Fake News Detection Through Temporally Evolving User Interactions
Advances in Knowledge Discovery and Data Mining(2023)
摘要
Detecting fake news on social media is an increasingly important problem, because of the rapid dissemination and detrimental impact of fake news. Graph-based methods that encode news propagation paths into tree structures have been shown to be effective. Existing studies based on such methods represent the propagation of news through static graphs or coarse-grained graph snapshots. They do not capture the full dynamics of graph evolution and hence the temporal news propagation patterns. To address this issue and model dynamic news propagation at a finer-grained level, we propose a temporal graph-based model. We join this model with a neural Hawkes process model to exploit the distinctive self-exciting patterns of true news and fake news on social media. This creates a highly effective fake news detection model that we named SEAGEN. Experimental results on real datasets show that SEAGEN achieves an accuracy of fake news detection of over 93% with an advantage of over 2.5% compared to other state-of-the-art models.
更多查看译文
关键词
Fake News Detection, Dynamic Graph Embedding
AI 理解论文
溯源树
样例
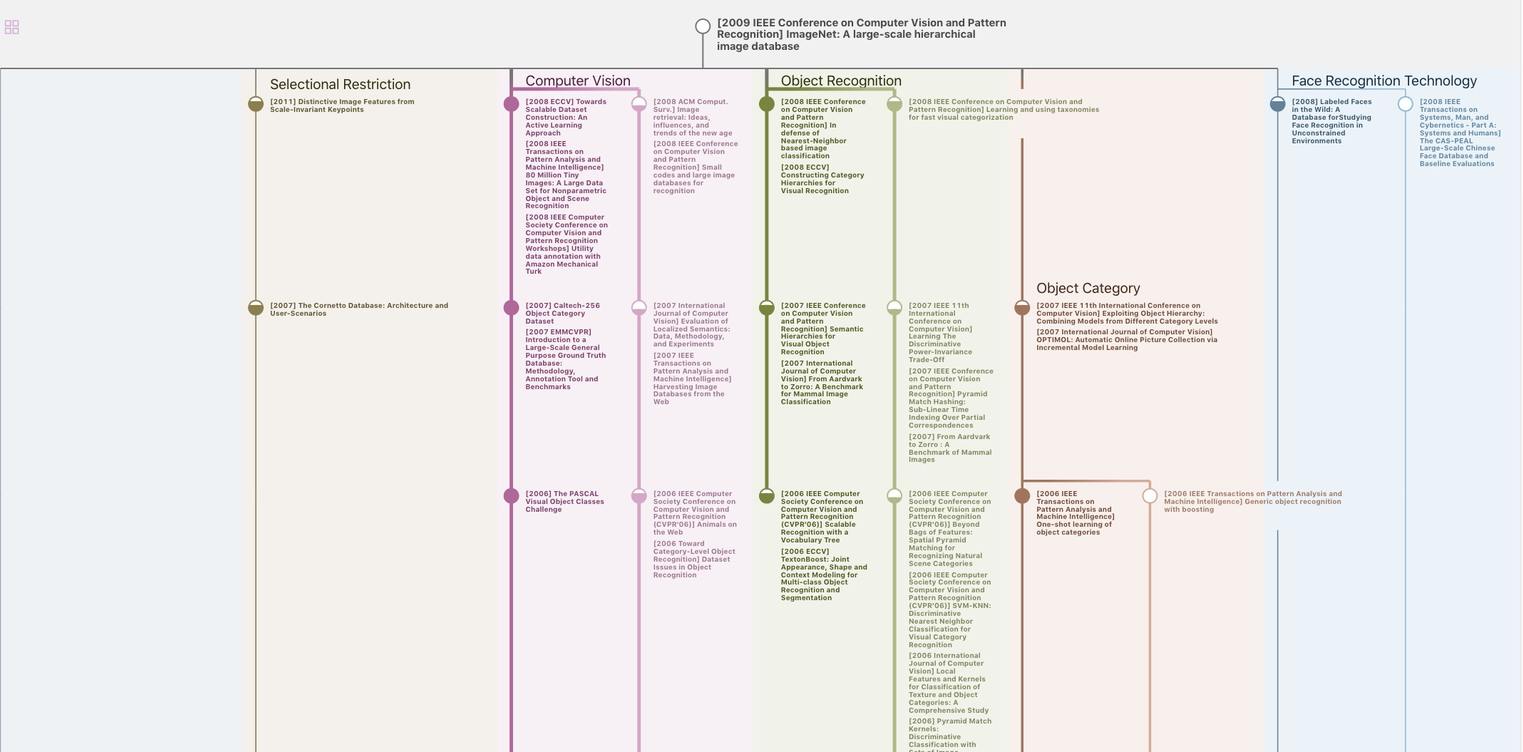
生成溯源树,研究论文发展脉络
Chat Paper
正在生成论文摘要