Reward Shaping from Hybrid Systems Models in Reinforcement Learning.
NFM(2023)
摘要
Reinforcement learning is increasingly often used as a learning technique to implement control tasks in autonomous systems. To meet stringent safety requirements, formal methods for learning-enabled systems, such as closed-loop neural network verification, shielding, falsification, and online reachability analysis, analyze learned controllers for safety violations. Besides filtering unsafe actions during training, these approaches view verification and training largely as separate tasks. We propose an approach based on logically constrained reinforcement learning to couple formal methods and reinforcement learning more tightly by generating safety-oriented aspects of reward functions from verified hybrid systems models. We demonstrate the approach on a standard reinforcement learning environment for longitudinal vehicle control.
更多查看译文
关键词
hybrid systems models,reinforcement,shaping
AI 理解论文
溯源树
样例
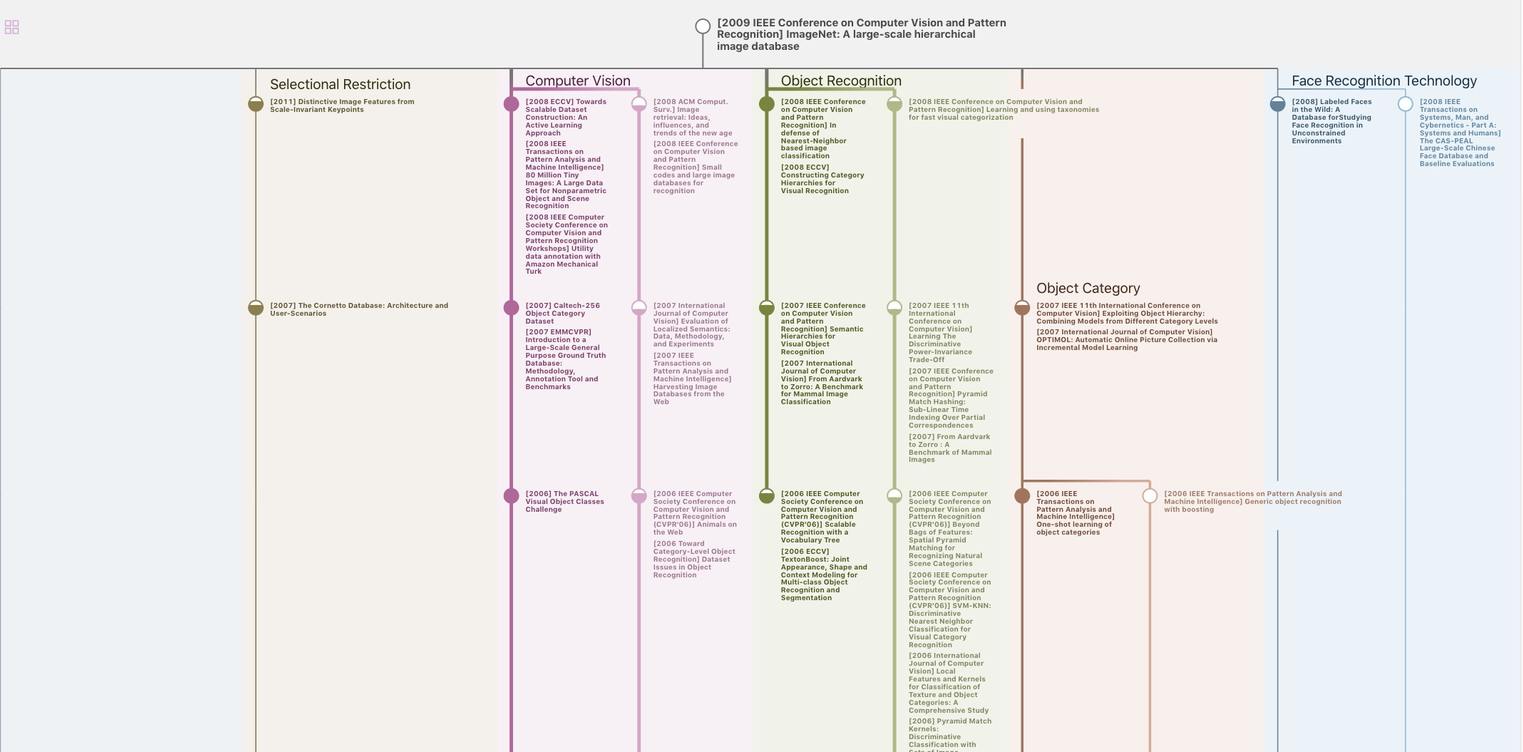
生成溯源树,研究论文发展脉络
Chat Paper
正在生成论文摘要