Efficiency of CNNs for Building Extraction: Comparative Analysis of Performance and Time.
JURSE(2023)
摘要
Openly available geodata of buildings are still incomplete or missing for many regions of the world. Convolutional neural networks (CNNs) have shown to be suitable for building extraction and thus, can help to overcome these shortcomings. In this study, we compare 16 encoder-decoder combinations for the task of building extraction from very high-resolution (VHR) aerial imagery in terms of performance, time needed for training and validation, and, efficiency. Therefore, we train and evaluate nine encoder models using a Feature Pyramid Network (FPN) decoder, and seven decoder models using a residual neural network (ResNet) encoder, more specifically ResNet50. The analysis is performed for two types of input data: RGB-NIR and RGB-NIR-nDSM. The results reveal that the majority of the investigated segmentation models show a high similarity in the area of performance, whereas the time needed for training and validation is exceptionally different. Both parameters, performance and time, are combined for an efficiency ranking, and the models are ranked accordingly. We found that a ResNet50 and FPN combination is the most suitable for our application. The presented results help to evaluate how each model combination should be rated in terms of efficiency for building extraction.
更多查看译文
关键词
building extraction,convolutional neural network,encoder,decoder,comparative analysis,efficiency,aerial imagery,semantic segmentation
AI 理解论文
溯源树
样例
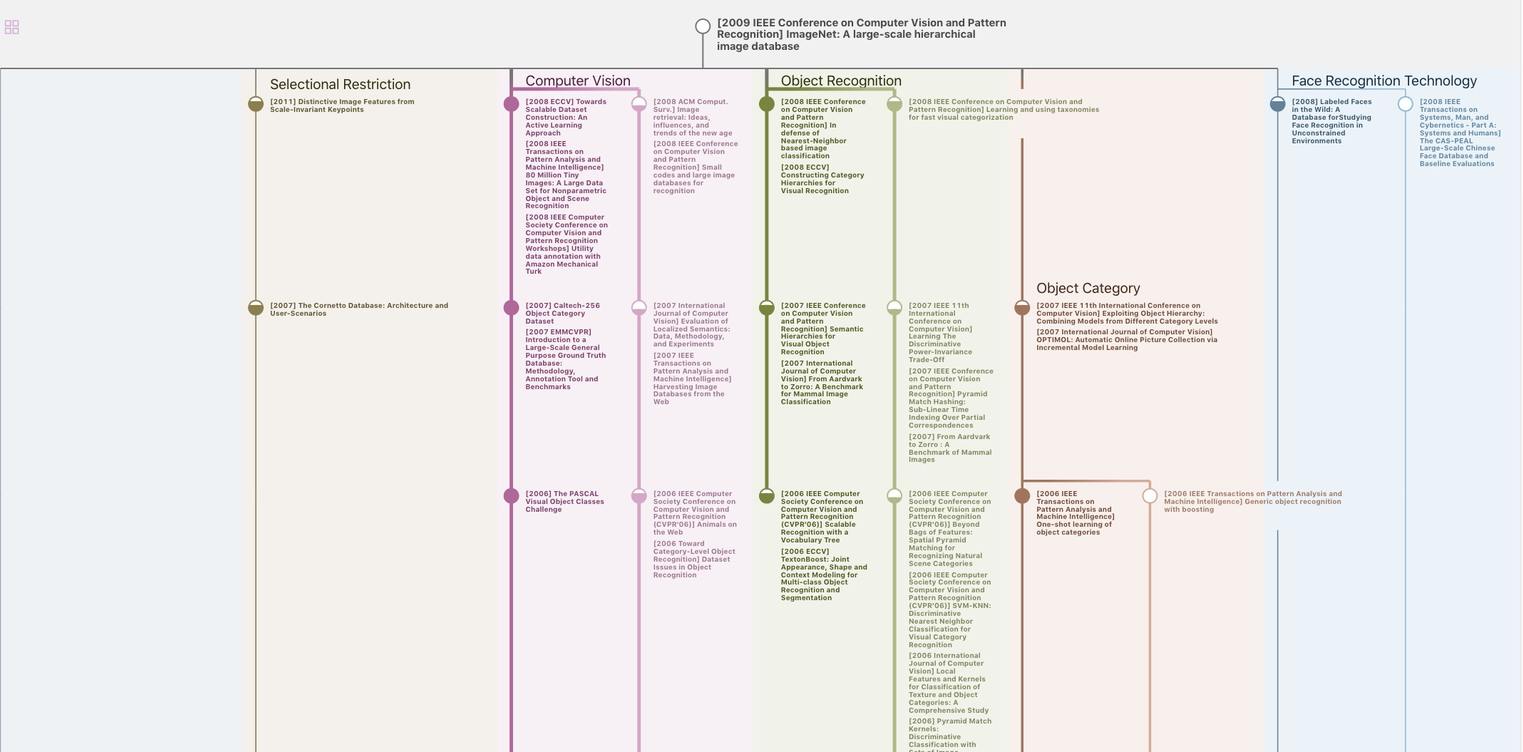
生成溯源树,研究论文发展脉络
Chat Paper
正在生成论文摘要