SFL-LEO: Secure Federated Learning Computation Based on LEO Satellites for 6G Non-Terrestrial Networks.
NOMS(2023)
摘要
We propose using federated learning (FL) in loiv Earth orbit (LEO) satellite networks for the Internet of Remote Things (IoRTs) to enable adaptive learning in massively networked devices while reducing costly traffic in satellite communication (SatCom). In this resource-constrained space setting, FL techniques in LEO satellite-based learning can improve system energy efficiency and save time. However, FL raises security and risk concerns, as local model updates can be used to infer device information by a hostile federated aggregator server in space. To address this, we propose using homomorphic-based encryption and decryption security techniques for federated aggregators and IoRTs. We evaluate the secure learning performance of our proposed framework using simulations on advanced datasets and aggregation approach. The results shoiv that compared to the benchmark scheme, the proposed secured computing networks improve communication overhead and latency performance.
更多查看译文
关键词
6G,federated learning,security,privacy
AI 理解论文
溯源树
样例
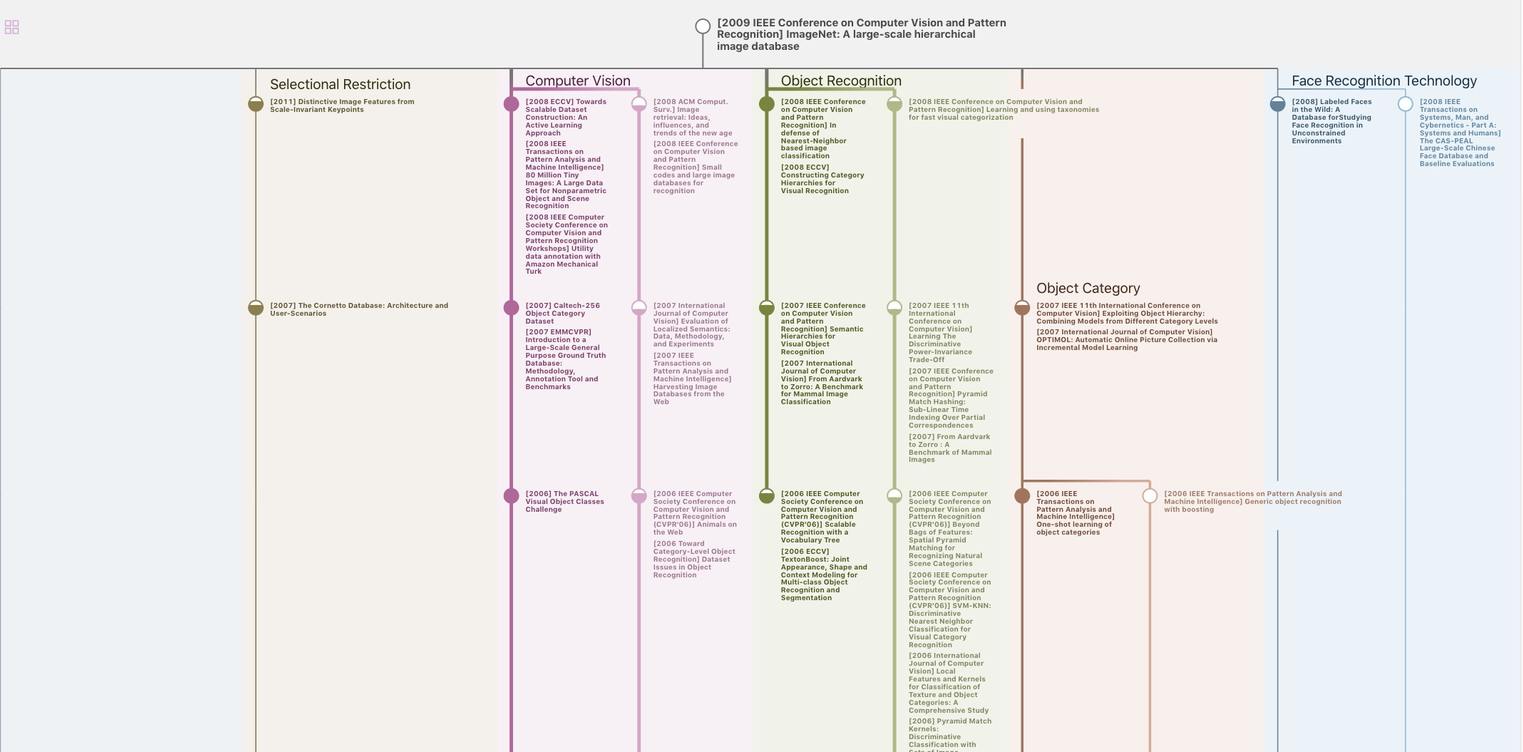
生成溯源树,研究论文发展脉络
Chat Paper
正在生成论文摘要