Decision Letter: Hippocampal Sharp Wave-Ripples and the Associated Sequence Replay Emerge from Structured Synaptic Interactions in a Network Model of Area CA3
crossref(2021)
摘要
Article Figures and data Abstract Editor's evaluation Introduction Results Discussion Materials and methods Data availability References Decision letter Author response Article and author information Metrics Abstract Hippocampal place cells are activated sequentially as an animal explores its environment. These activity sequences are internally recreated (‘replayed’), either in the same or reversed order, during bursts of activity (sharp wave-ripples [SWRs]) that occur in sleep and awake rest. SWR-associated replay is thought to be critical for the creation and maintenance of long-term memory. In order to identify the cellular and network mechanisms of SWRs and replay, we constructed and simulated a data-driven model of area CA3 of the hippocampus. Our results show that the chain-like structure of recurrent excitatory interactions established during learning not only determines the content of replay, but is essential for the generation of the SWRs as well. We find that bidirectional replay requires the interplay of the experimentally confirmed, temporally symmetric plasticity rule, and cellular adaptation. Our model provides a unifying framework for diverse phenomena involving hippocampal plasticity, representations, and dynamics, and suggests that the structured neural codes induced by learning may have greater influence over cortical network states than previously appreciated. Editor's evaluation This manuscript will be of interest to theoretical neuroscientists broadly defined and potentially also to experimentalists investigating the hippocampus. A biologically plausible spiking neural network model of subregion CA3 of the hippocampus is proposed and studied in order to pinpoint the mechanistic sources of sharp wave ripples and replay observed in vivo. https://doi.org/10.7554/eLife.71850.sa0 Decision letter Reviews on Sciety eLife's review process Introduction The hippocampal region plays a pivotal role in spatial and episodic memory (O’Keefe and Nadel, 1978; Morris et al., 1982). The different stages of memory processing (Marr, 1971; Buzsáki, 1989) are associated with distinct brain states and are characterized by distinct oscillatory patterns of the hippocampal local field potential (LFP) (Buzsáki et al., 1983; Colgin, 2016). When rodents explore their environment, place cells of the hippocampus are activated in a sequence that corresponds to the order in which the animal visits their preferred spatial locations (place fields) (O’Keefe and Dostrovsky, 1971). The same sequences of firing activity can also be identified, on a faster time scale, during individual cycles of the 4–10 Hz theta oscillation that dominates the hippocampal LFP in this state (O’Keefe and Recce, 1993; Dragoi and Buzsáki, 2006; Foster and Wilson, 2007). These compressed sequences are thought to be optimal for learning via activity-dependent synaptic plasticity (Jensen and Lisman, 2005; Foster and Wilson, 2007). Other behavioral states such as slow-wave sleep and quiet wakefulness are characterized by the repetitive but irregular occurrence of bursts of activity in the hippocampus, marked by the appearance of sharp waves (Buzsáki et al., 1983; Wilson and McNaughton, 1994) and associated high-frequency (150–220 Hz) ripple oscillations (O’Keefe and Nadel, 1978; Buzsáki et al., 1992) in the LFP. Disruption of SWRs was shown to impair long-term memory (Girardeau et al., 2009; Ego-Stengel and Wilson, 2010; Jadhav et al., 2012; Oliva et al., 2020). The activity sequences observed during exploration are also recapitulated during SWRs (Nádasdy et al., 1999; Kudrimoti et al., 1999; Lee and Wilson, 2002), in the absence of any apparent external driver, and this ‘replay’ can happen either in the same or in a reversed order relative to the original behaviorally driven sequence (Foster and Wilson, 2006; Csicsvari et al., 2007; Diba and Buzsáki, 2007; Davidson et al., 2009; Karlsson and Frank, 2009; Gupta et al., 2010). More specifically, awake replay is predominantly in the ‘forward’ direction near choice points during navigation (Diba and Buzsáki, 2007; Pfeiffer and Foster, 2013), while it is mainly ‘backward’ when the animal encounters a reward (Diba and Buzsáki, 2007). Consequently, while sleep replay was suggested to be involved in memory consolidation, forward and reverse replay in awake animals may contribute to memory recall and reward-based learning, respectively (Carr et al., 2011; Foster, 2017; Pfeiffer, 2017; Olafsdottir et al., 2018). Finally, behavioral tasks with a high memory demand led to an increase in the duration of SWRs, while artificial prolongation of SWRs improved memory (Fernández-Ruiz et al., 2019). For several decades, area CA3 of the hippocampus has been proposed to be a critical site for hippocampal memory operations, mainly due to the presence of an extensive set of modifiable excitatory recurrent connections that could support the storage and retrieval of activity patterns and thus implement long-term memory (Marr, 1971; McNaughton and Morris, 1987; Levy, 1996; Rolls, 1996; Káli and Dayan, 2000). Area CA3 was also shown to be strongly involved in the generation of SWRs and the associated forward and reverse replay (Buzsáki, 2015; Davoudi and Foster, 2019). Several modeling studies have addressed either theta oscillogenesis, learning, sequence replay or ripples; however, a unifying model of how the generation of SWRs and the associated neuronal activity are shaped by previous experience is currently lacking. In the present study, we built a minimal, yet data-driven model of the CA3 network, which, after learning via a symmetric spike-timing-dependent synaptic plasticity rule (Mishra et al., 2016) during simulated exploration, featured both forward and backward replay during autonomously generated sharp waves, as well as ripple oscillations generated in the recurrently connected network of perisomatic interneurons. After validating the model against several in vivo and in vitro results (Buzsáki et al., 1992; Hájos et al., 2013; English et al., 2014; Schlingloff et al., 2014; Stark et al., 2014; Pfeiffer and Foster, 2015; Gan et al., 2017), we took advantage of its in silico nature that made feasible a variety of selective manipulations of cellular and network characteristics. Analyzing the changes in the model’s behavior after these perturbations allowed us to establish the link between learning from theta sequences and the emergence of SWRs during ‘offline’ states, to provide a possible explanation for forward and backward replays, and to disentangle the mechanisms responsible for sharp waves and ripple oscillations. Results To identify the core mechanisms that are responsible for the relationship between learning during exploration and SWRs during rest, we built a scaled-down network model of area CA3 of the rodent hippocampus. In this model, we specifically did not aim to capture all the biological details of hippocampal neurons and circuits; instead, our goal was to reveal and analyze those mechanisms that are essential for the generation of sharp waves, ripple oscillations, and bidirectional activity replay. The main assumptions underlying the construction of our model are listed in Table 1. The complete network consisted of 8000 excitatory pyramidal cells (PCs) and 150 inhibitory parvalbumin-containing basket cells (PVBCs), corresponding roughly to the size of the CA3 area in a 600-µm-thick slice of the mouse hippocampus, which is known to be capable of generating SWRs (Hájos et al., 2013; Schlingloff et al., 2014). These two cell types are known to be indispensable to the generation of SWRs (Rácz et al., 2009; Ellender et al., 2010; English et al., 2014; Schlingloff et al., 2014; Stark et al., 2014; Gulyás and Freund, 2015; Buzsáki, 2015; Gan et al., 2017). All neurons in the network were modeled as single-compartment, adaptive exponential integrate-and-fire (AdExpIF) models whose parameters were fit to reproduce in vitro voltage traces of the given cell types in response to a series of step current injections (‘Materials and methods’ and Figure 1—figure supplement 2). Connections of all types (PC-PC, PC-PVBC, PVBC-PC, PVBC-PVBC) were established randomly using connection type-specific probabilities estimated from anatomical studies. All model parameters are detailed in the ‘Materials and methods’ section and summarized in Tables 2 and 3. Simulations of the network were run in two phases corresponding to spatial exploration and ‘offline’ hippocampal states (slow-wave sleep and awake immobility), respectively. During the exploration phase, the spiking activity of PCs was explicitly set to mimic the firing patterns of a population of place cells during simulated runs on a linear track, and the recurrent connections between PCs evolved according to an experimentally constrained, spike-timing-dependent plasticity (STDP) rule (see below). In the subsequent offline phase, we recorded the spontaneous dynamics of the network in the absence of structured external input (or plasticity), analyzed the global dynamics (including average firing rates and oscillations), and looked for the potential appearance of sequential activity patterns corresponding to the previous activation of place cells during exploration, often referred to as ‘replay.’ The main features of the model are summarized in Figure 1. Figure 1 with 2 supplements see all Download asset Open asset Overview of learning and the spontaneous generation of sharp wave-ripples (SWRs) and sequence replay in the model. (A) Tuning curves (Equation (1)) of exemplar place cells covering the whole 3-m-long linear track. (B) Broad, symmetric spike-timing-dependent plasticity (STDP) kernel used in the learning phase. The time constant was fit directly to experimental data from Mishra et al., 2016. (C) Learned excitatory recurrent weight matrix. Place cells are ordered according to the location of their place fields; neurons with no place field in the environment are scattered randomly among the place cells. Actual dimensions are 8000 * 8000, but, for better visualization, each pixel shown represents the average of an 80 * 80 square. (D) Pyramidal cell (PC) raster plot is shown in the top panel, color-coded, and ordered as the place fields in (A). PC population rate (middle), and local field potential (LFP) estimate (bottom panel), corresponding to the same time period. (E) Posterior matrix of the decoded positions from spikes within the high activity period shown in (D). Gray lines indicate the edges of the decoded, constant velocity path. Recurrent weights are learned during exploration via a symmetric STDP rule During exploration, half of the PCs had randomly assigned, overlapping place fields in the simulated environment, characterized by Gaussian spatial tuning curves, whereas the others fired at low rates in a spatially nonspecific manner (‘Materials and methods’ and Figure 1A). During simulated unidirectional runs along a 3-m-long linear track, these tuning curves, modulated by theta oscillation and phase precession, gave rise to generated spike trains similar to those observed for real place cells under similar conditions (‘Materials and methods’ and Figure 1—figure supplement 1). The simulated spike trains served as inputs for STDP, which was characterized by the broad, symmetric kernel observed in pairs of CA3 PCs recorded in hippocampal slices (Figure 1B; Mishra et al., 2016). The anatomical connectivity of PCs was sparse and random, assuming 10% PC-PC connection probability (Lisman, 1999; Andersen et al., 2007), and only these preexisting connections were allowed to evolve (‘Materials and methods’). The most prominent feature of the learned recurrent excitatory synaptic weight matrix was its highly organized structure (Figure 1C). Relatively few strong (>1 nS) synapses near the diagonal (representing pairs of cells with overlapping place fields) emerged from a background of much weaker connections. Similar symmetric weight structures have been used in continuous ‘bump’ attractor models of various neural systems such as head direction cells, place cells, and parametric working memory (Zhang, 1996; Samsonovich and McNaughton, 1997; Káli and Dayan, 2000; Compte et al., 2000). In these studies, the weights were typically either imposed or learned using rate-based (not STDP) learning rules, and led to stationary patterns of activity in the absence of external input. By contrast, most previous spiking neuron models of sequence learning used temporally asymmetric STDP rules, resulting in a weight structure dominated by feedforward chains (each neuron giving the strongest input to other neurons that follow it in the spatial sequence), and sequential activity patterns that follow these chains of strong connections (Jahnke et al., 2015). In order to reveal the combined effect of learned, essentially symmetric connections and realistic cell-type-specific neuronal spike responses, we next explored the spontaneously generated activity patterns in our full network model. The dynamics of the network model reproduce key features of SWRs and replay The sparse recurrent excitatory weight matrix resulting from the phenomenological exploration (Figure 1C) was used directly in network simulations mimicking resting periods, in which (with the exception of the simulations shown later in Figure 2C) PCs received only spatially and temporally unstructured random synaptic input (‘Materials and methods’). The maximal conductances of the other types of connections in the network (the weights of PC-PVBC, PVBC-PC, and PVBC-PVBC connections as well as the weight of the external random input) were optimized using an evolutionary algorithm with network-level objectives, which included physiological PC population firing rates, suppressed gamma (30–100 Hz) oscillation in the PC population, and strong ripple oscillation in the PVBC population (see ‘Materials and methods’ for details). Figure 2 with 2 supplements see all Download asset Open asset Forward and backward replay events, accompanied by ripple oscillations, can occur spontaneously but can also be cued. (A) Pyramidal cell (PC) raster plot of a 10-s-long simulation, with sequence replays initiating at random time points and positions and propagating either in forward or backward direction on the top panel. PC population firing rate is plotted below. Dashed vertical black lines indicate the periods marked as sustained high-activity states (above 2 Hz for at least 260 ms), which are submitted to automated spectral and replay analysis. (B) Estimated local field potential (LFP) in the top panel and its time-frequency representation (wavelet analysis) below. (C) Forward and backward sequence replays resulting from targeted stimulation (using 200-ms-long 20 Hz Poisson spike trains) of selected 100-neuron subgroups, indicated by the black rectangles in the raster plots. (C1) Example of cued forward replay. PC raster plot is shown at the top with PC population rate, LFP estimate, and its time-frequency representation (wavelet analysis) below. (C2) Same as (C1), but different neurons are stimulated at the beginning, leading to backward replay. Spontaneously generated activity in the network consisted of alternating periods of low activity (mean PC rates below 1 Hz) and high activity (mean PC rates around 3.5 Hz), which resembled the recurring appearance of sharp wave events during slow-wave sleep and quiet wakefulness (Figure 2A). Similar to experimental sharp waves, high-activity events in the model were accompanied by transient oscillations in the ripple frequency range (Figure 2B; Buzsáki, 1986; Buzsáki et al., 1992; Foster and Wilson, 2006; Buzsáki, 2015). We calculated an estimate of the LFP by summing the synaptic inputs of a small, randomly selected subset of PCs (Mazzoni et al., 2008), and found that ripple oscillations were reliably present in this signal during the high-activity periods (Figures 1D and 2B). When we plotted the spike times of all PCs with place cells ordered according to the location of their place fields on the track during the learning phase, it became clear that place cell sequences were replayed during simulated SWRs, while activity had no obvious structure in the low-activity periods between SWRs (Figure 2A). Interestingly, replay of place cell sequences could occur in either the forward or the backward direction relative to the order of activation in the learning phase (Figure 2A). Importantly, apart from some initial transients, simultaneous forward and backward replay was never observed. This is because simultaneous replay in both directions induces competition (mediated by global feedback inhibition) between the two active populations, and one of the sequences always dies out after a short period (this is also because mutual support through recurrent excitation diminishes as the two active populations move further away from each other). This is similar to the competitive behavior observed in ‘bump attractor’ models (Dayan and Abbott, 2001). These qualitative observations of sequence replay were confirmed by analyzing high-activity (SWR) periods with a Bayesian place decoding and path fitting method using spatial tuning curves introduced for spike train generation in the exploration part (‘Materials and methods’ and Figure 1E; Davidson et al., 2009; Karlsson and Frank, 2009; Olafsdottir et al., 2018). Interestingly, our network model also reproduced the broad, long-tailed step-size distribution in the sequence of decoded locations during SWRs, as observed experimentally by Pfeiffer and Foster, 2015; Figure 2—figure supplement 1. It was also possible to ‘cue’ replay by giving an additional external stimulation to a small subpopulation (n=100) of PCs that had overlapping place fields in the learned environment. In this case, sequence replay started at the location corresponding to the cells that received extra stimulation (Figure 2C). This feature may explain why awake replay tends to be forward when the animal is planning a trajectory from its current location and backward at the goal location. At the level of single cells, we found that both PCs and PVBCs received approximately balanced excitation and inhibition during SWR events, and inhibitory currents were modulated at the ripple frequency in these periods (Figure 2—figure supplement 2C and D). Excitatory inputs dominated between SWRs and during the initiation of SWR events. Only a small minority of PCs fired in individual ripple cycles, while the participation of PVBCs was much higher (but not complete), resulting in a mean PVBC firing rate of ∼65 Hz during the SWRs, which is much higher than their baseline rate, but significantly below the ripple frequency (Figure 2—figure supplement 2A and B). All of these findings were consistent with experimental results in vitro (Hájos et al., 2013; Schlingloff et al., 2014) and in vivo (Varga et al., 2012; English et al., 2014; Hulse et al., 2016; Gan et al., 2017). The firing of individual PCs appears to differ across experimental conditions, with mostly single spikes in vitro (Hájos et al., 2013; Schlingloff et al., 2014) and occasional burst firing and a lognormal firing rate distribution in vivo (Mizuseki and Buzsáki, 2013). In our simulations, many PCs fired more than one spike per SWR, but these were not bursts in the usual sense as even the shortest ISIs were over 5 ms. The firing rate distribution was skewed, although not particularly long-tailed (Figure 2—figure supplement 2B and C). SWRs and replay are robust when recurrent excitation is varied To show that our network model reproduces the wide range of experimental findings presented above in a robust manner, we ran a sensitivity analysis of several parameters. We started by studying the effects of the recurrent excitatory weights, which are the link between exploratory and resting dynamics. To this end, we ran simulations with PC-PC weights that were systematically up- or downscaled after the learning phase, and automatically evaluated various features of the network dynamics, such as population-averaged firing rates, the presence of sequence replay, as well as significant peaks in the ripple frequency range in the power spectra as shown before (‘Materials and methods’ and Figure 3). The network with PC-PC weights multiplied by 0.8 displayed a low-activity, noisy state with severely reduced mean PC firing rate, no sequence replay, and no clear oscillation (Figure 3A1). At the 0.9 multiplier level, sequence replays started to appear, but were less frequent than in the baseline model (Figure 3A2). As the PC-PC synaptic weights were scaled up, sequence replays became faster (completed in a shorter time) and occurred more often (Figure 3A3), a behavior that was stable and realistic up to the 1.5 multiplier level. Ripple oscillations had higher power and appeared at lower multiplier levels in the PVBC population than in the PC population (Figure 3—figure supplement 1), suggesting that they originate from the PVBCs and propagate to the PCs, in agreement with theories based on experimental findings (Buzsáki et al., 1992; Ylinen et al., 1995; Rácz et al., 2009; Schlingloff et al., 2014; Stark et al., 2014; Gan et al., 2017). Overall, the qualitative behavior of our model was similar in a fairly wide range of recurrent excitation, although our baseline model was relatively close to the lower end of this range. Figure 3 with 1 supplement see all Download asset Open asset Sharp waves, ripple oscillations, and replay are robust with respect to scaling the recurrent excitatory weights. (A) Pyramidal cell (PC) raster plots on top and PC population rates at the bottom for E-E scaling factors 0.9 (A1), 0.95 (A2), and 1.05 (A3). (Scaling factor of 1.0 is equivalent to Figure 2A.) Dashed vertical black lines have the same meaning as in Figure 2A. (B) Analysis of selected indicators of network dynamics across different E-E weight scaling factors (0.8–1.2). Mean PC (blue) and parvalbumin-containing basket cell (PVBC) (pink) population rates are shown on top. The frequency of significant ripple oscillations (black) and the percentage of power in the ripple frequency range (red) in the estimated local field potential (LFP) is shown at the bottom. Errors bars indicate standard deviation and are derived from simulations with five different random seeds. See also Figure 3—figure supplement 1. Multiple environments can be learned and replayed Next, we showed that it is possible to store the representations of multiple environments in the weight matrix, and this change does not fundamentally alter the network dynamics (Figure 4). In particular, we simulated experience and STDP-based learning in two linear environments with a different but overlapping random set of place cells (Figure 4A). The resulting population-level dynamics was quite similar to the one following experience in a single environment, but, during each SWR event, a place cell sequence from either one or the other environment was reactivated (Figure 4B and C). Learning two sequences with the same additive symmetric (nondecreasing) STDP rule led to stronger PC-PC synapses on average (Figure 4A4), which resulted in a higher overall mean PC rate (Figure 4B and D). As a consequence, detectable sequence replays and significant ripple oscillations appeared at lower PC-PC weight multiplier levels (Figure 4D). Figure 4 Download asset Open asset Two distinct environments can be learned and replayed by the network. (A) Learned excitatory recurrent weight matrices. (A1) Weights after learning the first environment. Note that the matrix appears random because neurons are arranged according to their place field location in the second environment, which has not been explored at this point. (A3) Weights after learning in the second environment. (A2, A4) Distribution of nonzero synaptic weights in the learned weight matrices in (A1) and (A2), respectively. (B) Pyramidal cell (PC) raster plots: in the top panel, neurons are ordered and colored according to the first environment; in the middle panel, neurons are ordered and colored according to the second environment; and PC population rate is shown at the bottom (see Figure 2A) from a simulation run with 0.9* the modified weight matrix shown in (A3). (C) Posterior matrices of decoded positions from spikes (see Figure 1E) within two selected high-activity periods (8th and 10th from B). From left to right: decoding of replay in first environment (eighth event from B) according to the first (significant) and second environment; decoding of replay in second environment (10th event from B) according to the first and second (significant) environment. (D) Analysis of selected network dynamics indicators across different E-E weight scaling factors (0.7–1.1) as in Figure 3B. Manipulating the model reveals mechanisms of SWR generation and sequence replay Our network model, equipped with structured recurrent excitation resulting from learning, was able to robustly reproduce recurring sharp wave events accompanied by bidirectional sequence replay and ripple oscillations. Next, we set out to modify this excitatory weight matrix to gain a more causal understanding of how the learned weight pattern is related to the emergent spontaneous dynamics of the network. Symmetric STDP rule is necessary for bidirectional replay First, in order to gauge the significance of the experimentally determined symmetric STDP rule, we changed the STDP kernel to the classical asymmetric one that characterizes many other connections in the nervous system (Figure 5A–C; Bi and Poo, 1998; Gerstner et al., 2014). In this case, the learned weight matrix was reminiscent of the feedforward chains that characterized several of the earlier models of hippocampal replay (Jahnke et al., 2015; Chenkov et al., 2017; Theodoni et al., 2018). We found that this weight structure also supported the generation of SWR events and the reactivation of learned sequences in our model; however, crucially, sequence replays occurred only in the forward direction (Figure 5D and E). Theodoni et al., 2018 presented a thorough analysis of the relationship between the shape of the (asymmetric) plasticity kernel and sequence replay in a rate-based model, and thus we shifted our focus towards different modifications. Figure 5 Download asset Open asset Learning with an asymmetric spike-timing-dependent plasticity (STDP) rule leads to the absence of backward replay. (A) Asymmetric STDP kernel used in the learning phase. (B) Learned excitatory recurrent weight matrix. (C) Distribution of nonzero synaptic weights in the weight matrix shown in (B). (D) Pyramidal cell (PC) raster plot on top and PC population rate at the bottom (see Figure 2A) from a simulation run with the weight matrix shown in (B). (E) Posterior matrix of the decoded positions from spikes (see Figure 1E) within a selected high-activity state (sixth one from D). (F) Analysis of selected network dynamics indicators across different E-E weight scaling factors (0.8–1.2) as in Figure 3B. The structure rather than the statistics of recurrent excitatory weights is critical for SWRs Inspired by the observation that many network-level properties, such as single PC firing rates, burst index, and participation in SWR events, follow a skewed, lognormal distribution in vivo (Mizuseki and Buzsáki, 2013), Omura et al., 2015 built a network model with recurrent excitatory weights following a lognormal distribution. Their network with unstructured, but lognormally distributed, recurrent synaptic strengths reproduced most of the in vivo observations of Mizuseki and Buzsáki, 2013; however, no sequence replay or ripple oscillation was involved. In the network presented here, the distribution of PC-PC weights is the result of the application of STDP to the generated spike trains and does not strictly follow a lognormal distribution, although it has a similar long tail (Figure 6D2). In order to establish whether the overall distribution or the fine structure of the weights is the key determinant of neural dynamics in our model, we performed two more drastic perturbations of the recurrent weight matrix itself, starting from the version established in the learning phase of our baseline model (Figure 1C). Figure 6 Download asset Open asset Altering the structure of recurrent excitatory interactions changes the network dynamics but altering the weight statistics has little effect. (A1) Binarized (largest 3% and remaining 97% nonzero weights averaged separately) recurrent excitatory weight matrix. (Derived from the baseline one shown in Figure 1C.) (A2) Distribution of nonzero synaptic weights in the learned weight matrix shown in (A1). (B) Pyramidal cell (PC) raster plot on top and PC population rate at the bottom (see Figure 2A) from a simulation run with 1.1* the binarized weight matrix shown in (A). (C) Analysis of selected network dynamics indicators across different E-E weight scaling factors (0.9–1.3) as in Figure 3B. (D1) Column-shuffled recurrent excitatory weight matrix. (Derived from the baseline one shown in Figure 1C.) (D2) Distribution of nonzero synaptic weights in the weight matrix shown in (D1) (identical to the distribution of the baseline weight matrix shown in Figure 1C). (E) PC raster plot on top and PC population rate at the bottom from a simulation run with the shuffled weight matrix shown in (D1). (F) Analysis of selected network dynamics indicators across different E-E weight scaling factors (1.0–4.0) as in Figure 3B. Note the significantly extended horizontal scale compared to other cases. Our first perturbation kept the structure of the interactions and the overall mean weight intact, but com
更多查看译文
关键词
Working Memory,Hippocampus,Synaptic Plasticity,Neural Synchrony
AI 理解论文
溯源树
样例
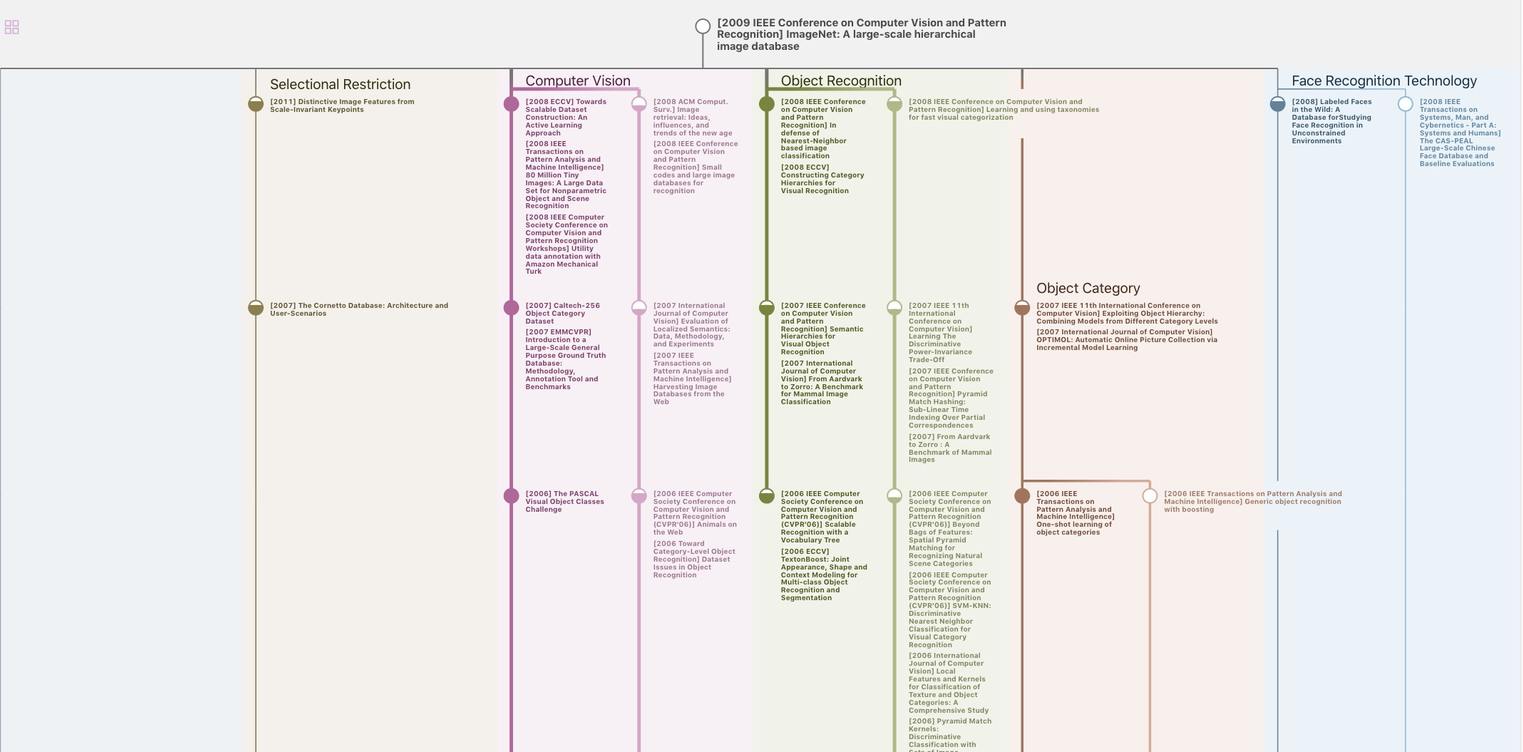
生成溯源树,研究论文发展脉络
Chat Paper
正在生成论文摘要