Contextualized Embeddings from Transformers for Sentiment Analysis on Code-Mixed Hinglish Data: An Expanded Approach with Explainable Artificial Intelligence
Speech and Language Technologies for Low-Resource Languages (2023)
摘要
Transformer-based models have gained traction for giving breakthrough performance on various Natural Language Processing (NLP) tasks in recent years. A number of studies have been conducted to understand the type of information learned by the model and its performance on different tasks. YouTube comments can serve as a rich source for multilingual data, which can be used to train state-of-the-art models. In this study, two transformer-based models, multilingual Bidirectional Encoder Representations from Transformers (mBERT) and RoBERTa, are fine-tuned and evaluated on code-mixed ‘Hinglish’ data. The representations learned by the intermediate layers of the models are also studied by using them as features for machine learning classifiers. The results show a significant improvement compared to the baseline for both datasets using the feature-based method, with the highest accuracy of 92.73% for Kabita Kitchen’s channel and 87.42% for Nisha Madhulika’s channel. Explanations of the model predictions using the Local Interpretable Model-Agnostic Explanations (LIME) technique show that the model is using significant features for classification and can be trusted.
更多查看译文
关键词
Bidirectional encoder representations from transformers, Natural Language Processing, Sentiment Analysis, Cookery Channels, Bertology, Transformers, Hinglish, Explainable Artificial Intelligence, Local Interpretable Model-Agnostic Explanations
AI 理解论文
溯源树
样例
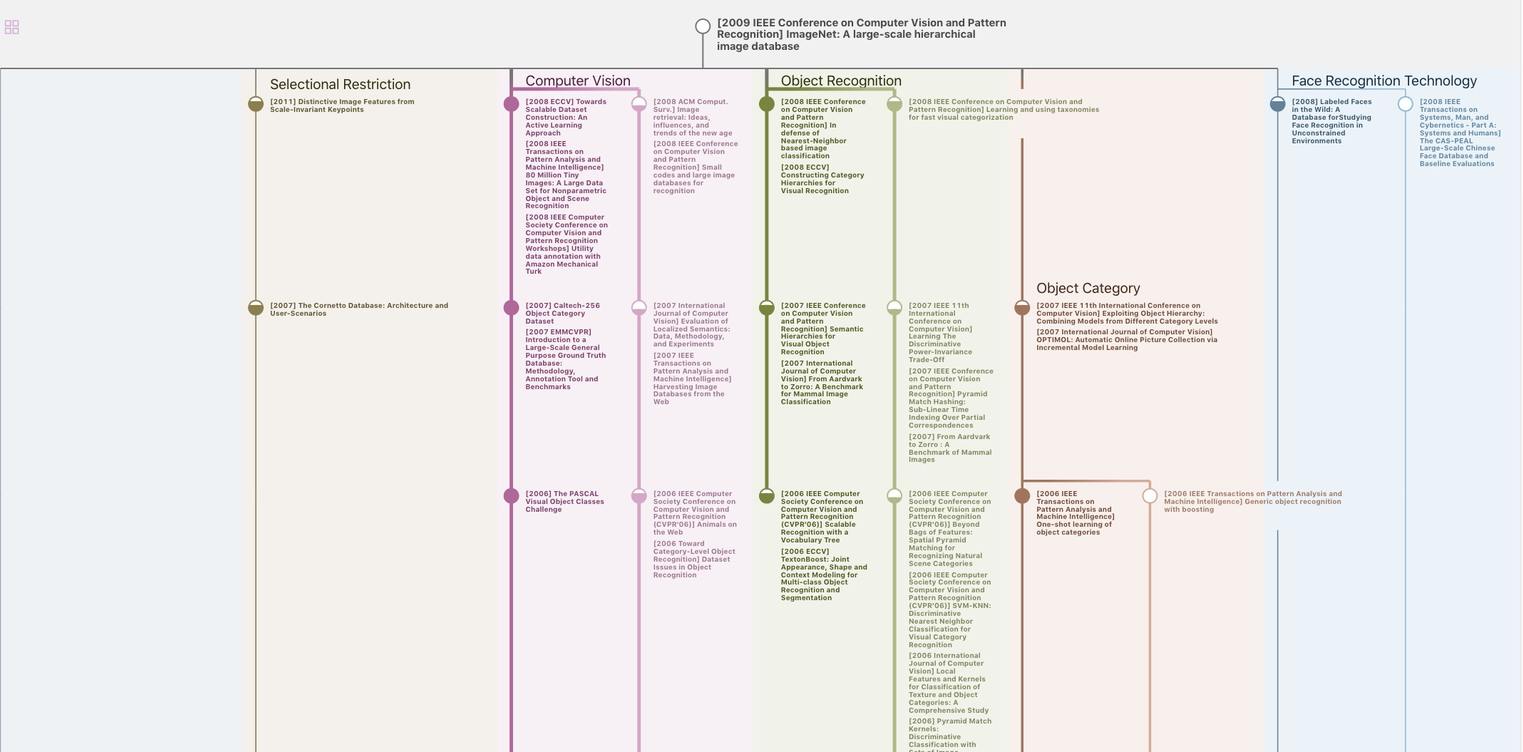
生成溯源树,研究论文发展脉络
Chat Paper
正在生成论文摘要