Fundamental Frequency Removal PCA Method and SVM Approach Used for Structure Feature Distilling and Damage Diagnosis
Communications, Networking, and Information Systems(2023)
摘要
In Structure Nondestructive Testing (SNT), it’s easy to obtain abundant vibration frequency features by advanced sensors and signal collectors. But too many features often lead to algorithms termination unexpectedly because of computer memory overflow, or trap in local optimum because of sparse nature of solutions in high dimension space. Classical Principal Component Analysis (PCA) algorithm can sort features by descending order but cannot directly select features used for classification. Many “principal components” choose by PCA usually lead to wrong decision because they are not always “principal features” which can separate samples among different classes rightly. Same or similar structures will have same or similar frequency and amplitude correspondingly, they were called “fundamental frequencies”. This paper proposed a new feature distilling method named “Fundamental Frequency Removal Principal Component Analysis (FFR-PCA)”, fundamental frequencies were removed so as to reduced features size substantially and improved calculation speed and accuracy successfully. Support Vector Machine (SVM) was used in pattern recognition experiments and showed its superior performance on engineering structure damage diagnosis. The proposed method in this paper is scalable and can be extended to an entire bridge engineering structure faults diagnosis.
更多查看译文
关键词
Structure Nondestructive Testing, Fundamental Frequency Removal Principal Component Analysis (FFR-PCA), PROE Modeling, ADAMS Simulation, Support Vector Machine (SVM)
AI 理解论文
溯源树
样例
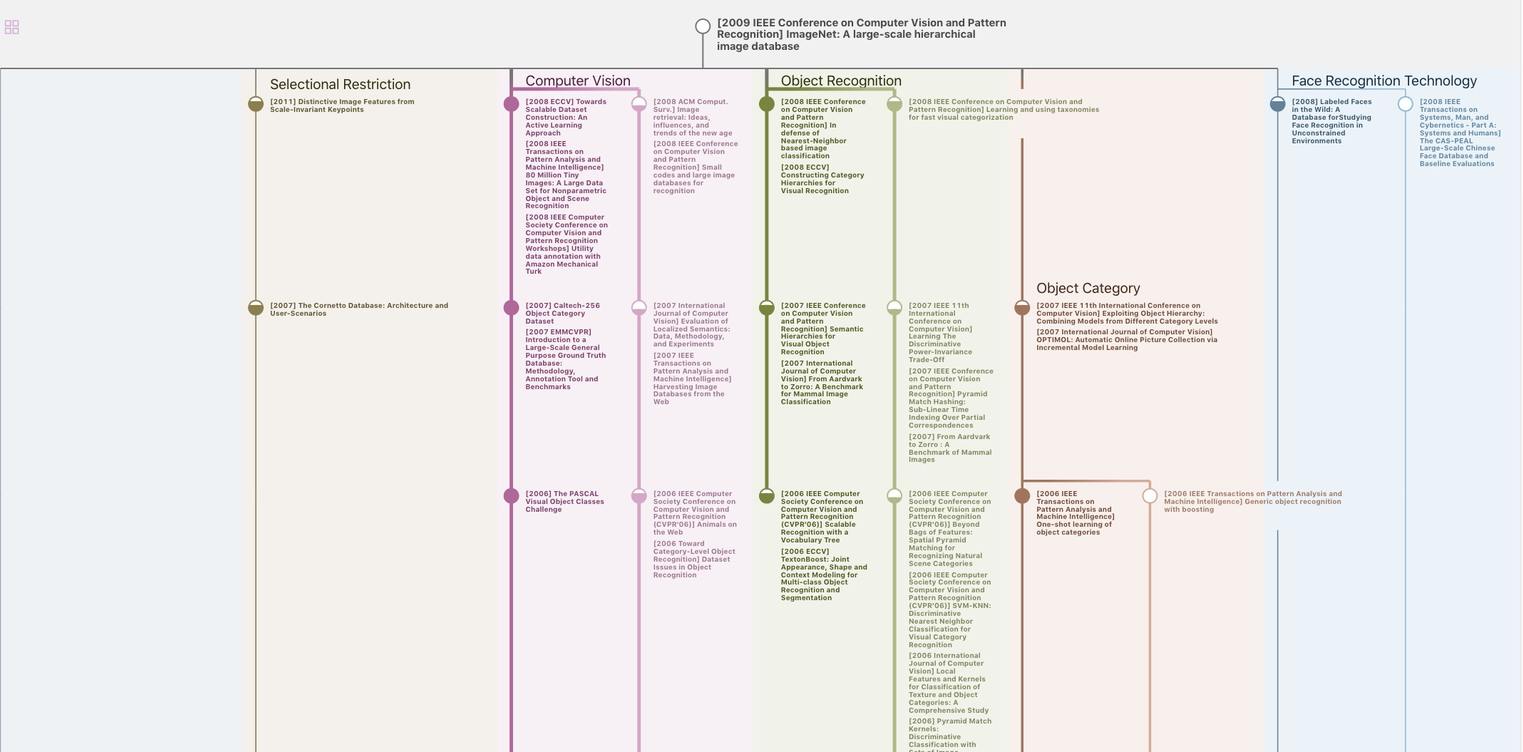
生成溯源树,研究论文发展脉络
Chat Paper
正在生成论文摘要