An Efficient Regional Co-location Pattern Mining Algorithm Over Extended Objects Based on Neighborhood Distribution Relation Computation
Big Data and Social Computing(2023)
摘要
Regional co-location patterns (RCPs) indicate the feature sets that generally co-occur within sub-regions but rarely co-occur in the whole study area. Spatial features have different densities and neighbor relationships in different regions, which poses great challenges to the two core steps of RCPs—regional partitioning and prevalence computation of RCPs in the sub-regions. Moreover, the existing RCPs mining methods are limited to point instances, while RCPs over extended spatial instances have not yet been explored. To address these challenges, this study develops a feature transaction method to detect RCPs and their prevalent regions. We first draw the radiation range of instances with distance buffers and generate feature transactions at buffer intersections between instances. Second, transactions are categorized into global prevalent and non-global prevalent. The locations of the non-global prevalent feature transactions are used to imply the range of candidate RCPs. In the local range, the distribution relation of the features is calculated to mine RCPs. We denote this process as neighborhood distribution relation computation. Experimental evaluations illustrate our proposed approach can capture more detailed RCPs information over extended instances and has better efficiency than the comparison algorithms.
更多查看译文
关键词
neighborhood,pattern,co-location
AI 理解论文
溯源树
样例
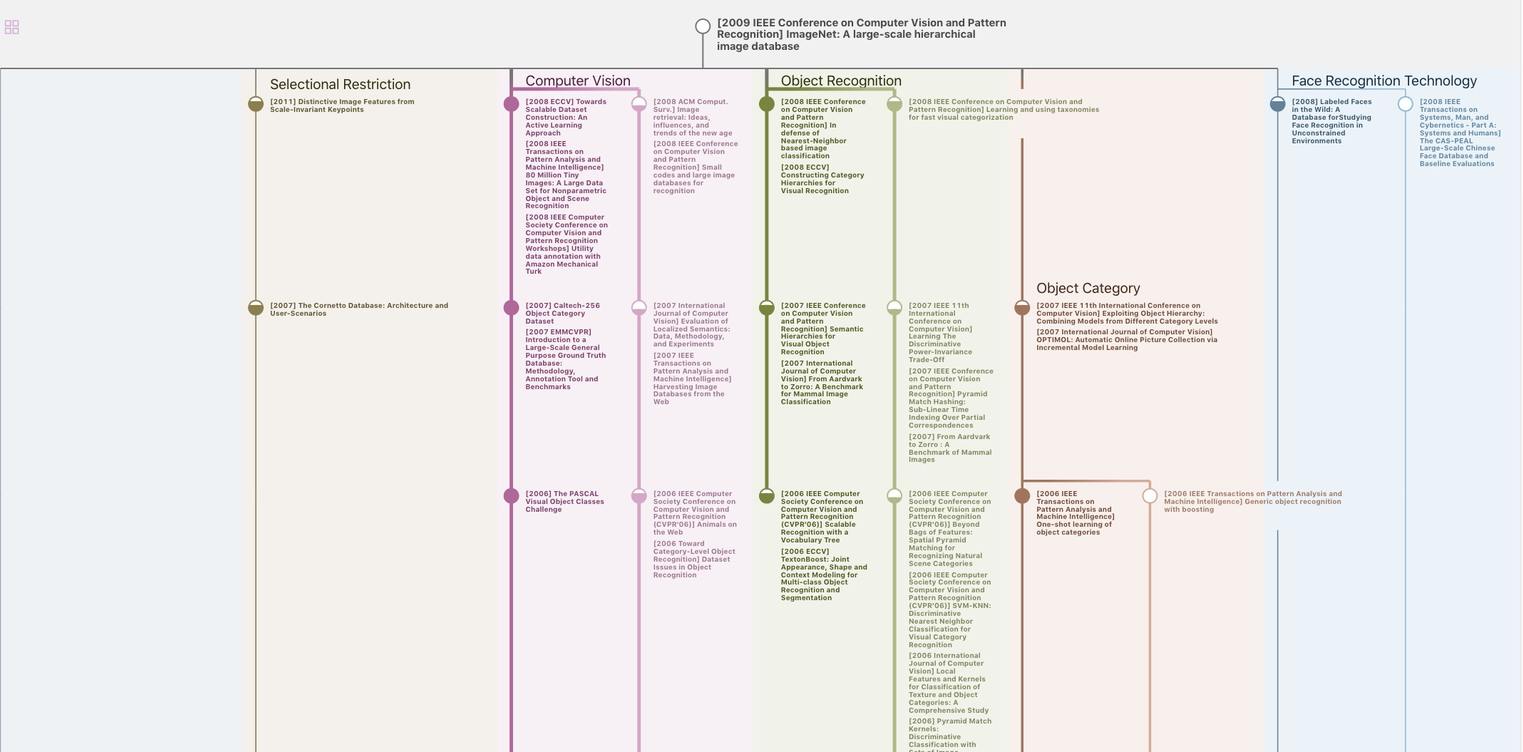
生成溯源树,研究论文发展脉络
Chat Paper
正在生成论文摘要