Deep Learning-Based Multi-tasking System for Diabetic Retinopathy in UW-OCTA Images.
MIDOG/DRAC@MICCAI(2022)
摘要
Diabetic retinopathy causes various abnormality in retinal vessels. In addition, Detection and identification of vessel anomaly are challenging due to nature of complexity in retinal vessels. UW-OCTA provides high-resolution image of those vessels to diagnose lesions of vessels. However, the image suffers noise of image. We here propose a deep learning-based multi-tasking systems for DR in UW-OCTA images to deal with diagnosis and checking image quality. We segment three kinds of retinal lesions with data-adaptive U-Net architectures, i.e. nnUNet, grading images on image quality and DR severity grading by soft-voting outputs of fine-tuned multiple convolutional neural networks. For three tasks, we achieve Dice similarity coefficient of 0.5292, quadratic weighted Kappa of 0.7246, and 0.7157 for segmentation, image quality assessment, and grading DR for test set of DRAC2022 challenge. The performance of our proposed approach demonstrates that task-adaptive U-Net planning and soft ensemble of CNNs can provide enhancement of the performance of single baseline models for diagnosis and screening of UW-OCTA images.
更多查看译文
关键词
diabetic retinopathy,learning-based,multi-tasking,uw-octa
AI 理解论文
溯源树
样例
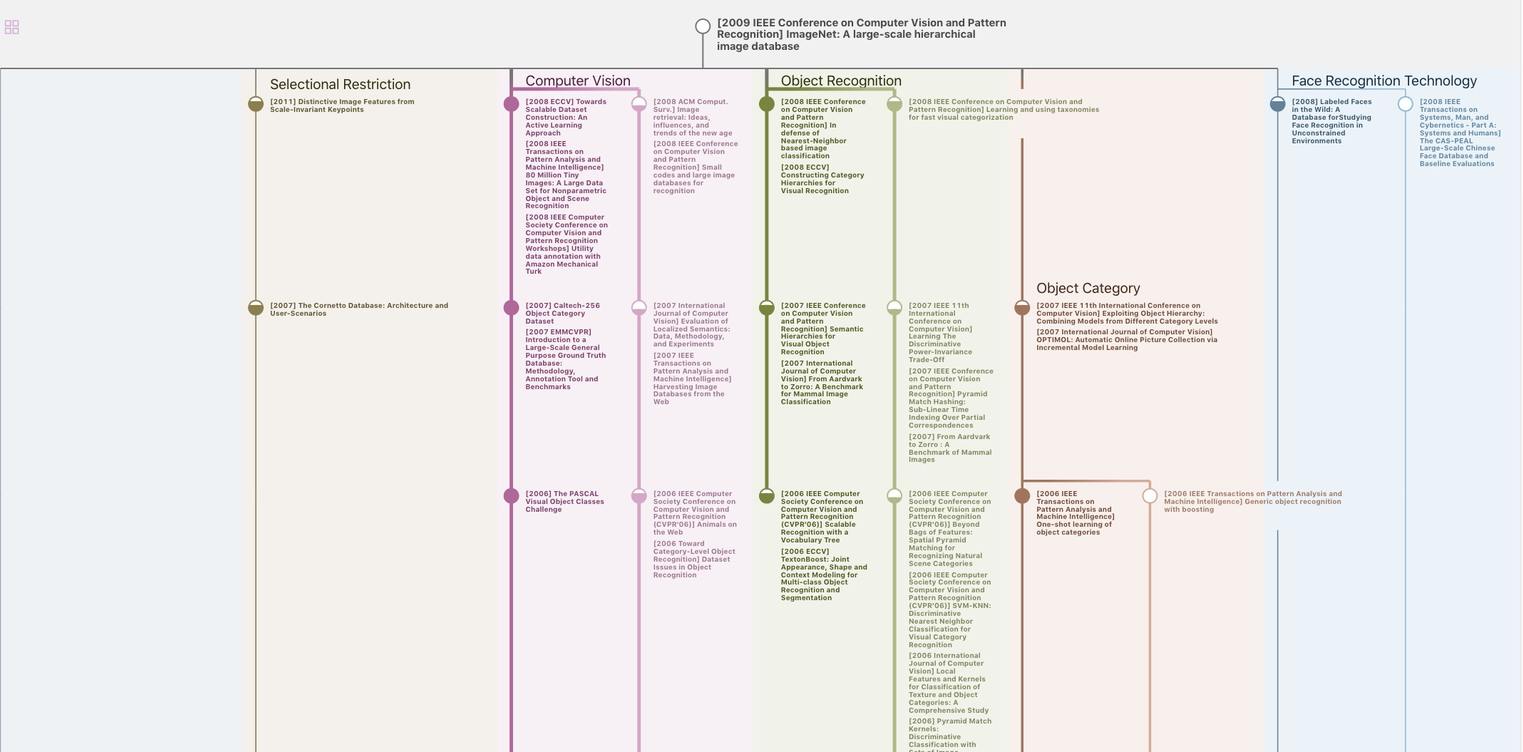
生成溯源树,研究论文发展脉络
Chat Paper
正在生成论文摘要