Scalable Orthonormal Projective NMF via Diversified Stochastic Optimization
INFORMATION PROCESSING IN MEDICAL IMAGING, IPMI 2023(2023)
摘要
The increasing availability of large-scale neuroimaging initiatives opens exciting opportunities for discovery science of human brain structure and function. Data-driven techniques, such as Orthonormal Projective Non-negative Matrix Factorization (opNMF), are well positioned to explore multivariate relationships in big data towards uncovering brain organization. opNMF enjoys advantageous interpretability and reproducibility compared to commonly used matrix factorization methods like Principal Component Analysis (PCA) and Independent Component Analysis (ICA), which led to its wide adoption in clinical computational neuroscience. However, applying opNMF in large-scale cohort studies is hindered by its limited scalability caused by its accompanying computational complexity. In this work, we address the computational challenges of opNMF using a stochastic optimization approach that learns over mini-batches of the data. Additionally, we diversify the stochastic batches via repulsive point processes, which reduce redundancy in the mini-batches and in turn lead to lower variance in the updates. We validated our framework on gray matter tissue density maps estimated from 1000 subjects part of the Open Access Series of Imaging (OASIS) dataset. We demonstrated that operations over mini-batches of data yield significant reduction in computational cost. Importantly, we showed that our novel optimization does not compromise the accuracy or interpretability of factors when compared to standard opNMF. The proposed model enables new investigations of brain structure using big neuroimaging data that could improve our understanding of brain structure in health and disease.
更多查看译文
关键词
NMF,Stochastic optimization,MRI,Big data
AI 理解论文
溯源树
样例
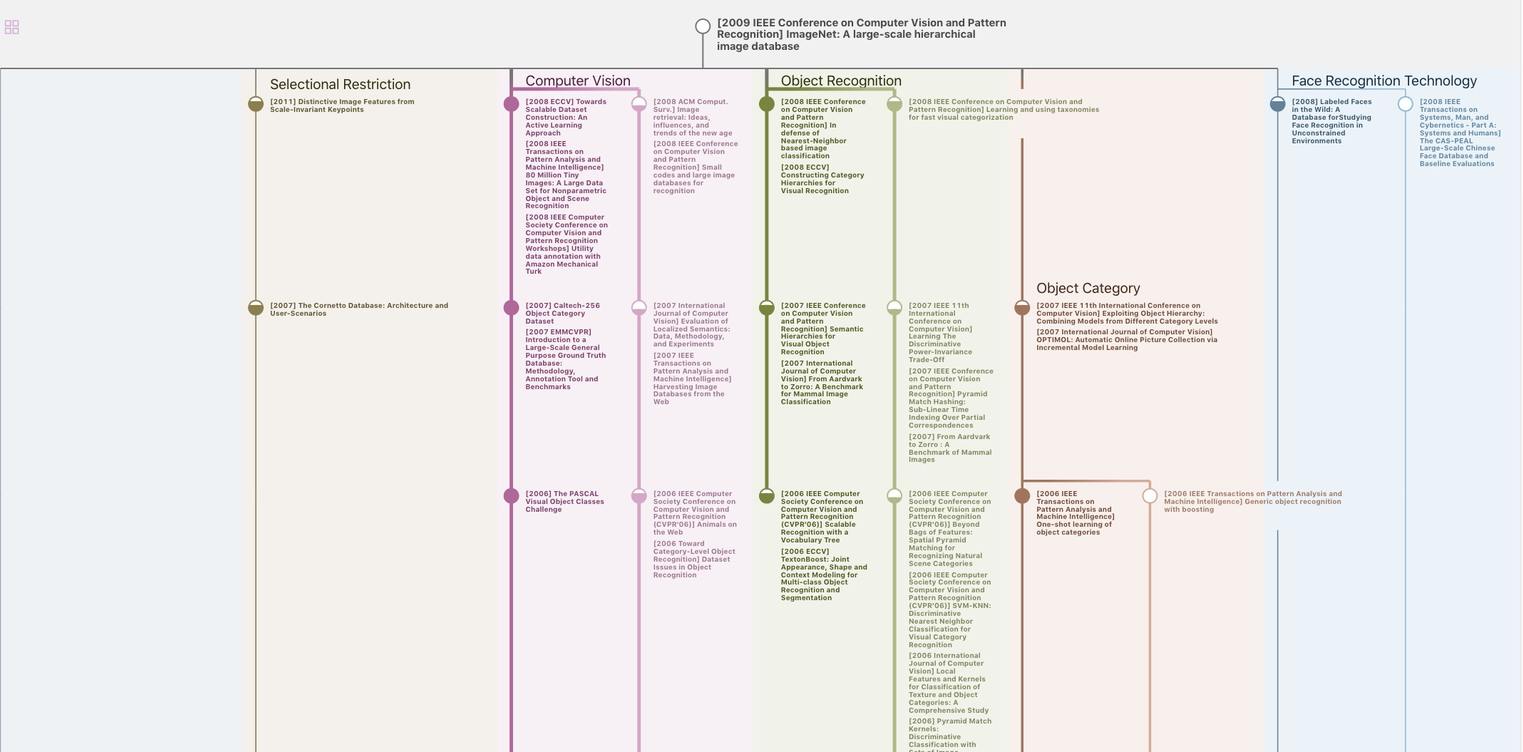
生成溯源树,研究论文发展脉络
Chat Paper
正在生成论文摘要