Knowledge-Enhanced Hierarchical Transformers for Emotion-Cause Pair Extraction.
PAKDD (4)(2023)
摘要
Emotion-cause pair extraction (ECPE) aims to extract all potential pairs of emotions and corresponding cause(s) from a given document. Current methods have focused on extracting possible emotion-cause pairs by directly analyzing the given documents on the basis of a large training set. However, there are many hard-matching emotion-cause pairs that require commonsense knowledge to understand. Exploiting only the given documents is insufficient to capture the latent semantics behind these hard-matching emotion-cause pairs, which may downgrade the performance of existing ECPE methods. To fill this gap, we propose a Knowledge-Enhanced Hierarchical Transformers framework for the ECPE task. Specifically, we first inject commonsense knowledge into the given documents to construct the knowledge-enhanced clauses. To incorporate the injected knowledge into the clause representations, we then develop a hierarchical Transformers module that leverages two different types of transformer blocks to encode knowledge-enriched clause representations at both global and local stages. Experimental results show that our method achieves state-of-the-art performance.
更多查看译文
关键词
hierarchical transformers,knowledge-enhanced,emotion-cause
AI 理解论文
溯源树
样例
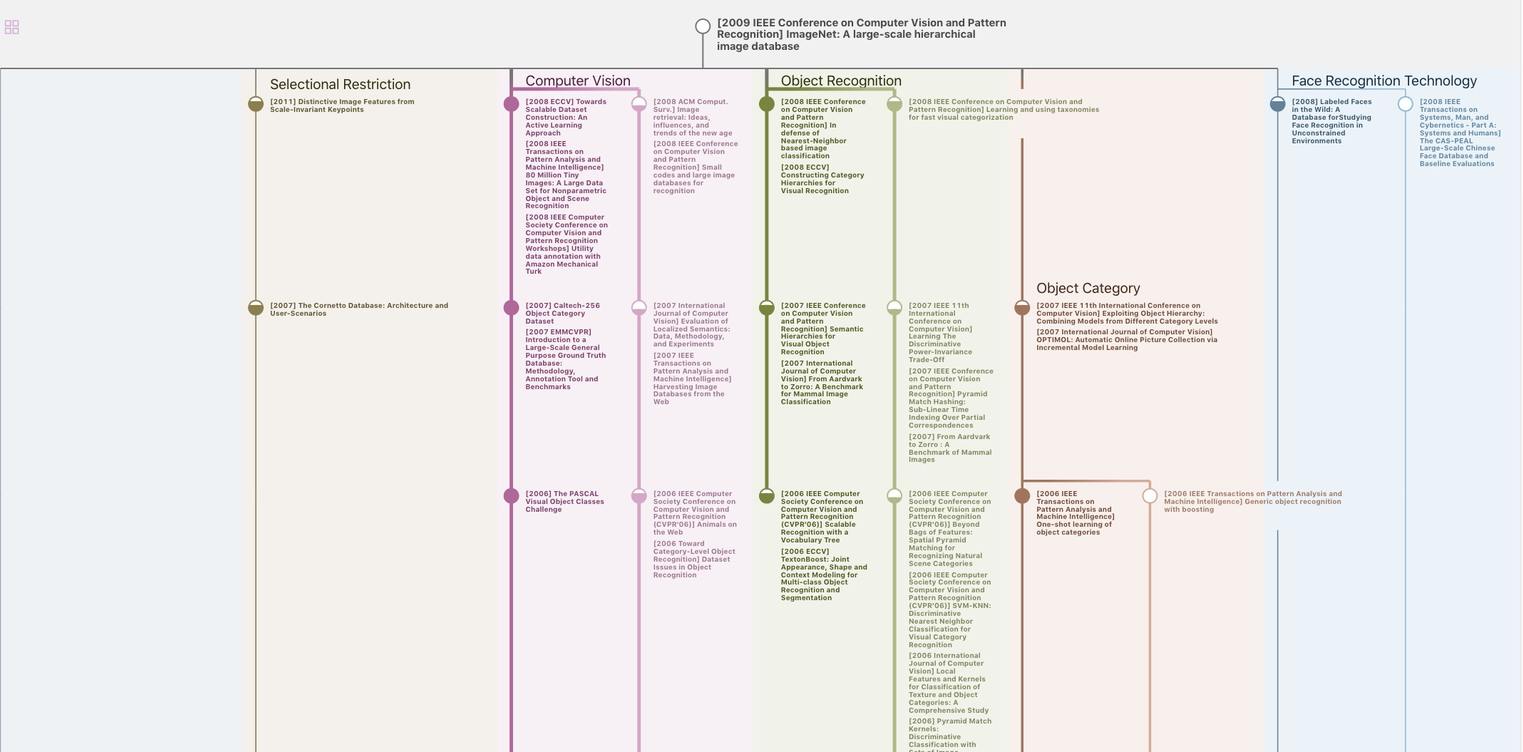
生成溯源树,研究论文发展脉络
Chat Paper
正在生成论文摘要