A method combining improved Mahalanobis distance and adversarial autoencoder to detect abnormal network traffic.
IDEAS(2023)
摘要
[]The Internet has been widely used in various industries, so the anomaly detection of network traffic is of great significance for the security of network applications. Currently, network traffic anomaly detection has a high detection accuracy, but it relies on supervised learning techniques, which have issues with label identification difficulties and limited scalability. To solve the above-mentioned problems, a method combining improved Mahalanobis distance and autoencoder (AE) to detect abnormal network traffic is proposed. To increase detection effectiveness, the approach is trained without using the labels and makes use of an enhanced inverse of the Mahalanobis distance and a threshold to easily differentiate the partly normal data. In this model, the AE and the generative adversarial network (GNN) are fused, and the output of the AE is fed to the discriminator for discrimination. The loss is constructed based on the output of AE and discriminator, which improves the feature extraction ability of the autoencoder, and is more conducive to distinguishing potential anomalies. Experiments show that the proposed method has an anomaly detection precision rate of 96% and 95% F1 value on the CICIDS2017 dataset and an anomaly detection precision rate of 90% on the cicids2018 dataset. This effectively demonstrates the suggested method’s ability to generalize and have strong network traffic anomaly detection.
更多查看译文
AI 理解论文
溯源树
样例
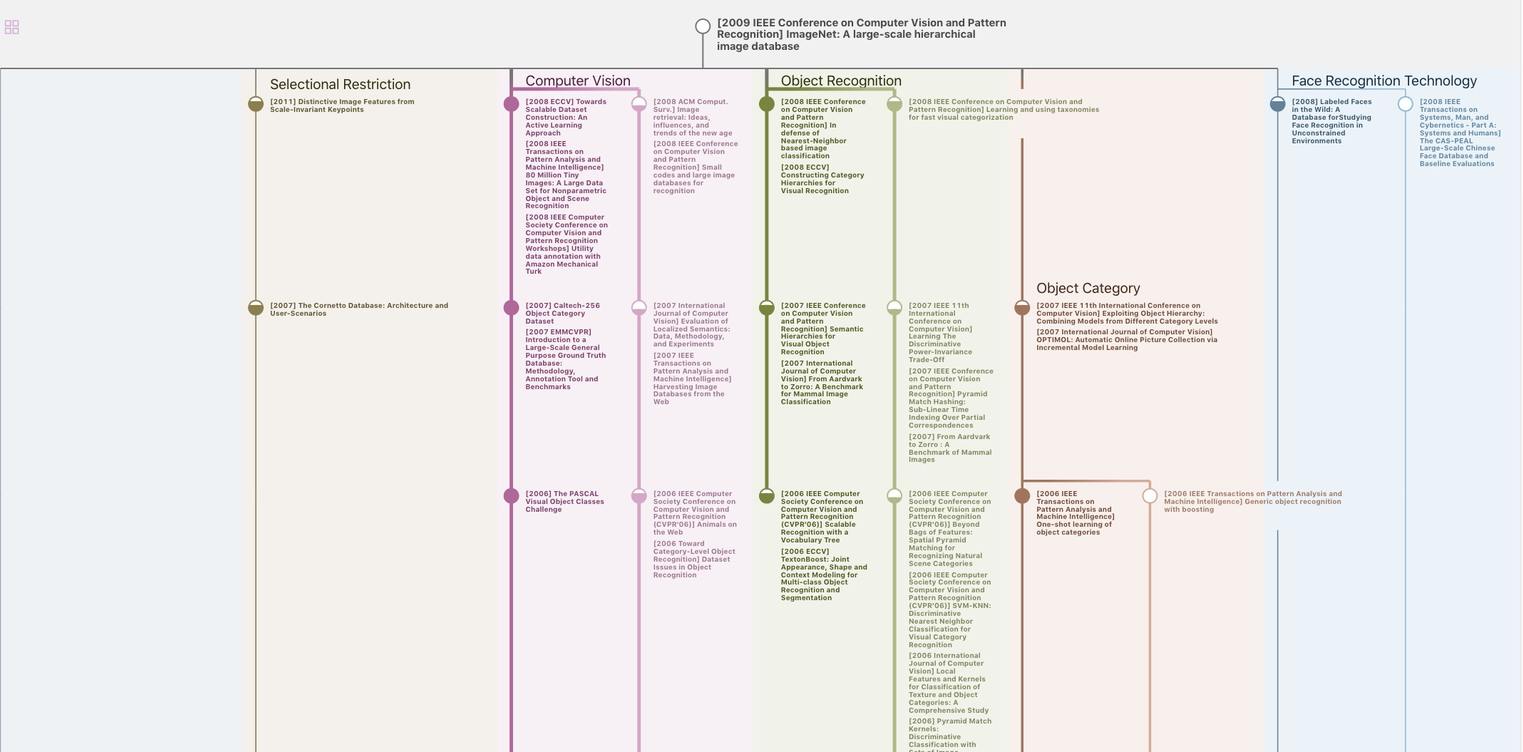
生成溯源树,研究论文发展脉络
Chat Paper
正在生成论文摘要