Informed Deep Learning for Anomaly Detection in Cyber-Physical Systems.
ICIT(2023)
摘要
Cyber-physical systems (CPS) are key components of modern infrastructure, and detecting anomalies is a critical role in assuring the safety and reliability of these cyber-physical systems. Deep neural networks (DNNs) have demonstrated excellent performance in a variety of domains due to their capacity to discover and identify patterns in high-dimensional data. However, their effectiveness is limited in the case of anomaly detection for cyber-physical systems where data collection is difficult, it is not always possible to obtain data for anomalous states, and people do not trust AI in securing critical infrastructure components. To address these limitations, prior knowledge can be incorporated into the modeling process to help fill in missing data and offer an explainable interface to the neural network's inner workings. In this paper, we will state the benefits and drawbacks of informed deep learning, present possible data sources and integration options, and provide examples from the literature where informed learning outperforms traditional data-driven methods in anomaly detection for cyber-physical systems. We hope that this evaluation will attract interest in informed learning and encourage the use of prior knowledge to improve purely data-driven approaches for anomaly detection in cyber-physical systems.
更多查看译文
关键词
Deep Learning,Informed Learning,Anomaly Detection,Cyber-Physical Systems
AI 理解论文
溯源树
样例
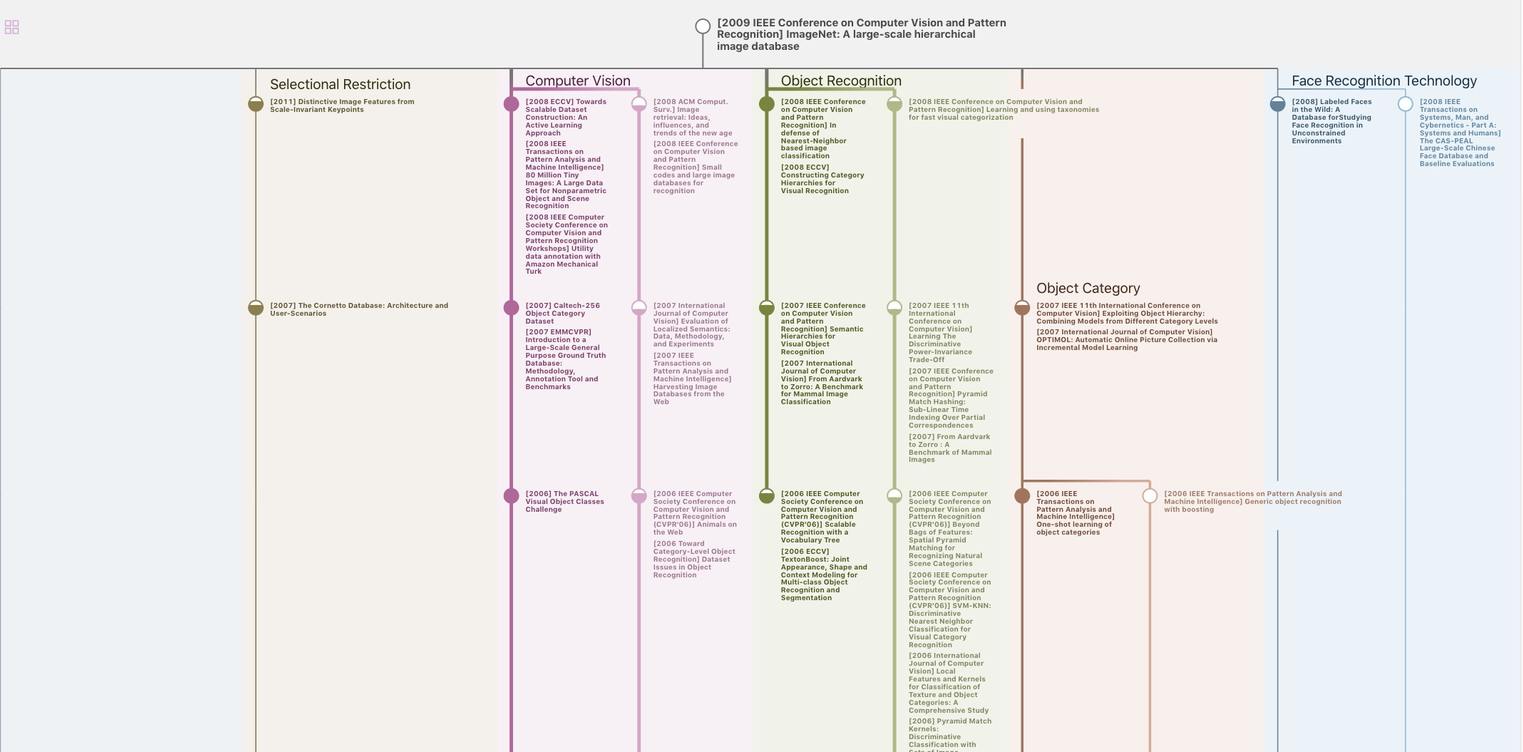
生成溯源树,研究论文发展脉络
Chat Paper
正在生成论文摘要