Basic Taste Sensation Recognition From EEG Based on Multiscale Convolutional Neural Network With Residual Learning
IEEE Trans. Instrum. Meas.(2023)
摘要
Taste sensation recognition is a keystone for taste-related brain-computer interface (BCI). A commonly used measurement of brain activity in response to specific stimulation is through electroencephalography (EEG) signals. However, it remains challenging to develop accurate and generalizable EEG-based measurement for human taste sensations. This article proposes EEG-MSRNet, a novel fully convolutional neural network (CNN) for EEG-based classification of basic taste sensations (blank, sour, sweet, bitter, salty, and umami). First, a multiscale temporal convolution operation with residual learning is designed to extract features in different frequencies from the downsampled EEG signals. Subsequently, a multiscale spatial convolution operation represents the features in a cross-channel manner. Finally, a convolutional layer and global average pooling (GAP) layer are introduced to make predictions with the feature representation instead of the commonly used fully connected layers for classification. An experimental procedure is developed to acquire the EEG signals under taste stimulation. Comparison experiments and ablation studies have proved the stable and generalizable recognition performance of EEG-MSRNet on our self-collected EEG dataset. The results suggest that our EEG-based system with EEG-MSRNet is effective and generalizable for taste sensation recognition, which provides a powerful measurement for taste-related BCI such as taste disorder diagnosis and virtual taste.
更多查看译文
关键词
Electroencephalography,Convolution,Feature extraction,Electrodes,Convolutional neural networks,Sugar,Recording,Basic taste sensation recognition,brain-computer interface (BCI),convolutional neural network (CNN),electroencephalography (EEG)
AI 理解论文
溯源树
样例
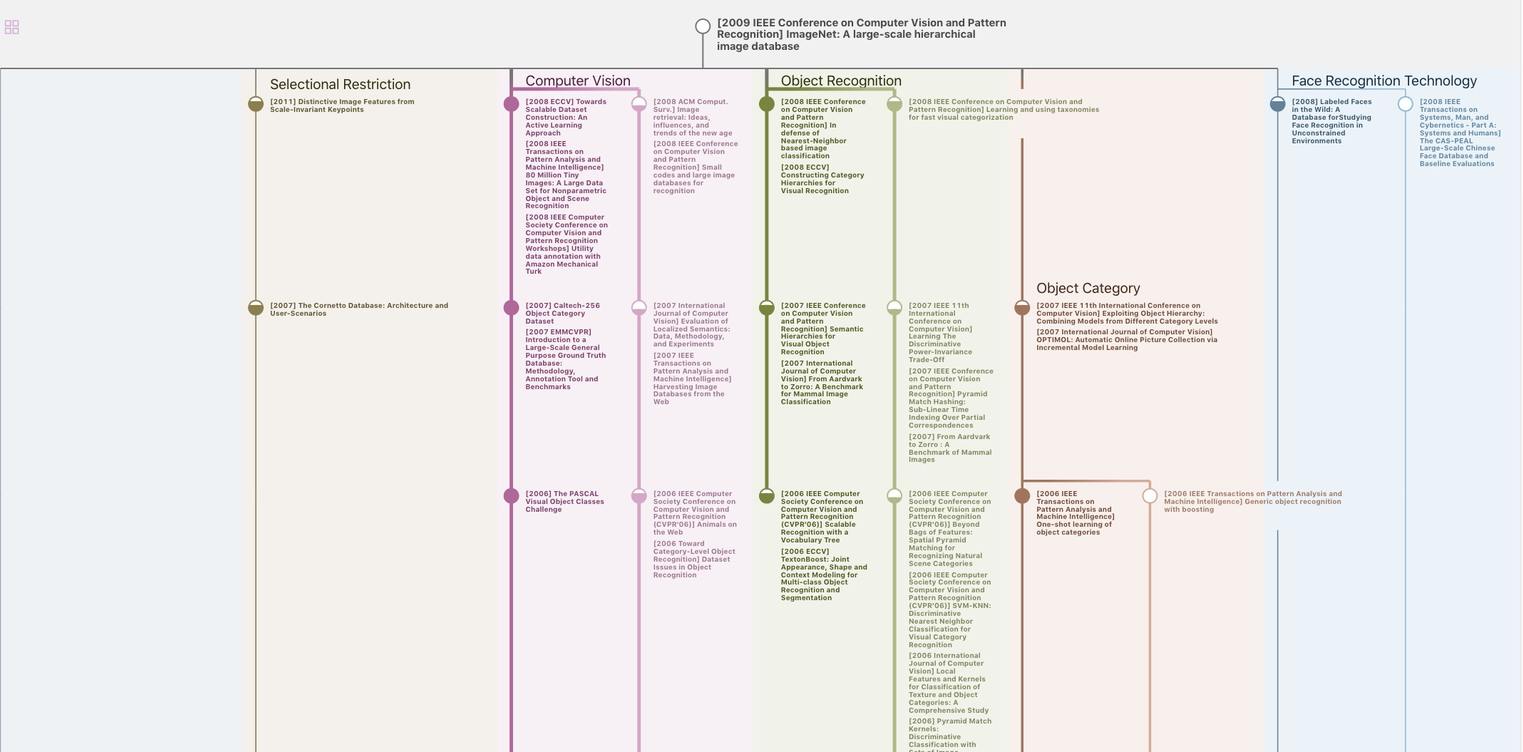
生成溯源树,研究论文发展脉络
Chat Paper
正在生成论文摘要