Hierarchical Bayesian Regression for Multi-Location Sales Transaction Forecasting
CoRR(2023)
摘要
The features in many prediction models naturally take the form of a hierarchy. The lower levels represent individuals or events. These units group naturally into locations and intervals or other aggregates, often at multiple levels. Levels of groupings may intersect and join, much as relational database tables do. Besides representing the structure of the data, predictive features in hierarchical models can be assigned to their proper levels. Such models lend themselves to hierarchical Bayes solution methods that ``share'' results of inference between groups by generalizing over the case of individual models for each group versus one model that aggregates all groups into one. In this paper we show our work-in-progress applying a hierarchical Bayesian model to forecast purchases throughout the day at store franchises, with groupings over locations and days of the week. We demonstrate using the \textsf{stan} package on individual sales transaction data collected over the course of a year. We show how this solves the dilemma of having limited data and hence modest accuracy for each day and location, while being able to scale to a large number of locations with improved accuracy.
更多查看译文
关键词
hierarchical bayesian regression,sales,forecasting,multi-location
AI 理解论文
溯源树
样例
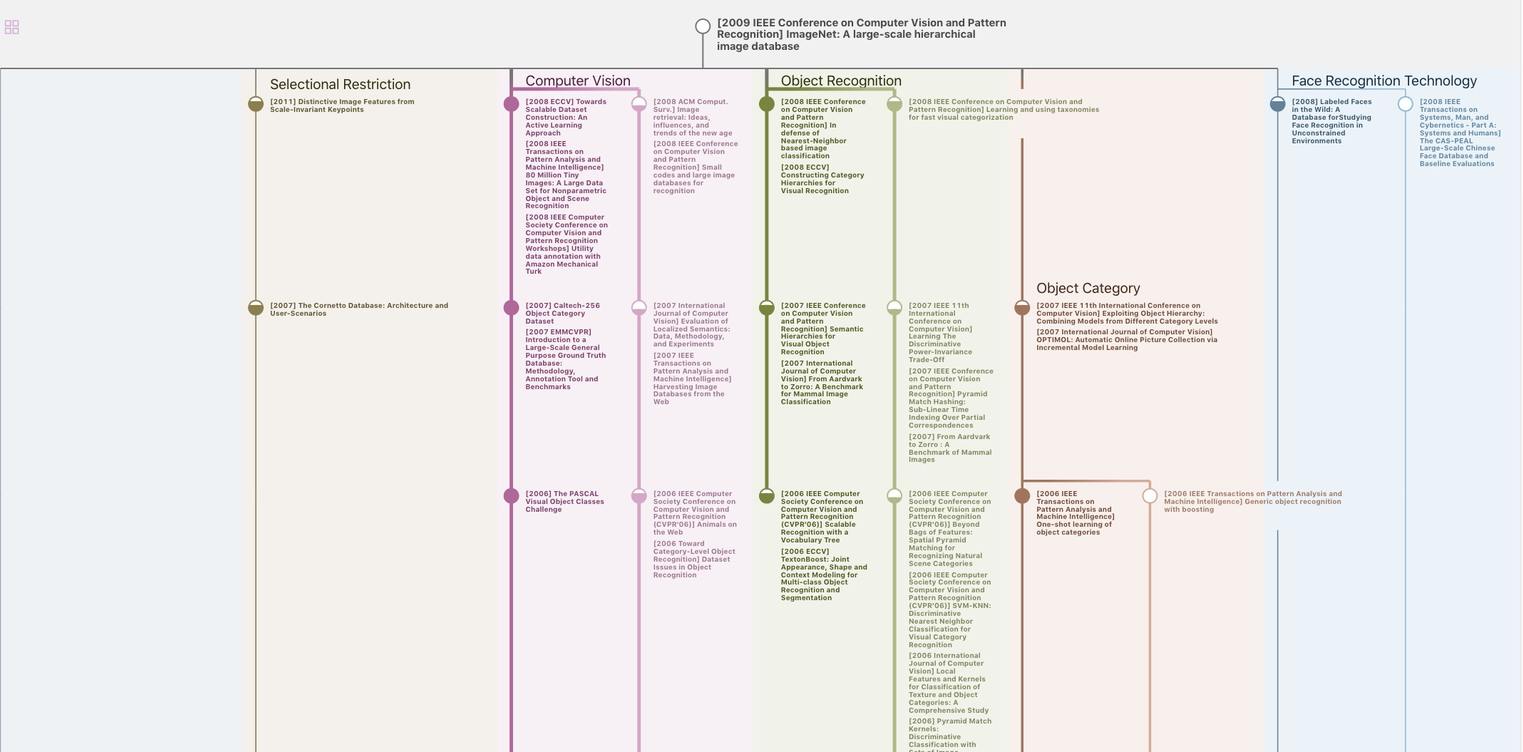
生成溯源树,研究论文发展脉络
Chat Paper
正在生成论文摘要