A Method of Social Context Enhanced User Preferences for Conversational Recommender Systems
Computational Science – ICCS 2023(2023)
摘要
Conversational recommender systems (CRS) can dynamically capture user fine-grained preference by directly asking whether a user likes an attribute or not. However, like traditional recommender systems, accurately comprehending users’ preferences remains a critical challenge for CRS to make effective conversation policy decisions. While there have been various efforts made to improve the performance of CRS, they have neglected the impact of the users’ social context, which has been proved to be valuable in modeling user preferences and enhancing the performance of recommender systems. In this paper, we propose a social-enhanced user preference estimation model (SocialCRS) to leverage the social context of users to better learn user embedding representation. Specifically, we construct a user-item-attribute heterogeneous graph and apply a graph convolution network (GCN) to learn the embeddings of users, items, and attributes. Another GCN is used on the user social context graph to learn the social embedding of users. To estimate better user preference, the attention mechanism is adopted to aggregate the embedding of the user’s friends. By aggregating these users’ embeddings, we obtain social-enhanced user preferences. Through extensive experiments on two public benchmark datasets in a multi-round conversational recommendation scenario, we demonstrate the effectiveness of our model, which significantly outperforms the state-of-the-art CRS methods.
更多查看译文
关键词
conversational recommender systems, user preference, social context
AI 理解论文
溯源树
样例
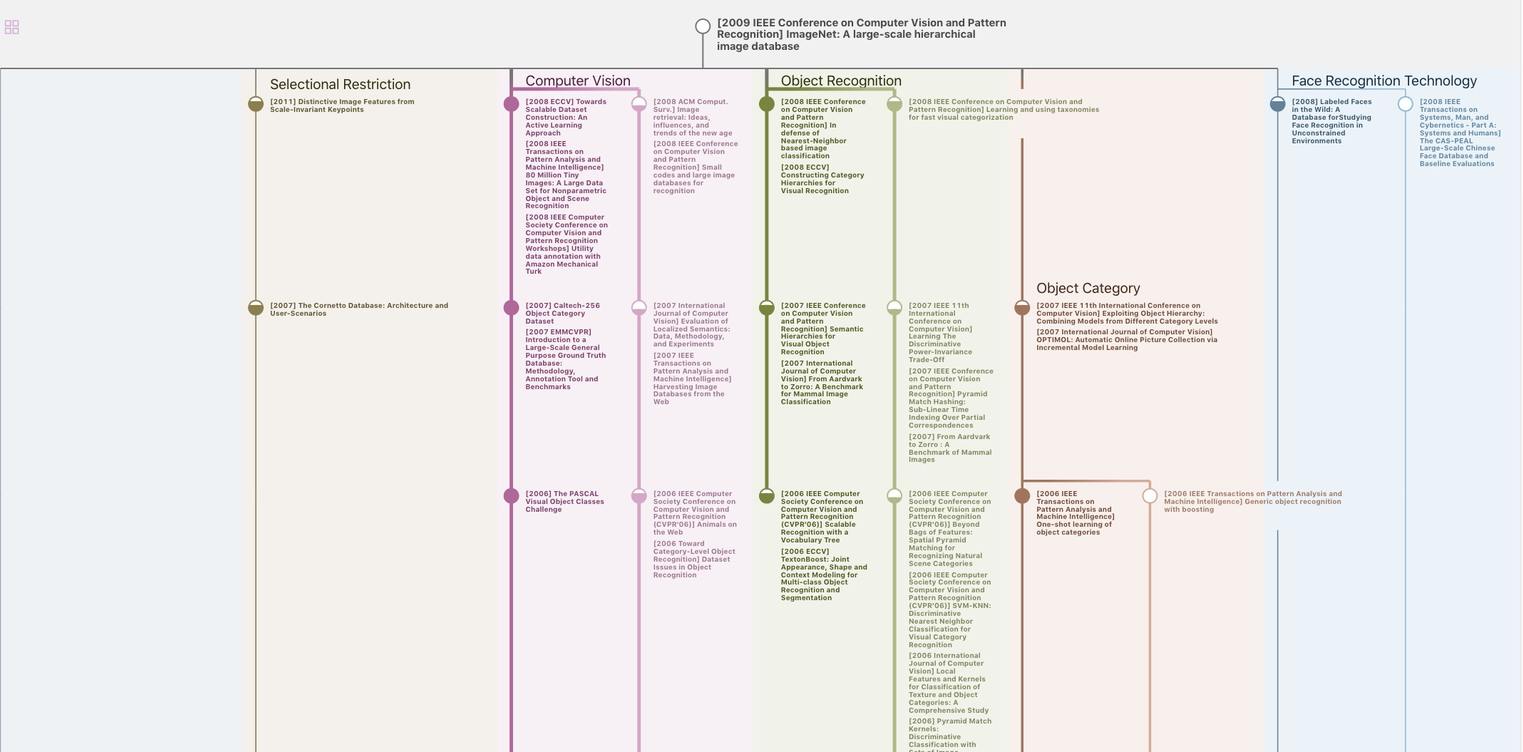
生成溯源树,研究论文发展脉络
Chat Paper
正在生成论文摘要