ECSSD: Hardware/Data Layout Co-Designed In-Storage-Computing Architecture for Extreme Classification
ISCA(2023)
摘要
With the rapid growth of classification scale in deep learning systems, the final classification layer becomes extreme classification with a memory footprint exceeding the main memory capacity of the CPU or GPU. The emerging in-storage-computing technique offers an opportunity on account of the fact that SSD has enough storage capacity for the parameters of extreme classification. However, the limited performance of naive in-storage-computing schemes is insufficient to support the heavy workload of extreme classification. To this end, we propose ECSSD, the first hardware/data layout co-designed in-storage-computing architecture for extreme classification, based on the approximate screening algorithm. We propose an alignment-free floating-point MAC circuit technique to improve the computational ability under the limited area budget of in-storage-computing schemes so that the computational ability can match SSD's high internal bandwidth. We present a heterogeneous data layout design for the 4/32-bit weight data in the approximate screening algorithm to avoid data transfer interference and further utilize the internal DRAM bandwidth of SSD. Moreover, we propose a learning-based adaptive interleaving framework to balance the access workload in each flash channel and improve channel-level bandwidth utilization. Putting them together, our ECSSD achieves 3.24-49.87x performance improvements compared with state-of-the-art baselines.
更多查看译文
关键词
In-storage-computing architecture,Extreme classification
AI 理解论文
溯源树
样例
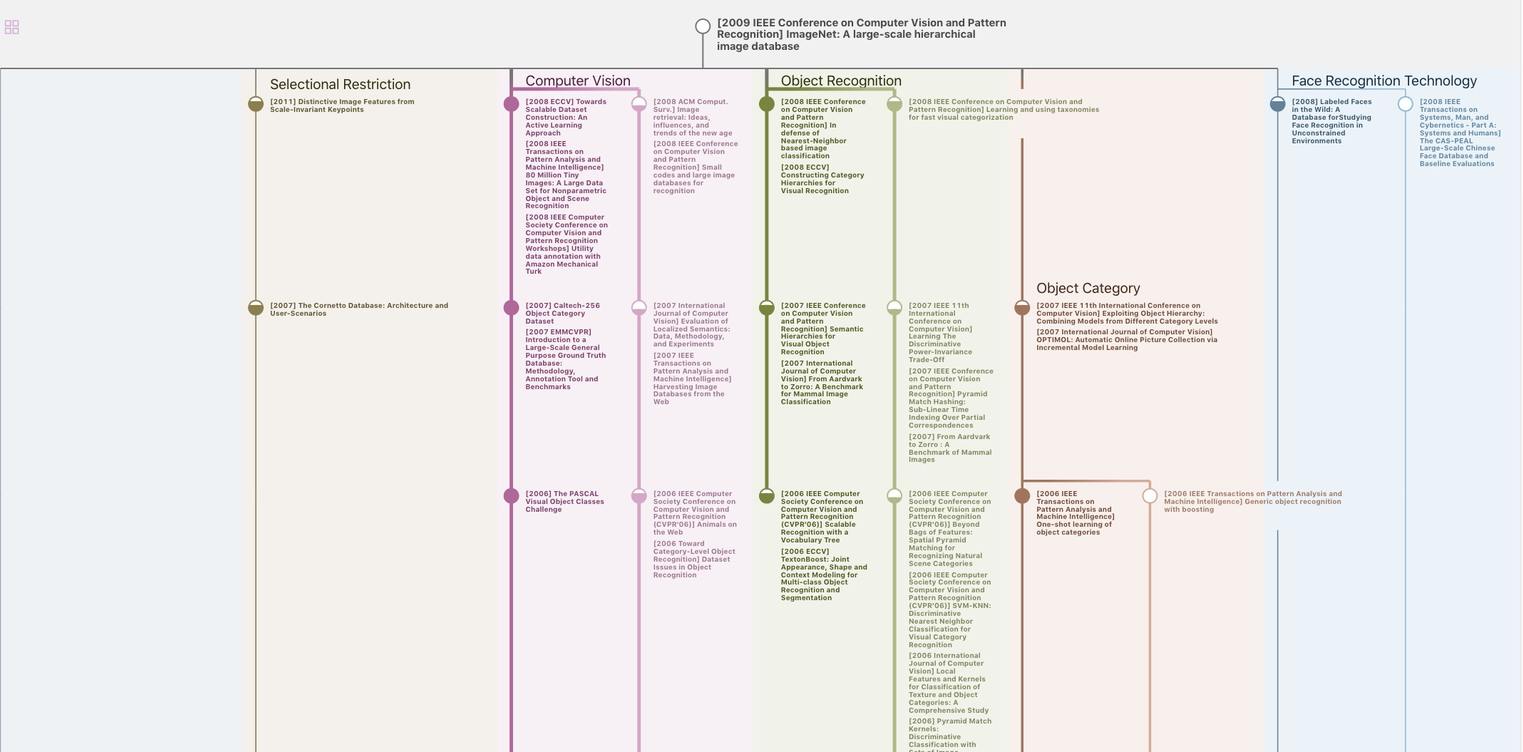
生成溯源树,研究论文发展脉络
Chat Paper
正在生成论文摘要