Epicardial Adipose Tissue Segmentation and Quantification Based on Transformer Model.
BIC(2023)
摘要
Epicardial adipose tissue (EAT) is an emerging marker and potential therapeutic target of cardiovascular diseases, and it is necessary to segment and quantify EAT. Clinical quantification of EAT requires experts to perform voxel-level labeling, which is time-consuming, laborious, and highly subjective. Therefore, an automatic method that can accurately segment and quantify the EAT are urgently needed clinically. The currently developed EAT segmentation and quantification methods are mainly based on convolutional neural network (CNN), but CNN cannot model global dependencies. However, EAT wraps the heart with a wide range of distribution in the computed tomography images, and the global information needs to be modeled to achieve the accurate segmentation. This study applied a Transformer-based model that can effectively model global dependencies, and for the first time evaluated the ability of the classical Swin UNETR in EAT. For EAT segmentation, the Dice correlation coefficient, Hausdorff distance, and surface distance were 0.839, 24.74, and 0.584. For EAT quantification, Swin UNETR model had high consistency (concordance correlation coefficient of 0.836) and correlation (Pearson correlation coefficient of 0.969) with expert. The average volume difference and mean absolute error were -21.73 cm³ and 21.77 cm³, which suggested that Transformer-based model had similar ability with expert to segment and quantify EAT. With Transformer-based model, it is expected to further improve the performance of EAT segmentation and quantification. These methods can assist clinical EAT segmentation and quantification, and had the potential to be applied in the clinical practice.
更多查看译文
AI 理解论文
溯源树
样例
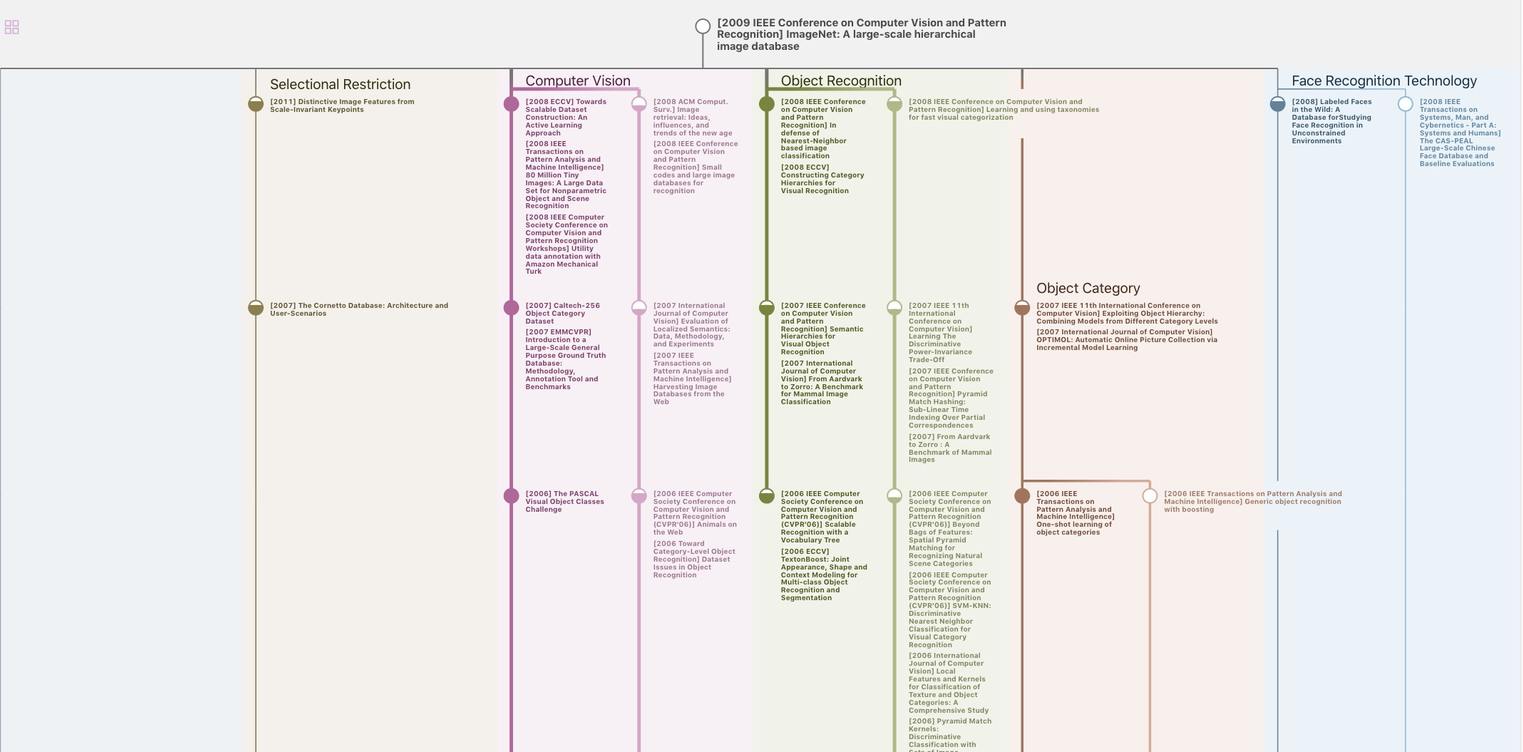
生成溯源树,研究论文发展脉络
Chat Paper
正在生成论文摘要