Enhancement of Building Energy Consumption Using a Digital Twin based Neural Network Model Predictive Control.
ICCAD(2023)
摘要
The development of an optimal control strategy to significantly reduce energy requirements while minimizing performance losses, including expected comfort during use, is of great necessity given today's environmental, energy and economic challenges. Advanced technological tools, data processing algorithms and digital twins must work together to overcome these energy issues. It turns out that 60% of the building sector's energy consumption comes from HVAC (Heating, Ventilation and Air Conditioning) systems, which requires changes in their control strategies. However, a prior knowledge of these needs is necessary for the optimization of these energy performances. Digital twins are a key element in the evolution of the industry of the future. The integration of such technology in the building sector could improve the energy performance, at local, regional and national level. MPC (Model Predictive Control) allows for the control of complex systems, as in the case of thermal comfort in buildings. Its use in this study allowed to reduce the energy demand for heating and cooling significantly, respectively from 4,631 kWh to 2,824 kWh (39.02%) and from 11,834 kWh to 9,025 kWh (27.34%). The model used in this controller is an artificial neural network, which was fed with data generated by a dynamic thermal simulator. The bi-directional data transfer between the real model and the digital twin, through the MQTT protocol, thanks to the IoT, and the storage of these data are precious, that will allow the continuous improvement of this neural network model and thus the energy performance of the building first and the building sector later.
更多查看译文
关键词
MPC,ANN,IoT,MQTT,Building energy efficiency
AI 理解论文
溯源树
样例
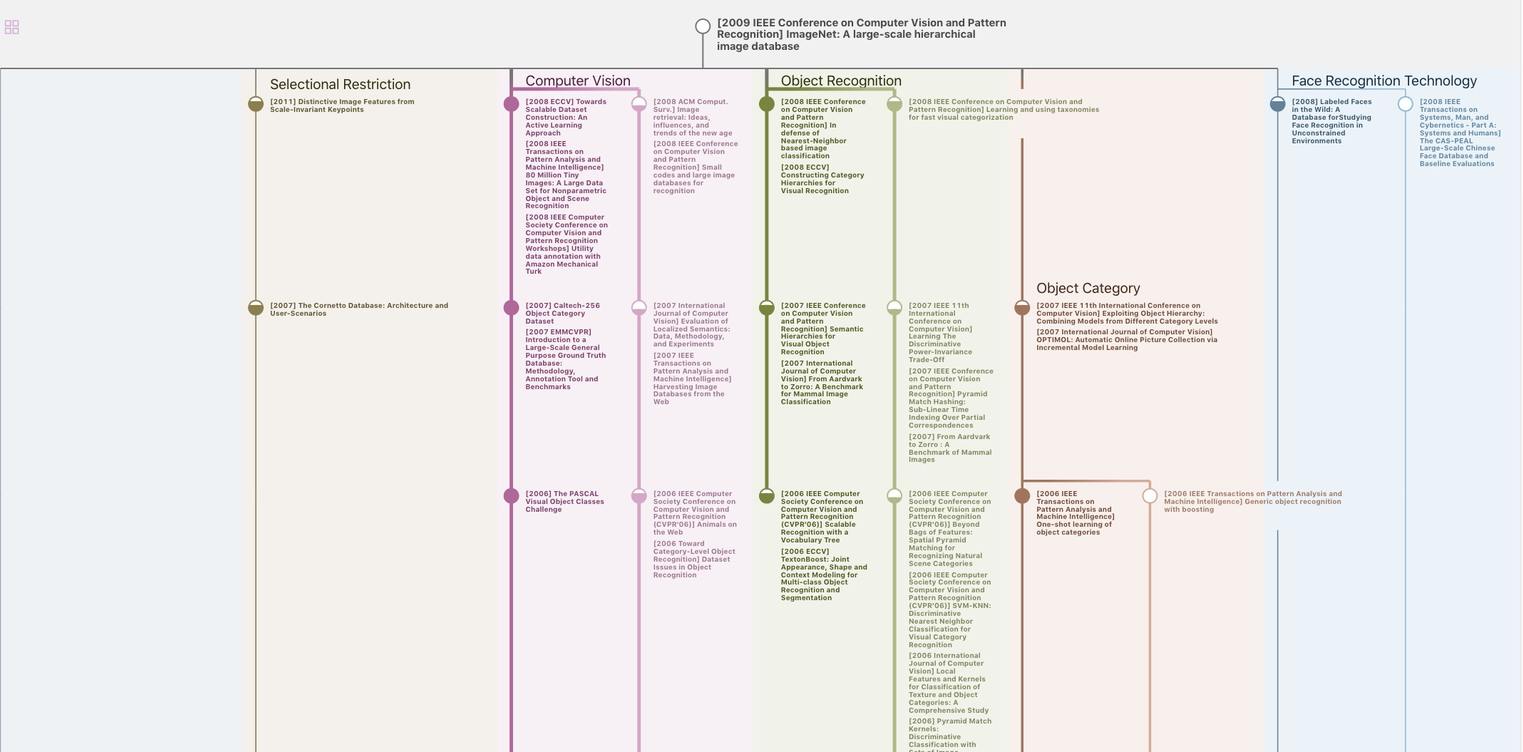
生成溯源树,研究论文发展脉络
Chat Paper
正在生成论文摘要