Radiogenomic Analysis of Vascular Endothelial Growth Factor in Patients With Glioblastoma
crossref(2022)
摘要
Abstract Purpose Vascular endothelial growth factor (VEGF) is very important for glioblastoma (GBM) angiogenesis. This study is to predict VEGF expression in cerebral GBM by radiomic analysis.Methods We analysed the axial T2WI and T1-weighted contrast-enhancement (T1CE) images of preoperative MRI in 217 patients with pathologically diagnosed GBM. Patients were divided into negative and positive VEGF groups, with the latter group further subdivided into low and high expression and analysed by the machine learning model built by the maximum relevance and minimum redundancy (mRMR) algorithm and the extreme gradient boosting (XGBoost) classifier. The area under the receiver operating curve (AUC) and accuracy were calculated for the training and validation sets. Results Positive VEGF in GBM was 63.1% (137/217), with a high expression ratio of 53.3% (73/137). To predict the positive and negative VEGF expression, seven radiomic features were selected with three features from T1CE and four from T2WI. The accuracy and AUC were 0.83 and 0.81, respectively, in the training set, and were 0.73 and 0.74, respectively, in the validation set. To predict high and low levels, seven radiomic features were selected, with two from T1CE, one from T2WI, and four from the data combinations of T1CE and T2WI. The accuracy and AUC were 0.88 and 0.88, respectively, in the training set, and 0.72, and 0.72, respectively, in the validation set. Conclusion The VEGF expression status in GBM can be predicted using a machine learning model. Radiomic features resulting from data combinations of different MRI sequences could be helpful for analysis.
更多查看译文
AI 理解论文
溯源树
样例
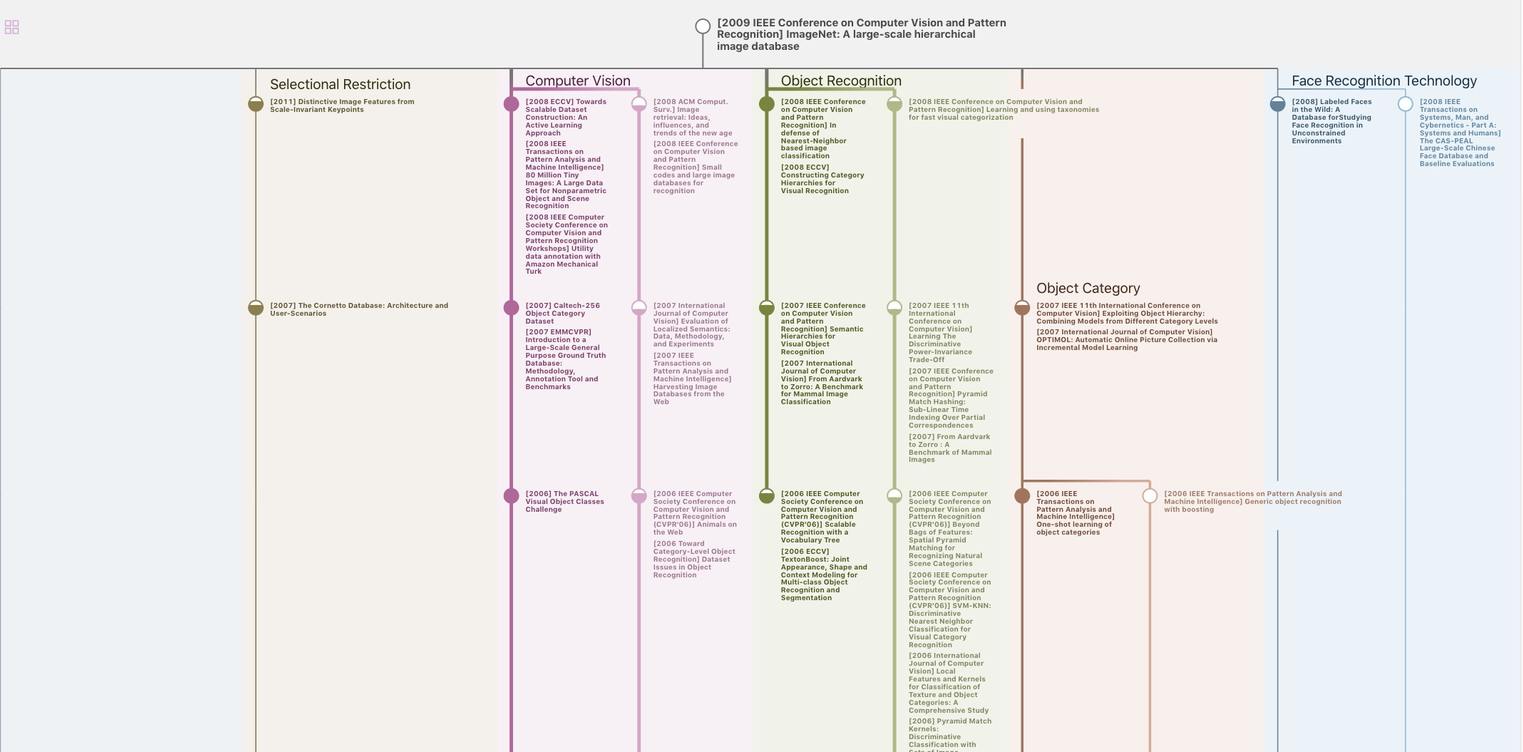
生成溯源树,研究论文发展脉络
Chat Paper
正在生成论文摘要