Leveraging Values to Promote Adherence to Endocrine Therapy among Breast Cancer Survivors: A Mixed-Methods Investigation
crossref(2022)
摘要
Background: Improving medication adherence represents one of the greatest behavioral challenges in medicine. Personal values are an under-examined source of motivation for adhering to medication. This secondary analysis leverages a mixed-method approach to understanding a novel, online, values-based intervention (‘REACH’) for promoting endocrine therapy (ET) adherence among breast cancer survivors, using data from a randomized trial. Methods: Breast cancer survivors with challenges taking ET (n=88) were randomized 1:1 to ET Education+Values (REACH) or Education-only online interventions. This report focuses on the three values strategies used in REACH to promote ET adherence: affirming health-related values, using values-based perspective-taking to motivate adherence, and creating a personalized cue-to-action values sticker for participants’ ET pillbox. Responses to these strategies were analyzed using Linguistic Inquiry and Word Count (LIWC) and structured exit interviews. Further, in both conditions, positive and negative affective states were repeatedly evaluated. Affective responses to REACH were used to predict objective ET adherence. Results: During REACH values exercises, LIWC showed greater use of positive emotion words than negative emotion words, and thematic coding of interviews identified mostly positive responses to values content. Compared to Education, REACH quantitatively led to less tense and sad and more loving states. Within REACH, a large spike in guilt and drop in enthusiasm occurred after the perspective-taking values exercise, with strong recovery after the values sticker exercise. Greater area-under-the-curve for guilt predicted lower subsequent ET adherence. Conclusions: Distinct approaches to linking domain-relevant values to ET adherence produced different affective responses, which in turn predicted ET adherence, informing theory and practice.
更多查看译文
AI 理解论文
溯源树
样例
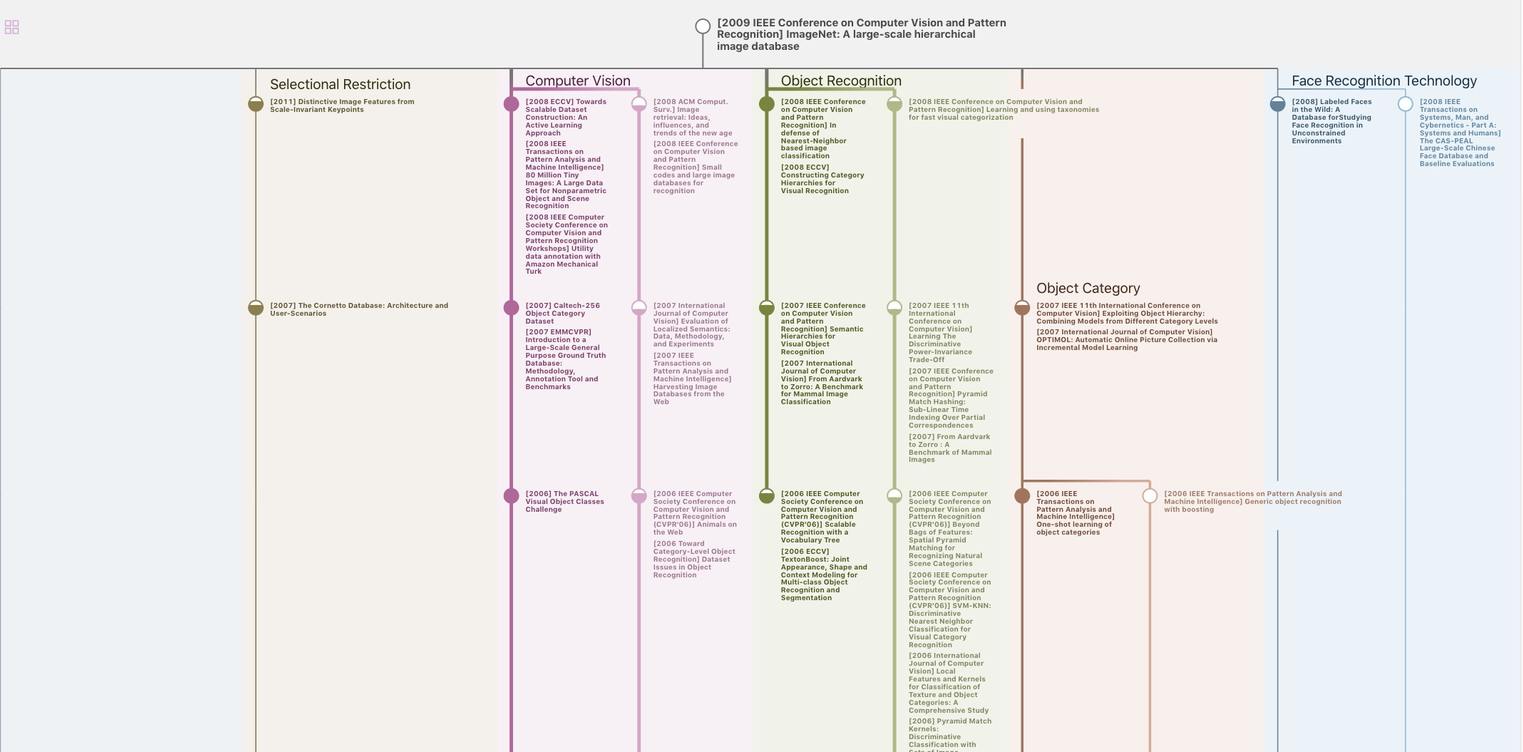
生成溯源树,研究论文发展脉络
Chat Paper
正在生成论文摘要