Using Large Language Models to Provide Formative Feedback in Intelligent Textbooks
Artificial Intelligence in Education. Posters and Late Breaking Results, Workshops and Tutorials, Industry and Innovation Tracks, Practitioners, Doctoral Consortium and Blue Sky(2023)
摘要
As intelligent textbooks become more ubiquitous in classrooms and educational settings, the need arises to automatically provide formative feedback to written responses provided by students in response to readings. This study develops models to automatically provide feedback to student summaries written at the end of intelligent textbook sections. The study builds on Botarleanu et al. (2022), who used the Longformer Large Language Model, a transformer Neural Network, to build a summary grading model. Their model explains around 55% of holistic summary score variance when compared to scores assigned by human raters on an analytic rubric. This study uses a principal component analysis to distill scores from the analytic rubric into two principal components – content and wording. When training the models on the summaries and the sources using these principal components, we explained 79% and 66% of the score variance for content and wording, respectively. The developed models are freely available on HuggingFace and will allow formative feedback to users of intelligent textbooks to assess reading comprehension through summarization in real-time. The models can also be used for other summarization applications in learning systems.
更多查看译文
关键词
intelligent textbooks, large language models, automated summary scoring, transformers
AI 理解论文
溯源树
样例
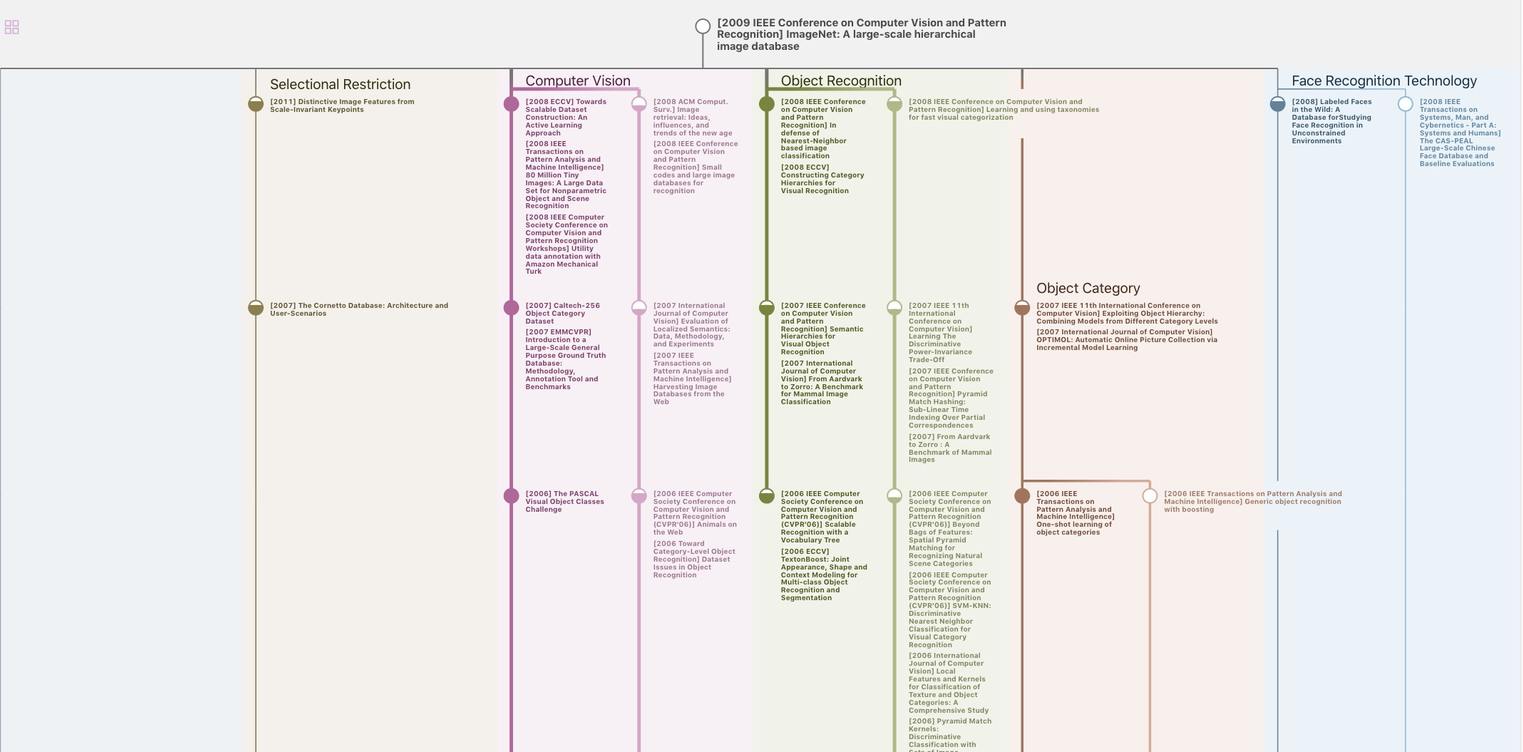
生成溯源树,研究论文发展脉络
Chat Paper
正在生成论文摘要