COMPACT: Co-processor for Multi-mode Precision-adjustable Non-linear Activation Functions
2023 DESIGN, AUTOMATION & TEST IN EUROPE CONFERENCE & EXHIBITION, DATE(2023)
摘要
Non-linear activation functions imitating neuron behaviors are ubiquitous in machine learning algorithms for time series signals while also demonstrating significant gain in precision for conventional vision-based deep learning networks. State-of-th-eart implementation of such functions on GPU-like devices incurs a large physical cost, whereas edge devices adopt either linear interpolation or simplified linear functions leading to degraded precision. In this work, we design COMPACT, a co-processor with adjustable precision for multiple non-linear activation functions including but not limited to exponent, sigmoid, tangent, logarithm, and mish. Benchmarking with state-of-the-arts, COMPACT achieves a 26% reduction in the absolute error on a 1.6x widen approximation range taking advantage of the triple decomposition technique inspired by Hajduk's formula of Pade approximation. A SIMD-ISA-based vector co-processor has been implemented on FPGA which leads to a 30% reduction in execution latency but the area overhead nearly remains the same with related designs. Furthermore, COMPACT is adjustable to 46% latency improvement when the maximum absolute error is tolerant to the order of 1E-3.
更多查看译文
关键词
Approximate computing,non-linear functions,architecture exploration
AI 理解论文
溯源树
样例
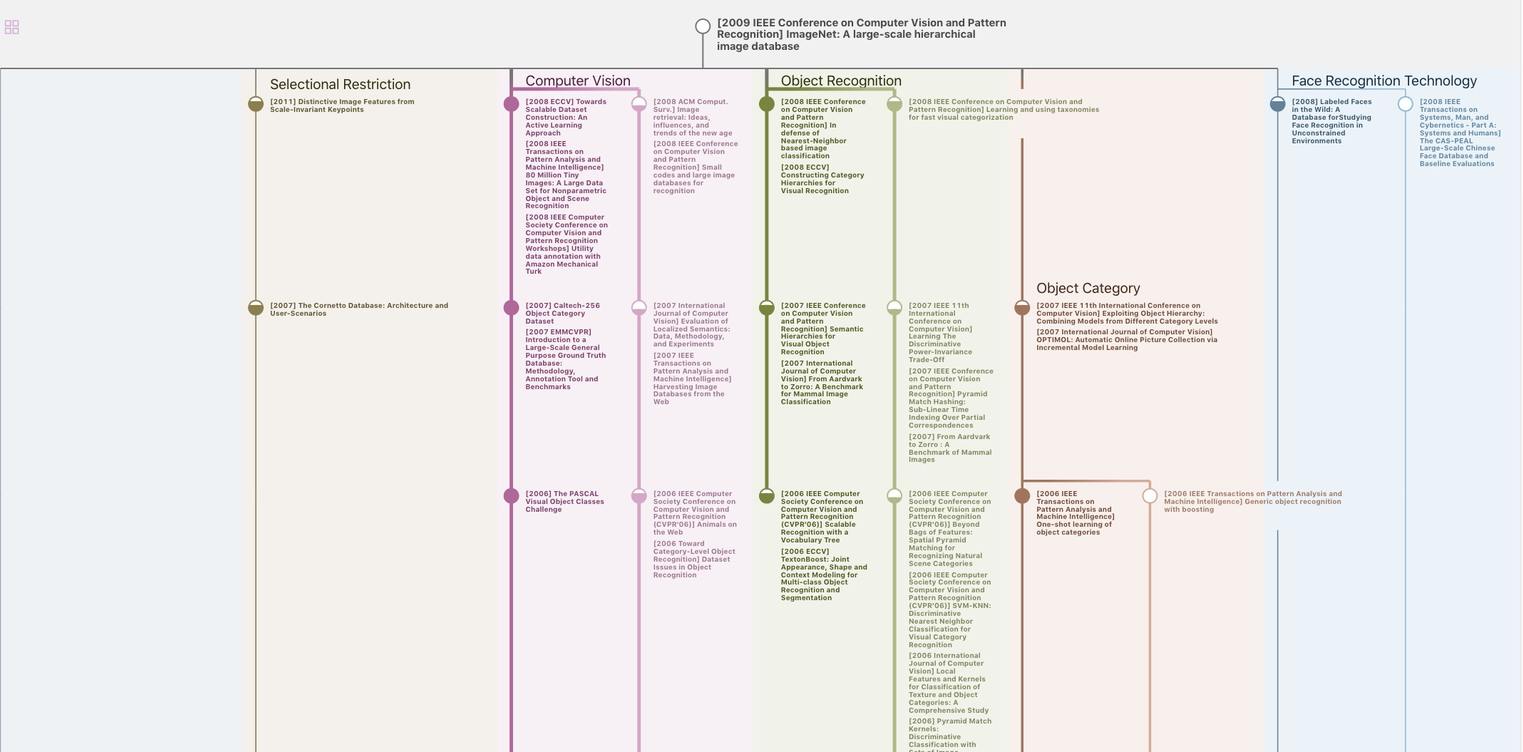
生成溯源树,研究论文发展脉络
Chat Paper
正在生成论文摘要