Direct Window-to-Wall Ratio Prediction Using Deep Learning Approaches.
JURSE(2023)
摘要
A building’s window-to-wall ratio (WWR) plays a critical role in estimating heat loss, solar gain and daylighting levels, and is therefore essential for building energy modeling applications. Typically, an accurate WWR estimation corresponds to an accurate window segmentation result, which requires high quality rectified and annotated façade images. In this paper, we propose a novel end-to-end regression model that directly predicts the invisible building attribute, the WWR, from façade imagery. For comparison, we have adopted the latest proposed semantic segmentation of windows from façade images and calculate the WWR based on the result of the semantic segmentation. These two approaches are performed and compared on three public façade benchmarks. The experimental results demonstrate that the direct prediction of invisible building attributes is feasible. Furthermore, the regression-based approach can achieve similar WWR accuracy as the segmentation-based method when they use the same backbone.
更多查看译文
关键词
Deep learning,semantic segmentation,regression,window-to-wall ratio (WWR),urban building energy modeling (UBEM)
AI 理解论文
溯源树
样例
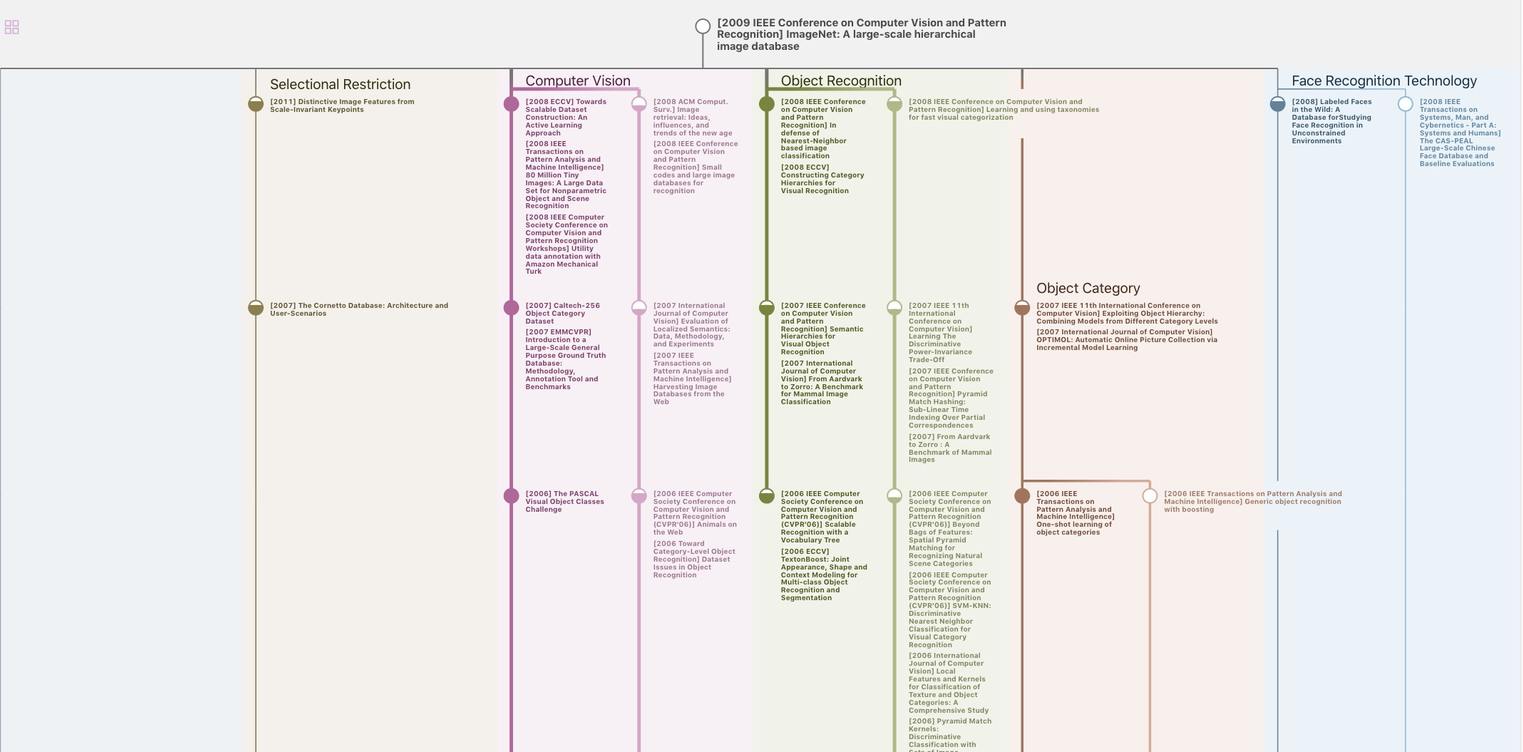
生成溯源树,研究论文发展脉络
Chat Paper
正在生成论文摘要