Learning General Representations for Semantic Segmentation and Height Estimation from Remote Sensing Images.
JURSE(2023)
摘要
Deep-learning methods have been applied to remote-sensing imagery to achieve height estimation and semantic segmentation. Recent research has demonstrated that multi-task learning methods can act as a welcome addition to task-specific features by improving prediction accuracy across a range of tasks. How to effectively learn representations that achieve good performance for multiple tasks still remains challenging. We propose to adopt a unified network jointly learning multiple vision tasks’ general representations by aligning them via small task-specific adapters. The experimental results on the Vaihingen dataset demonstrate that general representation learning can improve the performance of state-of-the-art methods in height estimation and semantic segmentation tasks.
更多查看译文
关键词
Multi-task learning,semantic segmentation,height estimation,remote sensing image,knowledge distillation
AI 理解论文
溯源树
样例
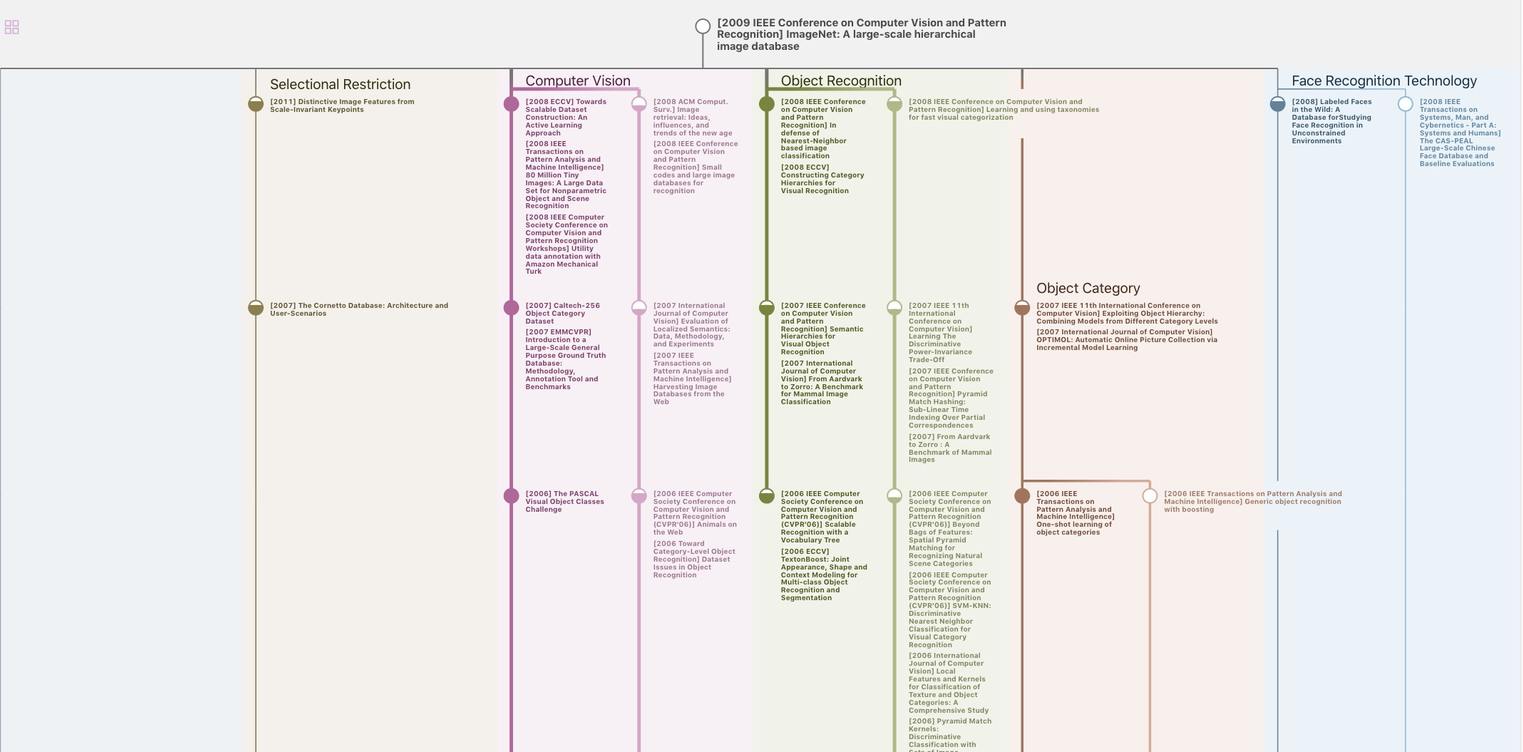
生成溯源树,研究论文发展脉络
Chat Paper
正在生成论文摘要