorithm. We obtained the p-value and AUC with 95% CI for each feature and then all features together. We also utilized a Bayesian Network (BN) classifier.Among the 16 selected features, 12 features showed a significant effect on PPC prediction in the testing dataset (p<0.05). Peak expiratory flow rate was the most predictive feature (AUC = 0.75) in the testing dataset. Multivariate analysis showed that our features are highly robust in training (p-value<0.001, AUC=0.73) and testing (p-value=0.002, AUC=0.70) datasets. BN classifier showed that type of surgery, opium usage, renal dysfunction, and history of chronic obstructive pulmonary diseases have the highest conditional probability with the occurrence of PPC.In conclusion, a combination of preoperative and postoperative features in patients can be used to predict PPCs in patients undergoing cardiac surgeries.
更多查看译文
关键词
postoperative pulmonary complications,machine learning,clinical data,prediction
AI 理解论文
溯源树
样例
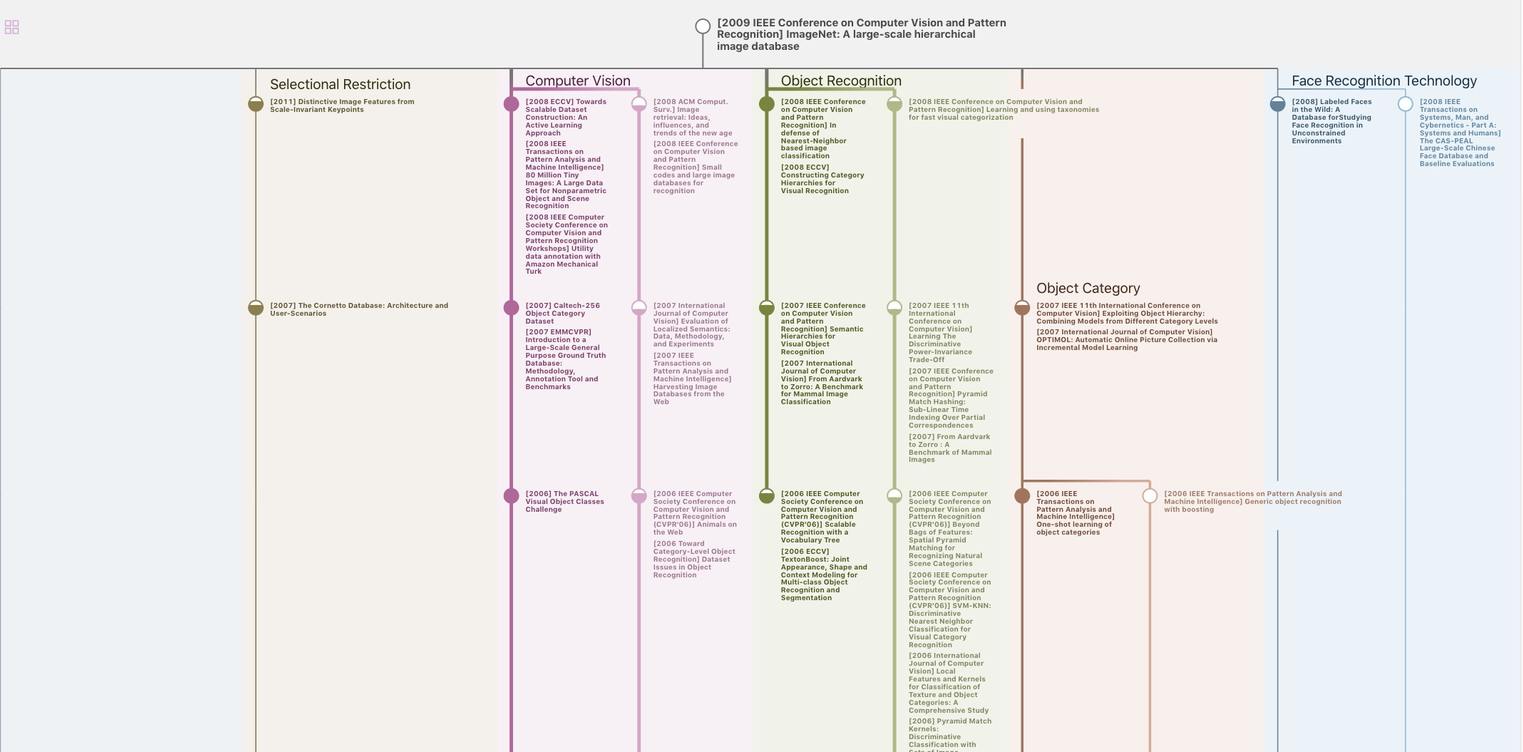
生成溯源树,研究论文发展脉络
Chat Paper
正在生成论文摘要