Odor-GCN: Graph Convolutional Network for Predicting Odor Impressions Based on Molecular Structures
Research Square (Research Square)(2022)
摘要
Abstract Predicting odor impression from molecular structure can help develop olfactory product such as perfumes, cosmetics and fragrances. At present, the methods of odor impression prediction can be divided into three categories: based on molecular physical and chemical characteristics, based on electronic nose, and based on mass spectrometry. In these methods, collecting odor data usually requires expensive equipment or time-consuming chemical and physical experiments. In order to overcome these difficulties, we proposed a molecular structure-based odor impression prediction method, which can be obtained in the existing chemical database (such as PubChem) without additional experiments. This greatly saves the time and cost of obtaining odor data. We believe that the olfactory information of odor is implicit in its molecular structure. First, molecules are represented as graphs, called molecule-graph, that is, atoms are regarded as the vertices of the graph, and covalent bonds are regarded as edges of the graph. Then a graph convolution network (GCN) was designed to extract olfactory information from molecule-graph. The GCN contains three con-volutional layers, an aggregation layer and a fully connected layer. In each graph convolution layer, vertices (atoms) can send message to the vertices (atoms) connected to it. The aggregation layer aggregates all the features of all vertices and calculates their average value. The fully connected layer outputs the odor impression value of the odor. Finally, we predicted the odor impressions (pleasantness , intensity and familiarity) for 476 odor single molecules. The best prediction of Pearson Correlation Coefficient for odor pleasantness impressions was r = 0.52 (p < 0.0000005), which is between the predicted and actual ratings, and the Mean Absolute Error was M AE = 8.53.
更多查看译文
关键词
predicting odor-gcn impressions,graph convolutional network,molecular structures
AI 理解论文
溯源树
样例
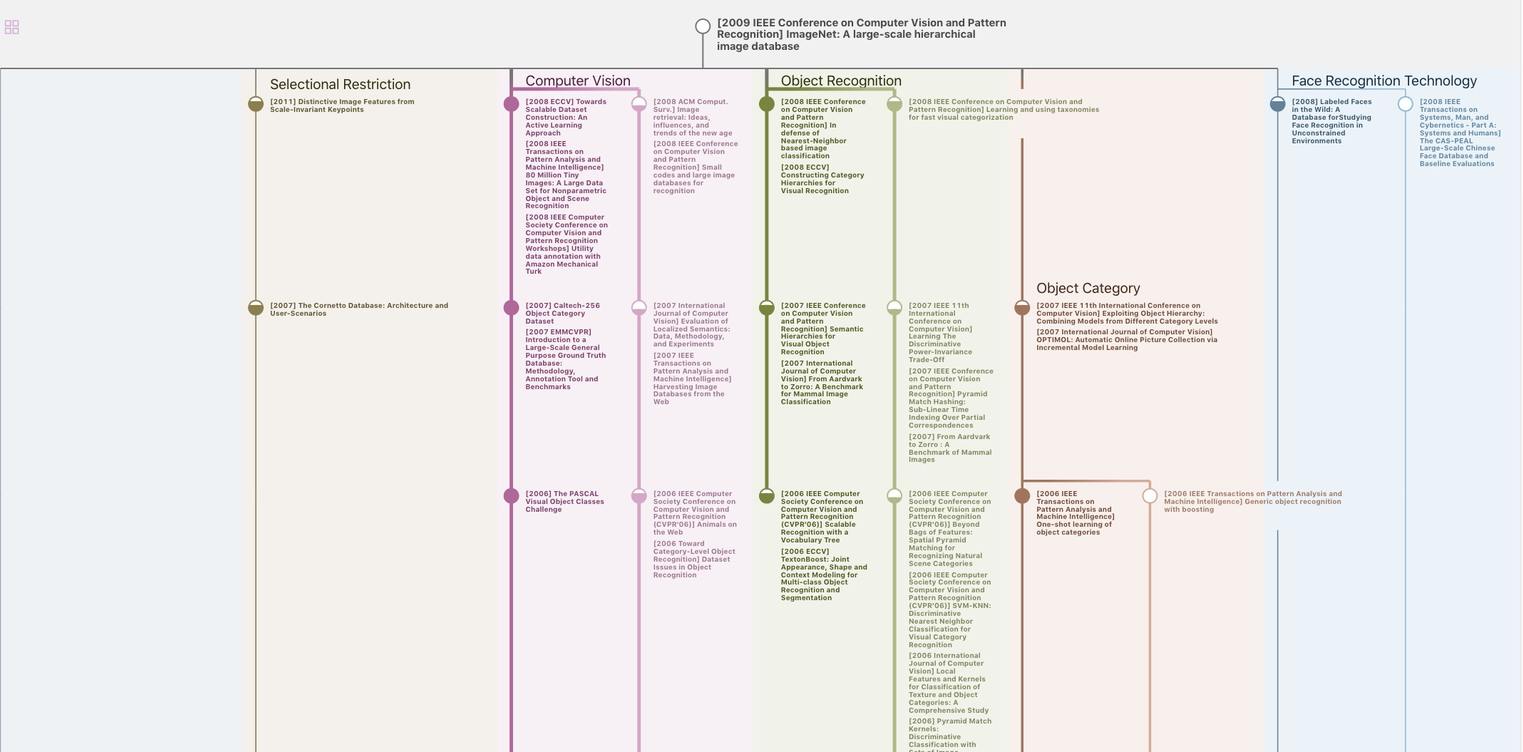
生成溯源树,研究论文发展脉络
Chat Paper
正在生成论文摘要