Deep Physics-Informed Super-Resolution of Cardiac 4D-Flow MRI
INFORMATION PROCESSING IN MEDICAL IMAGING, IPMI 2023(2023)
摘要
4D-flow magnetic resonance imaging (MRI) provides noninvasive blood flow reconstructions in the heart. However, low spatio-temporal resolution and significant noise artefacts hamper the accuracy of derived haemodynamic quantities. We propose a physics-informed super-resolution approach to address these shortcomings and uncover hidden solution fields. We demonstrate the feasibility of the model through two synthetic studies generated using computational fluid dynamics. The Navier-Stokes equations and no-slip boundary condition on the endocardium are weakly enforced, regularising model predictions to accommodate network training without high-resolution labels. We show robustness to each type of data degradation, achieving normalised velocity RMSE values of under 16% at extreme spatial and temporal upsampling rates of 16x and 10x respectively, using a signal-to-noise ratio of 7.
更多查看译文
关键词
Physics-informed machine learning,4D-flow MRI,Super-resolution
AI 理解论文
溯源树
样例
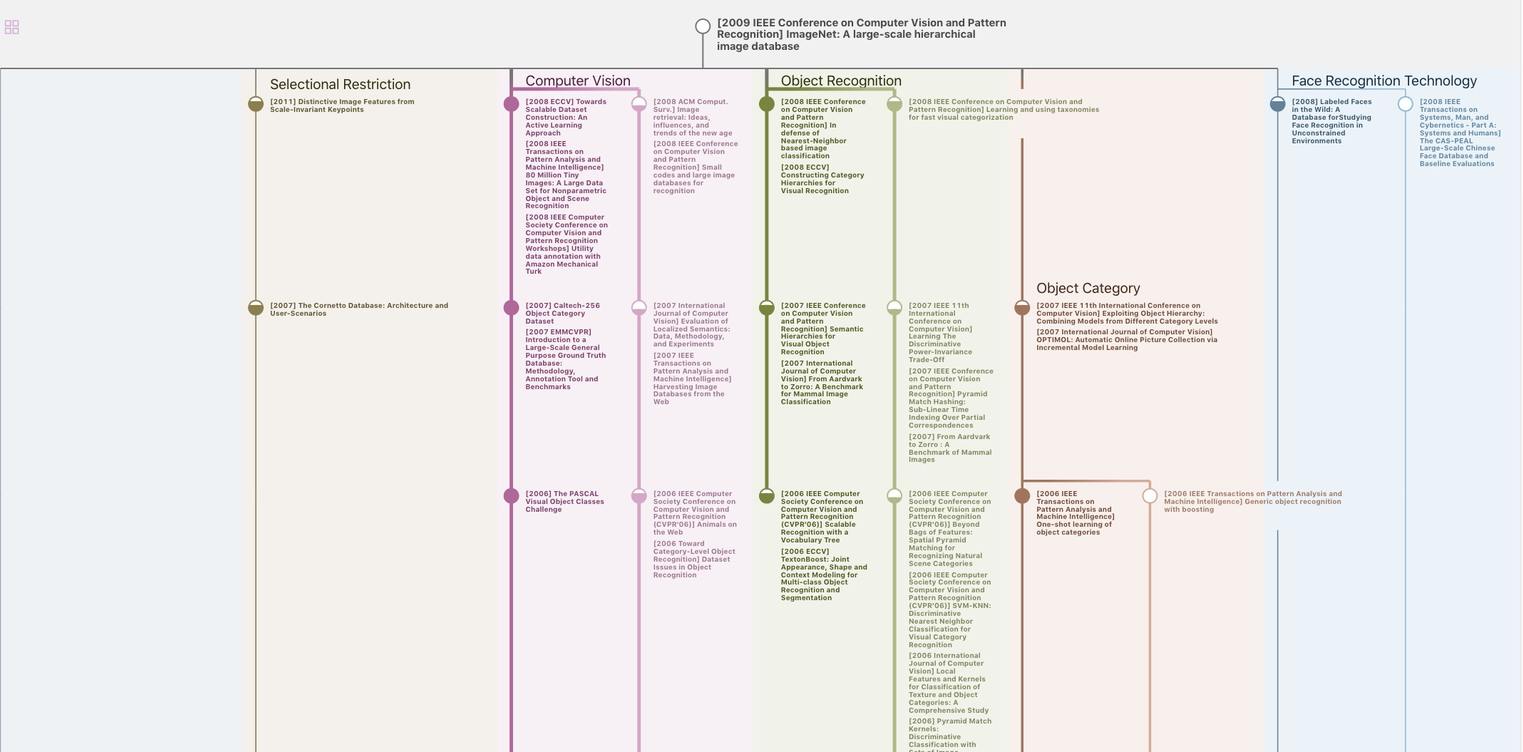
生成溯源树,研究论文发展脉络
Chat Paper
正在生成论文摘要