Federated Learning based Object Detection using Dampened Harmonic Optimization.
ICMLSC(2023)
摘要
Object Detection is the task of detecting and localizing objects of importance in visual media. The rapid increase in the number of powerful end devices such as mobiles and surveillance cameras have to lead to increasing in both resources and media generation. Object Detection has now become possible on end devices, but certain challenges need to be tackled to fully utilize the resources. Federated Learning is one such framework that leverages the end device resources to build machine learning models while preserving data privacy. We model the federated learning framework for object detection on real-world heterogeneous datasets using a novel dampened harmonic optimizer to enhance local learning on the end device and hence reducing the communication cost during the learning process. We provide comparison of the commonly used FedAvg with our FedHarm optimization on multiple object detection models and datasets to demonstrate the merits of our proposition. Our method FedHarm with its dampened updates allows for greater local computation which reduces the overall communication rounds between edge devices and cloud and better handles heterogeneity in real-world datasets. FedHarm leads to faster convergence by 41% on average over FedAvg which is supported by extensive experiments.
更多查看译文
AI 理解论文
溯源树
样例
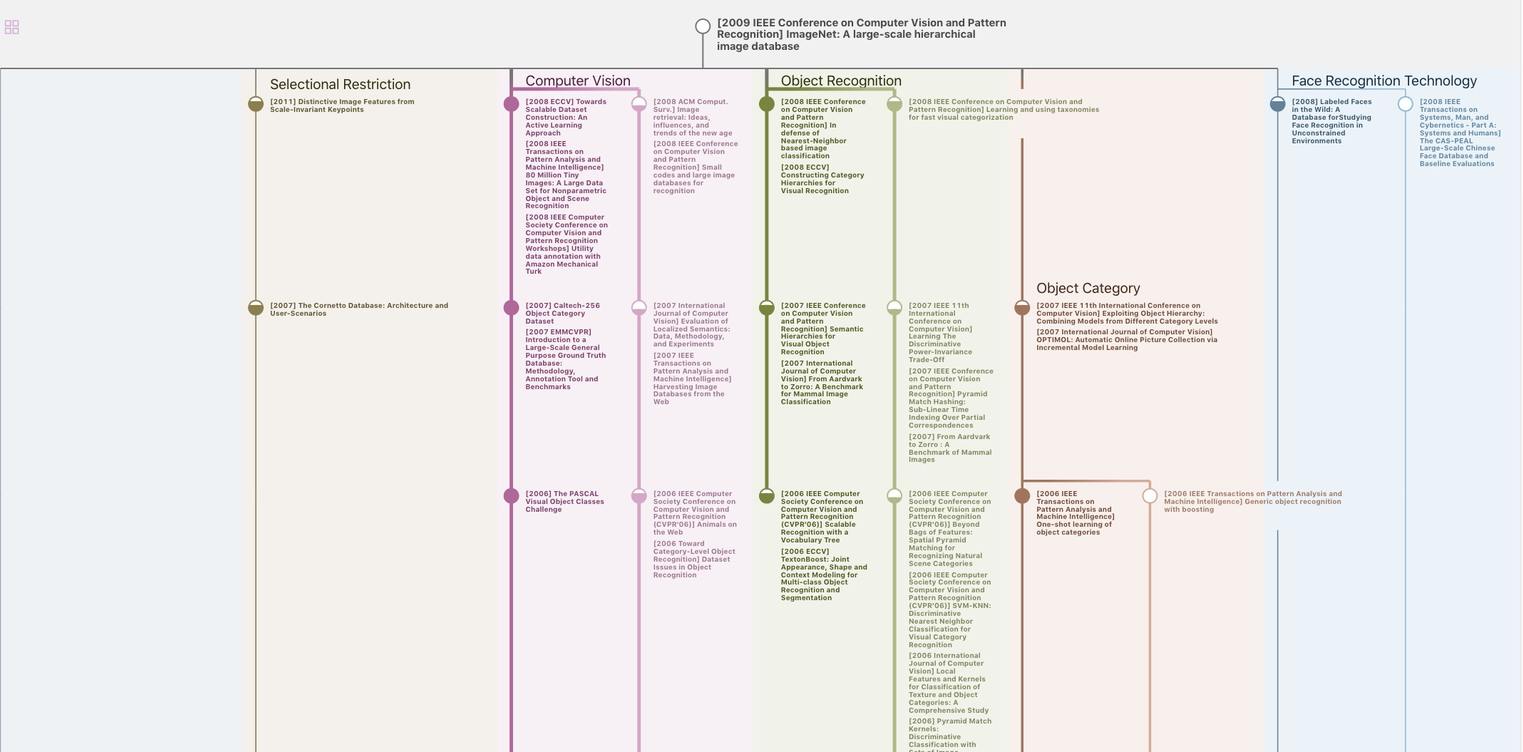
生成溯源树,研究论文发展脉络
Chat Paper
正在生成论文摘要