A Difference Measuring Network for Few-Shot Learning.
AIAI (2)(2023)
摘要
Few-Shot Learning (FSL) aims to distinguish novel categories for which only a few labeled samples are accessible. Due to significant advances in metric learning over the past decade, several metric-based approaches have been investigated for FSL to classify unseen categories with the k-nearest neighbor strategy. However, the existing metric-based FSL methods are typically based on the instance-level or class-level representations that might suffer from the adaptation problem because of the gap between seen and unseen classes. A fact is that human beings can quickly acquire new concepts by comparing similarities and differences between instances. Inspired by this fact, we explore a novel idea that learns difference-level representations instead of instance-level representations. This paper proposes a Difference Measuring Network (DMNet) that learns the patterns of difference from input paires of query-support. The DMNet converts both seen and unseen categories into a binary space that only contains the Same and the Different categories. Therefore, it concentrates on mining the patterns of differences between any two samples rather than the patterns of an individual instance. Finally, the DMNet distinguish whether a pair of inputs belongs to the same category or not. Experiments on five benchmark datasets demonstrate that the proposed DMNet is conducive to generalization ability of FSL.
更多查看译文
关键词
difference measuring network,learning,few-shot
AI 理解论文
溯源树
样例
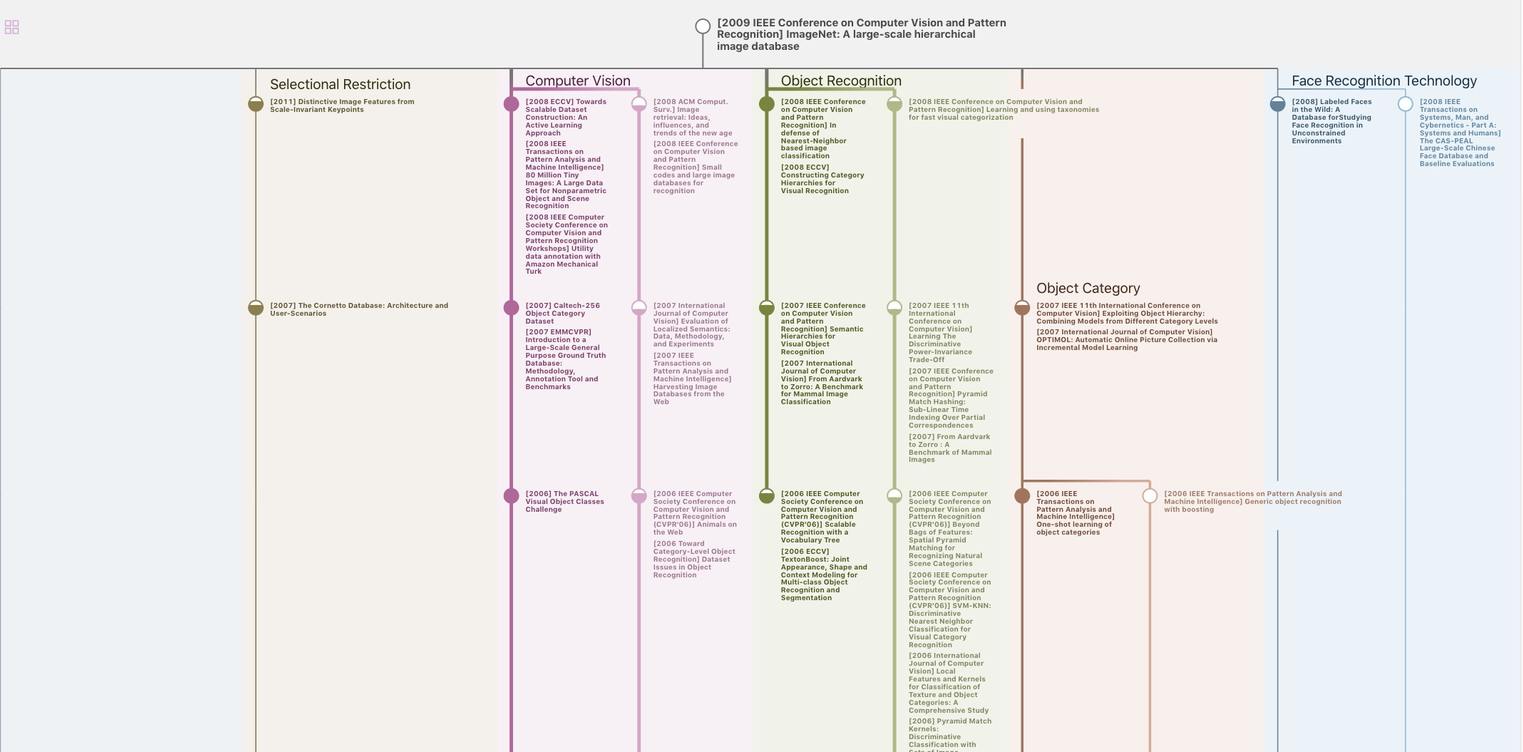
生成溯源树,研究论文发展脉络
Chat Paper
正在生成论文摘要