Comparative Analysis of Membership Inference Attacks in Federated Learning.
IDEAS(2023)
摘要
Given a federated learning model and a record, a membership inference attack can determine whether this record is part of the model’s training dataset. Federated learning is a machine learning technique that enables different parties to train a model without the need to centralize or share that data. Membership inference attack risks the private datasets if those datasets are used to train the federated learning model and access to the generated model is available. There is a need for further study in a federated learning environment to develop effective countermeasures against the membership inference attack without compromising the utility of the target model. In this study, we empirically investigated and compared various membership inference attack approaches in a federated learning environment. We also evaluated these attacks on several optimizers and analyzed them with and without countermeasures.
更多查看译文
AI 理解论文
溯源树
样例
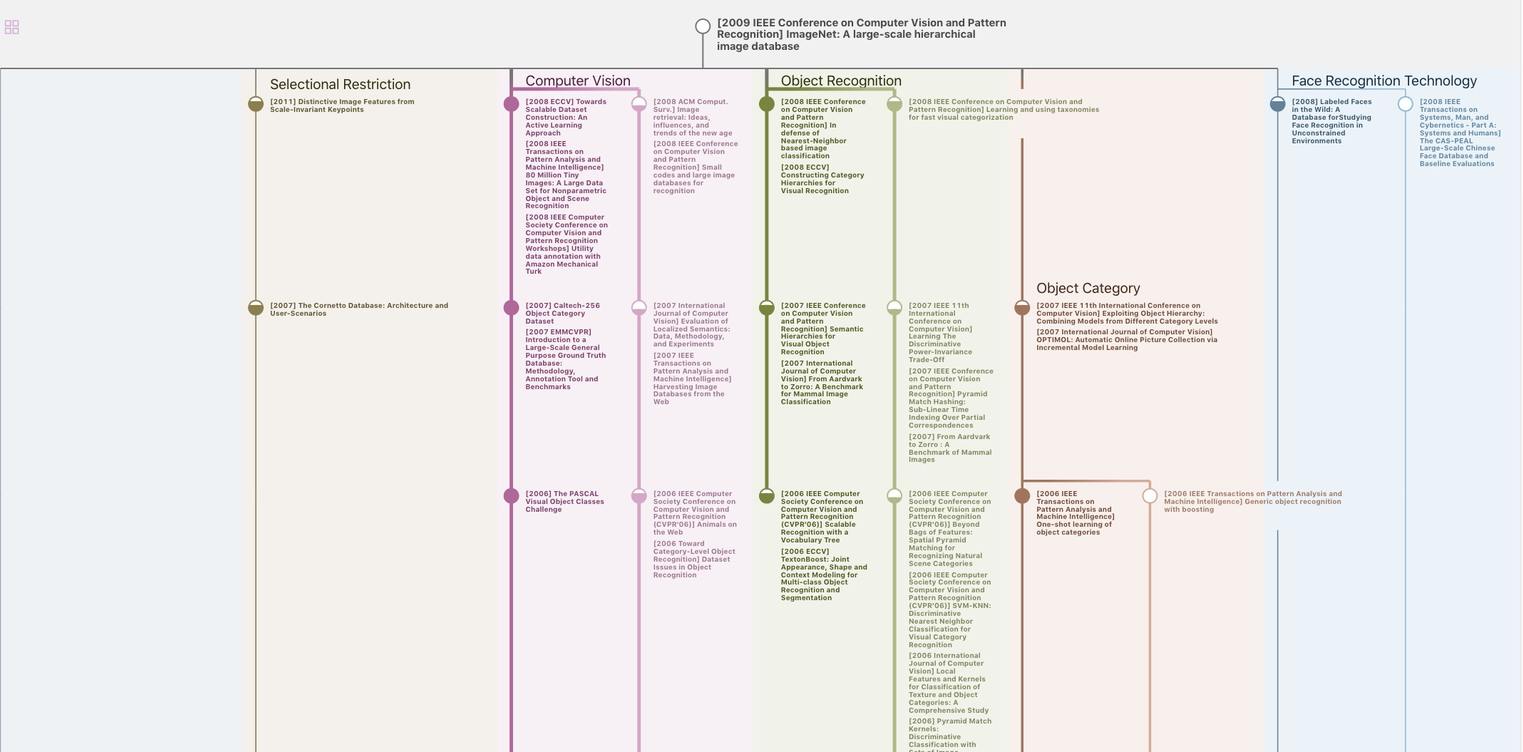
生成溯源树,研究论文发展脉络
Chat Paper
正在生成论文摘要