Learning by Small Loss Approach Multi-label to Deal with Noisy Labels
Computational Science and Its Applications – ICCSA 2023(2023)
摘要
Noisy data samples is a common problem for deep learning models applied to real-world applications. In this context, noisy samples refer to samples with incorrect labels, which can potentially degenerate the robustness of a model. Several works account for this issue in multi-class scenarios. However, despite a number of possible applications, multi-label noise remains an under-explored research field. In this work, two novel approaches to handle noise in this scenario are presented. First, we propose a new multi-label version of the Small Loss Approach (SLA), formerly multi-class, to handle multi-label noise. Second, we apply the multi-label SLA to a novel model, Learning by SLA Multi-label, based on Co-teaching. The proposed model achieves a performance gain of
$$15\%$$
in the benchmark UcMerced when compared to its baseline Co-teaching and a standard model (without any noise-handling technique). In addition, the model is also evaluated in a real-world scenario of underwater equipment imagery classification, yielding a relative improvement of
$$9\%$$
in F1-Score.
更多查看译文
关键词
learning,loss,multi-label
AI 理解论文
溯源树
样例
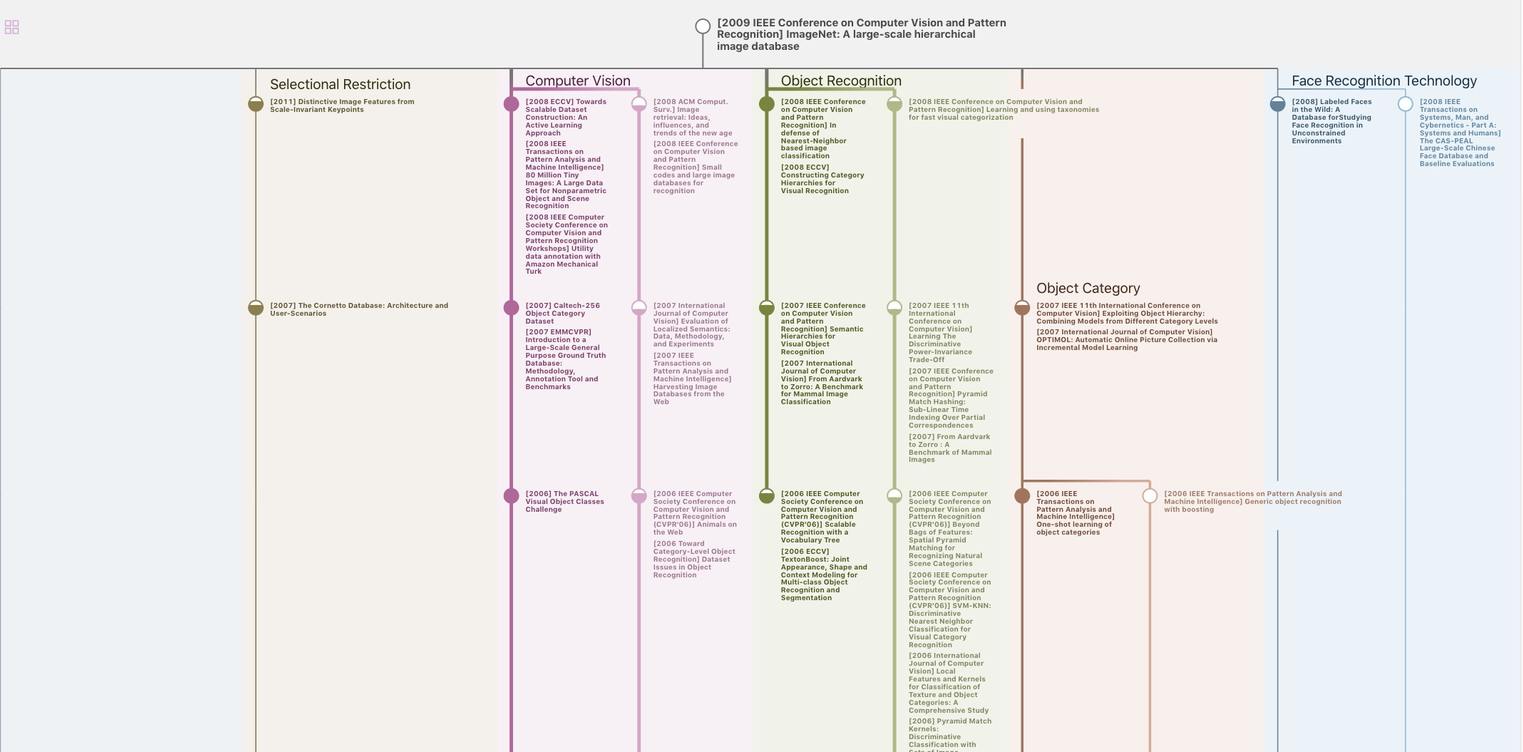
生成溯源树,研究论文发展脉络
Chat Paper
正在生成论文摘要