A Comparative Analysis of Automatic Speech Recognition Errors in Small Group Classroom Discourse
UMAP(2023)
摘要
In collaborative learning environments, effective intelligent learning systems need to accurately analyze and understand the collaborative discourse between learners (i.e., group modeling) to provide adaptive support. We investigate how automatic speech recognition (ASR) errors influence discourse models of small group collaboration in noisy real-world classrooms. Our dataset consisted of 30 students recorded by consumer off-the-shelf microphones (Yeti Blue) while engaging in dyadic- and triadic- collaborative learning in a multi-day STEM curriculum unit. We found that two state-of-the-art ASR systems (Google Speech and OpenAI Whisper) yielded very high word error rates (0.822, 0.847) but very different profiles of error with Google being more conservative, rejecting 38% of utterances instead of 12% for Whisper. Next, we examined how these ASR errors influenced down-stream small group modeling based on pre-trained large language models for three tasks: Abstract Meaning Representation parsing (AMRParsing), on-task/off-task detection (ONTASK), and Accountable Productive Talk prediction (TALKMOVE). As expected, models trained on clean human transcripts yielded degraded performance on all three tasks, measured by the transfer ratio (TR). However, the TR of the specific sentencelevel AMRParsing task (.39 -.62) was much lower than that of the abstract discourse-level ONTASK (.63-.94) and TALKMOVE tasks (.64.72). Furthermore, different training strategies that incorporated ASR transcripts alone or as augmentations of human transcripts increased accuracy for the discourse-level tasks (ONTASK and TALKMOVE) but not AMRParsing. Simulation experiments suggested that the models were tolerant of missing utterances in the dialog context, and that jointly improving ASR accuracy on important word classes (e.g., verbs and nouns) can improve performance across all tasks. Overall, our results provide insights into how different types of NLP-based tasks might be tolerant of ASR errors under extremely noisy conditions and provide suggestions for how to improve accuracy in small group modeling settings for a more equitable, engaging, and adaptive collaborative learning environment.
更多查看译文
关键词
Group Discourse Analysis,Automatic Speech Recognition,Text Tagging,Collaborative Learning
AI 理解论文
溯源树
样例
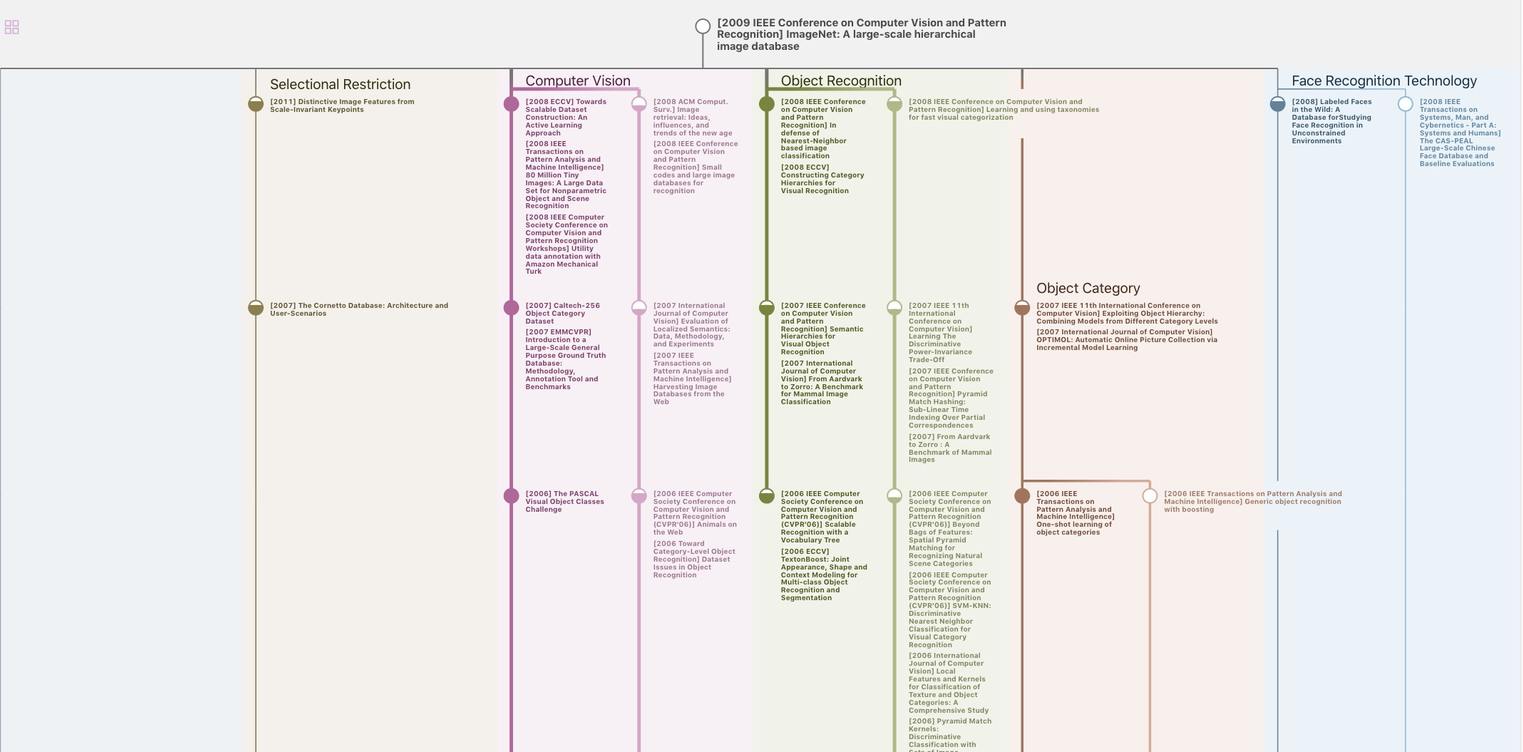
生成溯源树,研究论文发展脉络
Chat Paper
正在生成论文摘要