Generalizable GNN-based 5G RAN/MEC Slicing and Admission Control in Metropolitan Networks.
NOMS(2023)
摘要
The 5G RAN functions can be virtualized and distributed across the radio unit (RU), distributed unit (DU), and centralized unit (CU) to facilitate flexible resource management. Complemented by multi-access edge computing (MEC), these components create network slices tailored for applications with diverse quality of service (QoS) requirements. However, as the requests for various slices arrive dynamically over time and the network resources are limited, it is non-trivial for an infrastructure provider (InP) to optimize its long-term revenue from real-time admission and embedding of slice requests. Prior works have leveraged Deep Reinforcement Learning (DRL) to address this problem, however, these solutions either require re-training when facing topology changes or do not consider the slice admission and embedding problems jointly. In this paper, we use multi-agent DRL and Graph Attention Networks (GATs) to address these limitations. Specifically, we propose novel topology-independent admission and slicing agents that are scalable and generalizable to large and different metropolitan networks. Results show that the proposed approach converges faster and achieves up to 35.2% and 20% gain in revenue compared to heuristics and other DRL-based approaches, respectively. Additionally, we demonstrate that our approach is generalizable to scenarios and substrate networks previously unseen during training, as it maintains superior performance without re-training or re-tuning.
更多查看译文
关键词
5G RAN,MEC,network slicing,Deep Reinforcement Learning,Graph Attention Networks
AI 理解论文
溯源树
样例
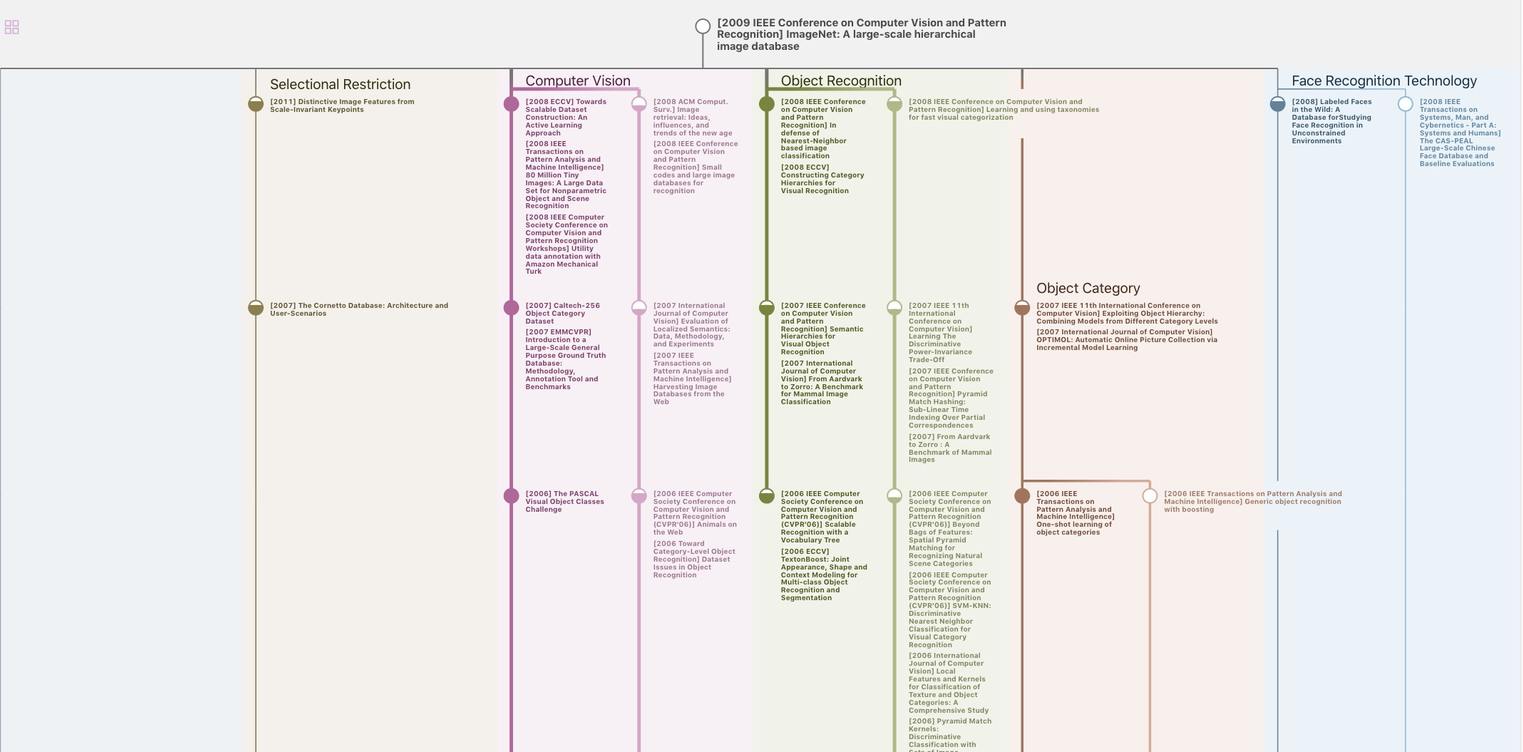
生成溯源树,研究论文发展脉络
Chat Paper
正在生成论文摘要