Improving Patients’ Length of Stay Prediction Using Clinical and Demographics Features Enrichment
Computational Science – ICCS 2023(2023)
摘要
Predicting patients’ length of stay (LOS) is crucial for efficient scheduling of treatment and strategic future planning, in turn reduce hospitalisation costs. However, this is a complex problem requiring careful selection of optimal set of essential factors that significantly impact the accuracy and performance of LOS prediction. Using an inpatient dataset of 285k of records from 14 general care hospitals in Vermont, USA from 2013–2017, we presented our novel approach to incorporate features to improve the accuracy of LOS prediction. Our empirical experiment and analysis showed considerable improvement in LOS prediction with an XGBoost model RMSE score of 6.98 and R2 score of 38.24%. Based on several experiments, we provided empirical analysis of the importance of different feature sets and its impact on predicting patients’ LOS.
更多查看译文
关键词
stay prediction,demographics features enrichment,patients,clinical
AI 理解论文
溯源树
样例
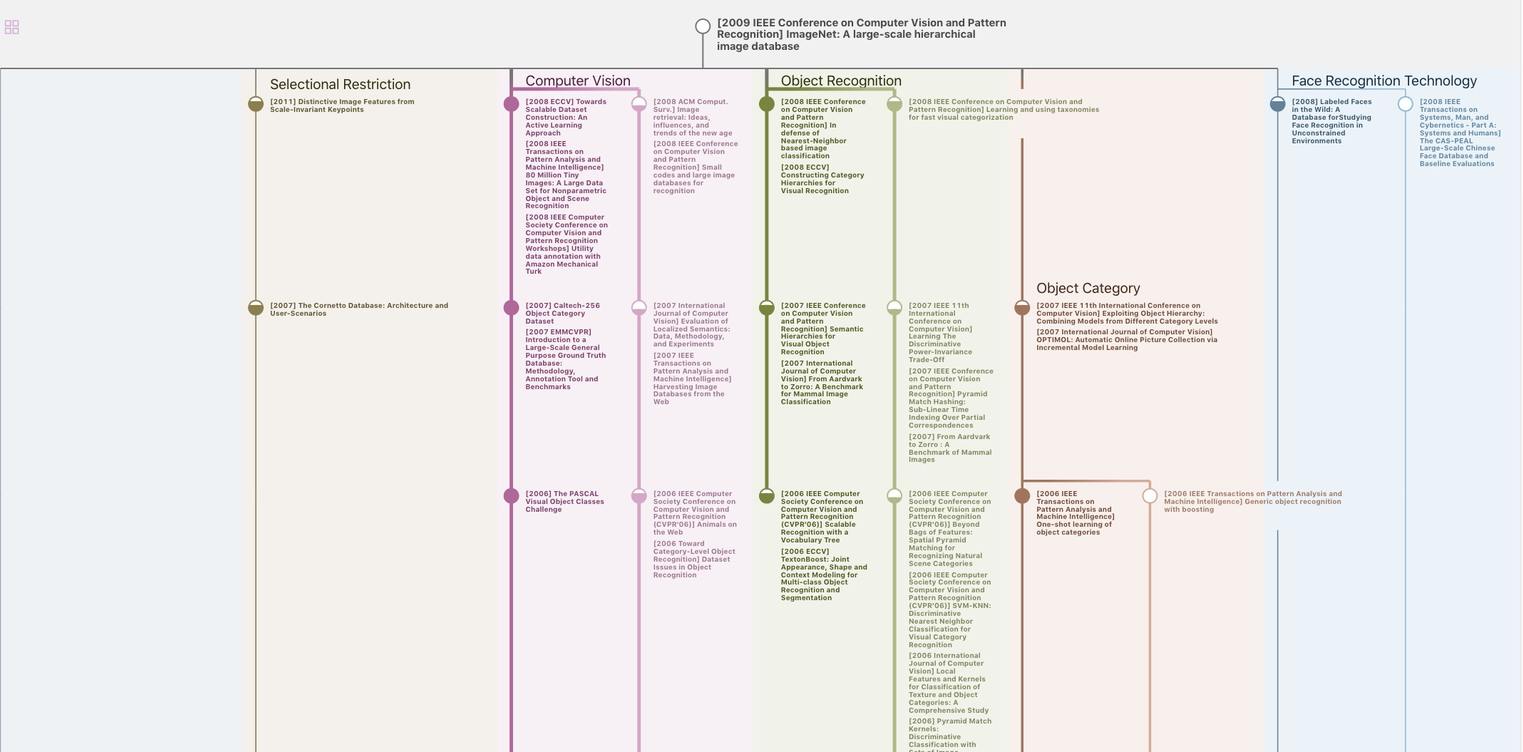
生成溯源树,研究论文发展脉络
Chat Paper
正在生成论文摘要