Learnable Slice-to-volume Reconstruction for Motion Compensation in Fetal Magnetic Resonance Imaging
Bildverarbeitung für die Medizin 2023(2023)
摘要
Reconstructing motion-free 3D magnetic resonance imaging (MRI) volumes of fetal organs comes with the challenge of motion artefacts due to fetal motion and maternal respiration. Current methods rely on iterative procedures of outlier removal, super-resolution (SR) and slice-to-volume registration (SVR). Long runtimes and missing volume preservation over multiple iterations are still challenges for widespread clinical implementation. We envision an end-to-end learnable reconstruction framework that enables faster inference times and that can be steered by downstream tasks like segmentation. Therefore, we propose a new hybrid architecture for fetal brain reconstruction, consisting of a fully differentiable pre-registration module and a CycleGAN model for 3D image-toimage translation that is pretrained on our custom-generated dataset of 209 pairs of low-resolution (LoRes) and high-resolution (HiRes) fetal brain volumes. Our results are evaluated quantitatively with respect to five different similarity metrics. We incorporate the learned perceptual image patch similarity (LPIPS) metric and apply it to quantify volumetric image similarity for the first time in literature. Furthermore, we evaluate the model outputs qualitatively and conduct an expert survey to compare our method’s reconstruction quality to an established approach.
更多查看译文
关键词
magnetic resonance imaging,motion compensation,magnetic resonance,slice-to-volume
AI 理解论文
溯源树
样例
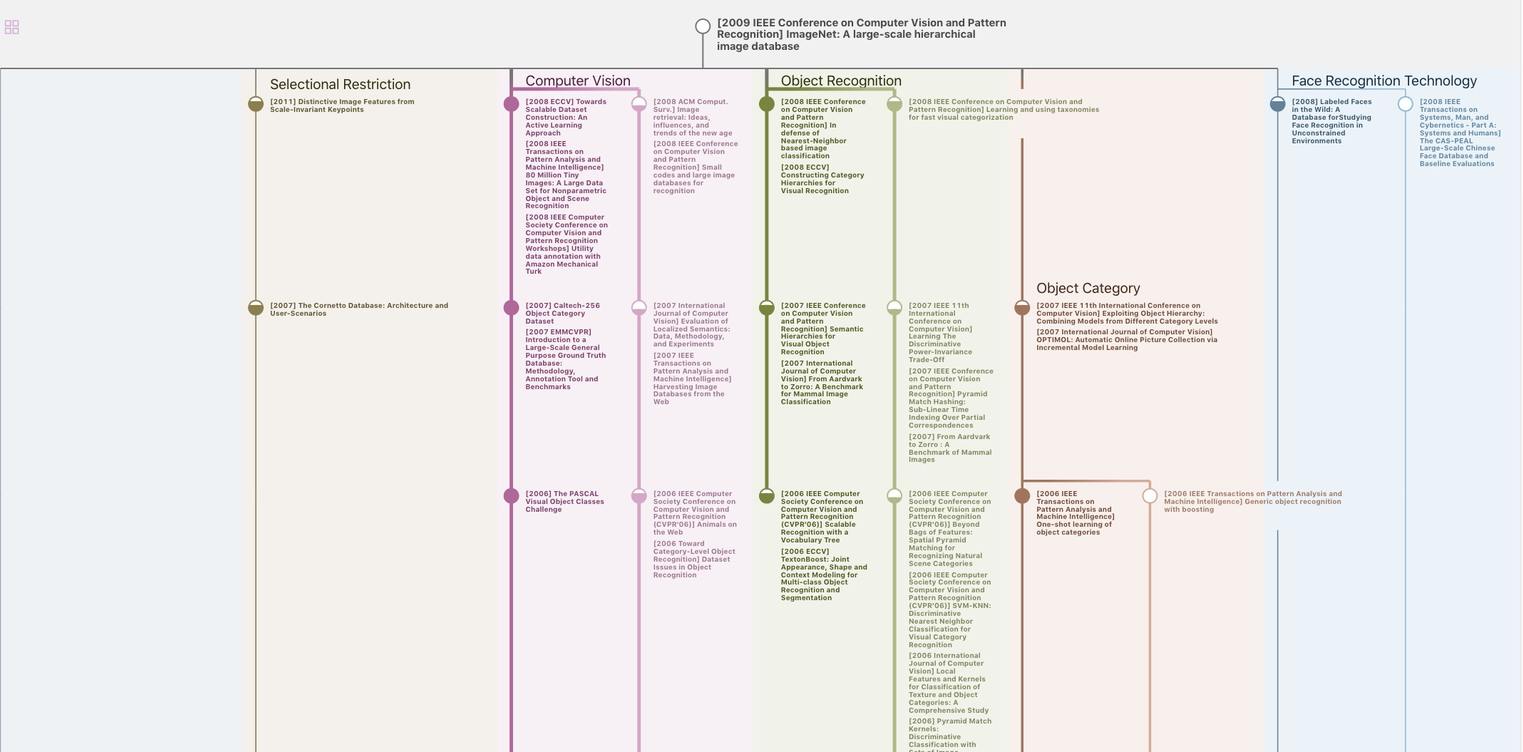
生成溯源树,研究论文发展脉络
Chat Paper
正在生成论文摘要