FSL-HD: Accelerating Few-Shot Learning on ReRAM using Hyperdimensional Computing
2023 DESIGN, AUTOMATION & TEST IN EUROPE CONFERENCE & EXHIBITION, DATE(2023)
摘要
Few-shot learning (FSL) is a promising meta-learning paradigm that trains classification models on the fly with a few training samples. However, existing FSL classifiers are either computationally expensive, or are not accurate enough. In this work, we propose an efficient in-memory FSL classifier, FSL-HD, based on hyperdimensional computing (HDC) that achieves state-of-the-art FSL accuracy and efficiency. We devise an HDC-based FSL framework with efficient HDC encoding and search to reduce high complexity caused by the large dimensionality. Also, we design a scalable in-memory architecture to accelerate FSL-HD on ReRAM with distributed dataflow and organization that maximizes the data parallelism and hardware utilization. The evaluation shows that FSL-HD achieves 4.2% higher accuracy compared to other FSL classifiers. FSL-HD achieves 100-1000x better energy efficiency and 9 - 66x speedup over the CPU and GPU baselines. Moreover, FSL-HD is more accurate, scalable and 2.5x faster than the state-of-the-art ReRAM-based FSL design, SAPIENS, while requiring 85% less area.
更多查看译文
关键词
In-memory processing,Few-shot learning,Hyperdimensional computing
AI 理解论文
溯源树
样例
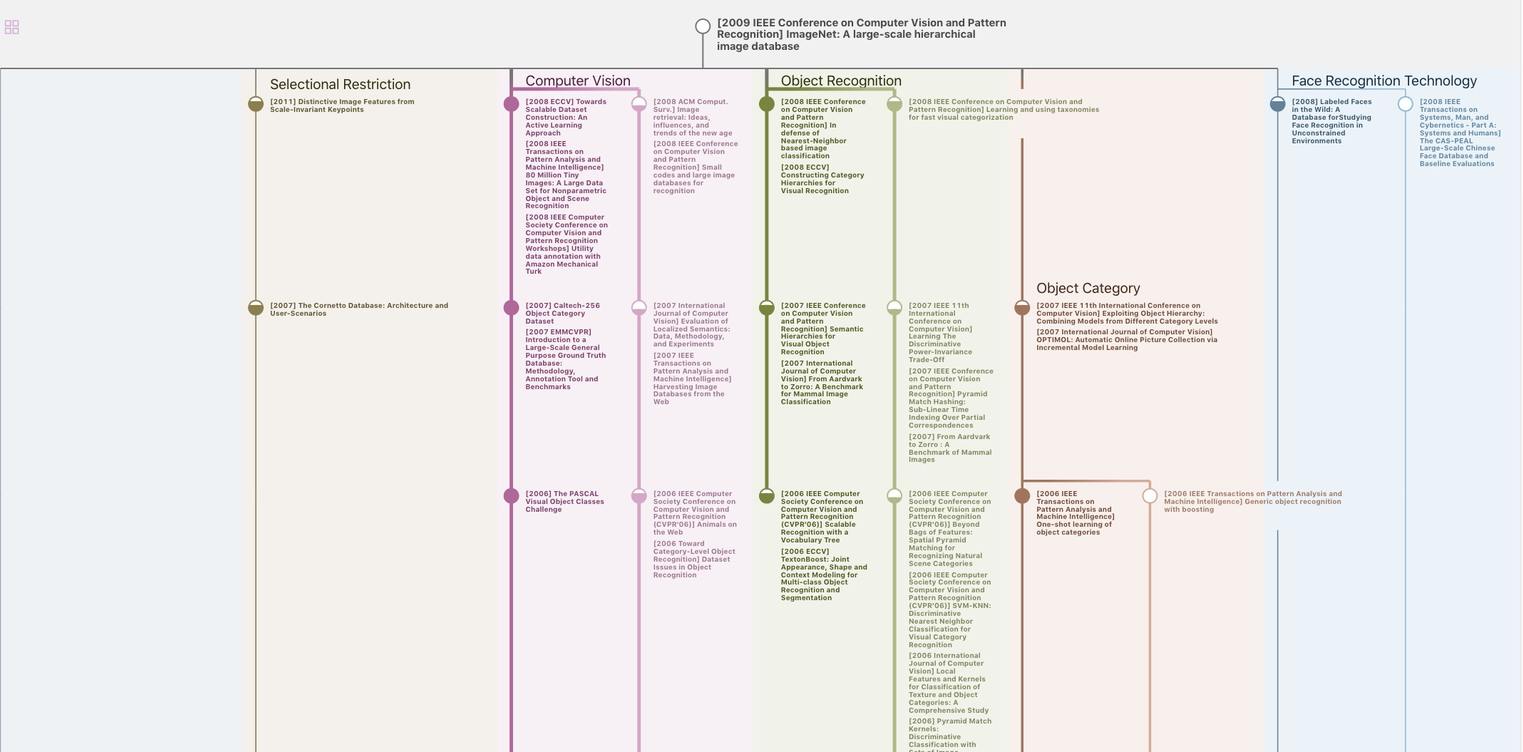
生成溯源树,研究论文发展脉络
Chat Paper
正在生成论文摘要