Early Detection of Intrusion in SDN.
NOMS(2023)
摘要
An intrusion detection system (IDS) is an essential component of any modern network. The purpose of an IDS is to detect intrusion and generate appropriate alarms so that the intrusion can be mitigated. Implementing an IDS in a Software Defined Network (SDN) is easier since an SDN controller has a centralized view of the whole network. Researchers have made many efforts to use machine learning (ML) for developing network-based IDS in SDN. The network-based IDS analyzes different characteristics of incoming network traffic to detect intrusion. Early detection of intrusion is crucial for an IDS because if the intrusion is not detected quickly enough, it can cause severe damage, such as data breaches and service shutdowns. This paper focuses on detecting intrusion in SDN as early as possible using real-time flow-based features. Our aim is to detect intrusion with less amount of packets per flow, which not only facilitates early intrusion detection but also is useful when an intrusion flow has less number of packets. We show that although ML models perform well in offline training on a dataset, their performance decreases ~25% when fewer packets are used to generate features for the ML model. In all our experiments, a simple Random Forest (RF) algorithm outperforms a complex deep learning model on a publicly available dataset for intrusion detection in SDN.
更多查看译文
关键词
Real-time intrusion detection,SDN,machine learning,flow-based features
AI 理解论文
溯源树
样例
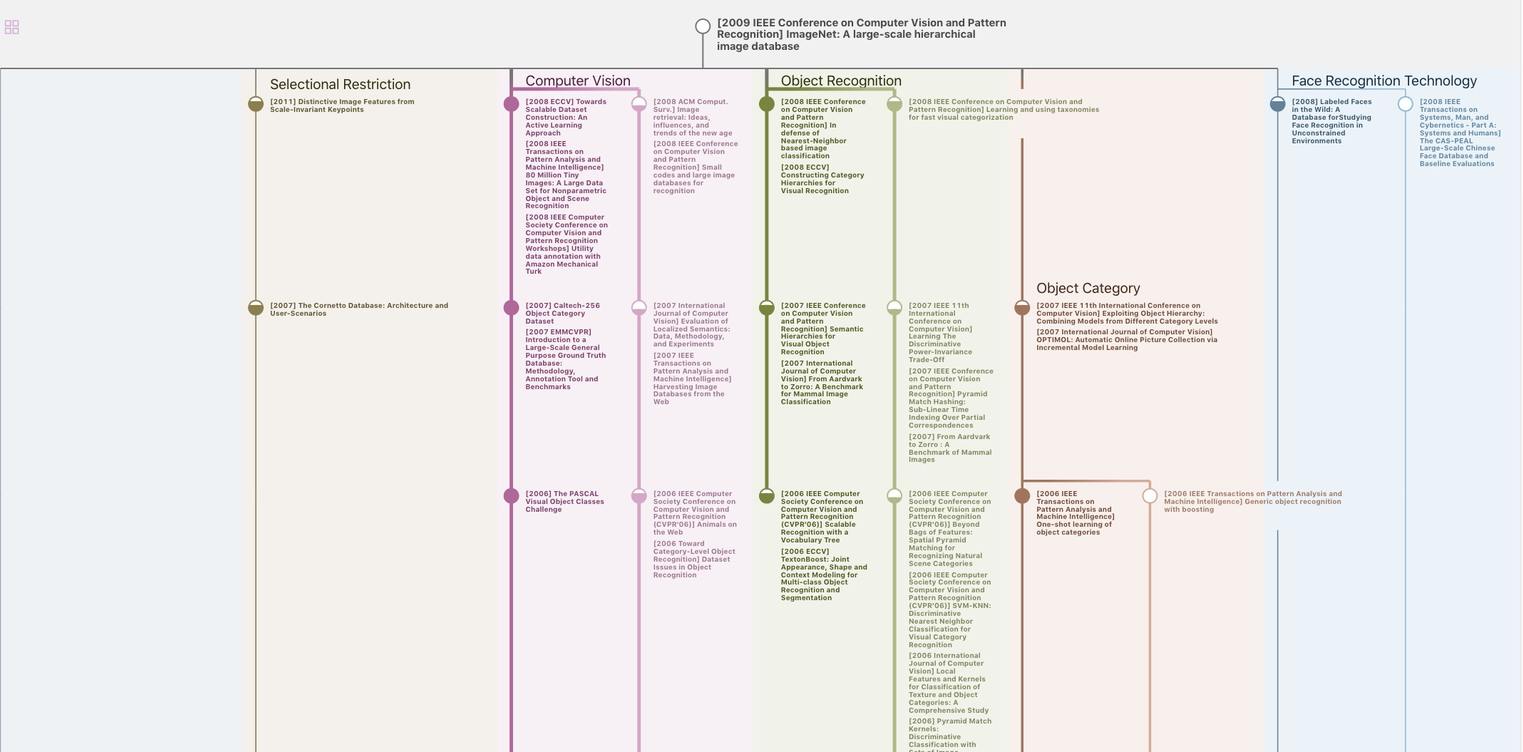
生成溯源树,研究论文发展脉络
Chat Paper
正在生成论文摘要