A Speed- and Energy-Driven Holistic Training Framework for Sparse CNN Accelerators
2023 DESIGN, AUTOMATION & TEST IN EUROPE CONFERENCE & EXHIBITION, DATE(2023)
摘要
Sparse convolution neural network (CNN) accelerators have shown to achieve high processing speed and low energy consumption by leveraging zero weights or activations, which can be further optimized by finely tuning the sparse activation maps in training process. In this paper, we propose a CNN training framework targeting at reducing energy consumption and processing cycles in sparse CNN accelerators. We first model accelerator's energy consumption and processing cycles as functions of layer-wise activation map sparsity. Then we leverage the model and propose a hybrid regularization approximation method to further sparsify activation maps in the training process. The results show that our proposed framework can reduce the energy consumption of Eyeriss by 31.33%, 20.6% and 26.6% respectively on MobileNet-V2, SqueezeNet and Inception-V3. In addition, the processing speed can be increased by 1.96x, 1.4x and 1.65x respectively.
更多查看译文
关键词
CNN Accelerator,Training,Activation Map Sparsification,Energy Consumption,Processing Speed
AI 理解论文
溯源树
样例
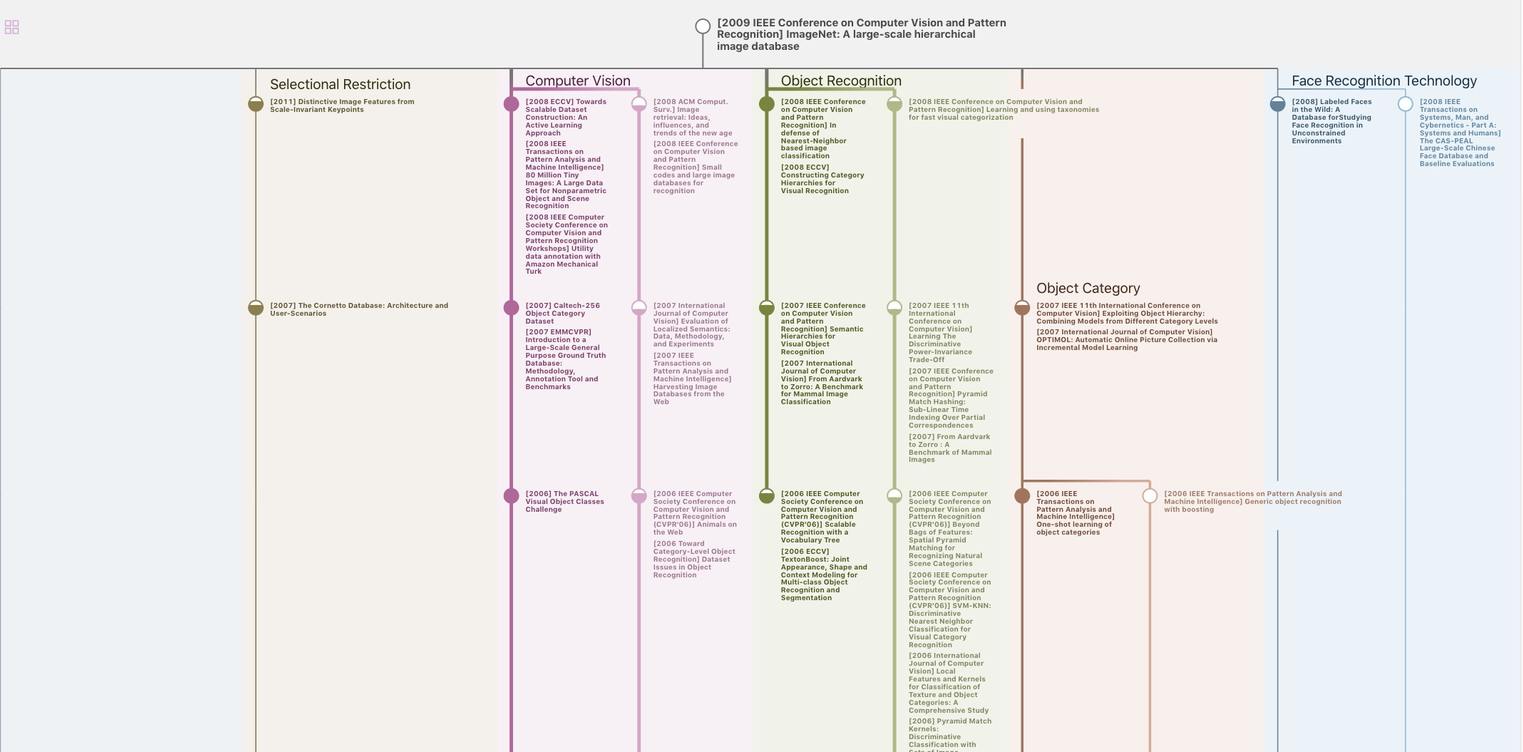
生成溯源树,研究论文发展脉络
Chat Paper
正在生成论文摘要